An Adversarial Attack Detection Paradigm With Swarm Optimization
2020 INTERNATIONAL JOINT CONFERENCE ON NEURAL NETWORKS (IJCNN)(2020)
摘要
The rise of smart devices and applications has increased the dependence of human beings on machine learning (ML) based code-driven systems. While many of the pragmatic problems such as image classification, medical diagnosis, and statistical arbitrage have been addressed by extensive recent research in machine learning, it still lacks substantial work in the field of adversarial attacks on safety-critical networked systems. It is a matter of significant importance, as using the adversarial samples, attackers are now able to evade pre-trained systems and mount black-box attacks hence increasing the false positives. In this research, we are proposing a Random Neural Network based Adversarial intrusion detection system (RNN-ADV). For adversarial attack generation, the Jacobian Saliency Map Attack (JSMA) algorithm has been used. Swarm optimization capabilities have been implemented by training the system with the Artificial Bee Colony (ABC) algorithm. Different scenarios have been designed and the proposed system is then evaluated with benchmark benign NSL-KDD dataset, adversarial data, and the performance is compared with deep neural networks (DNN) using several performance metrics. The results suggest that the proposed scheme outperforms DNN in terms of adversarial attack detection where it has successfully classified benign samples from crafted samples with better accuracy and high F1 scores.
更多查看译文
关键词
Intrusion Detection, Swarm Intelligence, Adversarial Machine Learning, NSL-KDD, JSMA
AI 理解论文
溯源树
样例
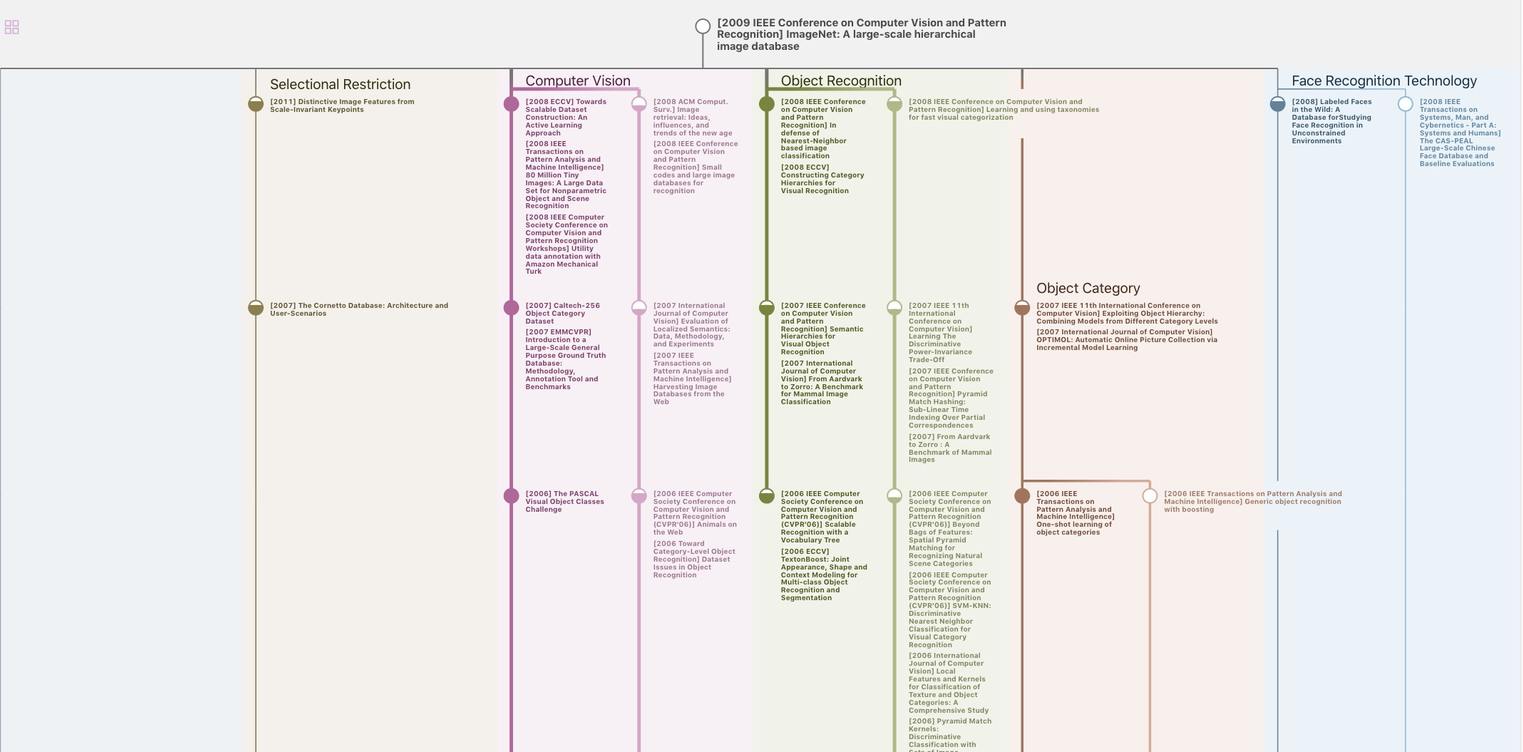
生成溯源树,研究论文发展脉络
Chat Paper
正在生成论文摘要