Subspace-Based Dynamic Selection: A Proof Of Concept Using Protein Microarray Data
2020 INTERNATIONAL JOINT CONFERENCE ON NEURAL NETWORKS (IJCNN)(2020)
摘要
Traditional dynamic selection methods fail to perform effectively when data dimensionality increases. In addition, those methods do not provide any insights into important features in the data, as the regions of competence used for data classification are always constituted by the same set of features. In this paper, we propose a two-stage framework based on subspace clustering using a Gaussian Based Estimator, followed by a k-Nearest subspace search mechanism to overcome these limitations of dynamic selection. The idea of subspace allows for regions of competence with different numbers of instances and dimension sizes. Our hypothesis is that by using our framework, we will achieve comparable results to the state-of-the-art dynamic selection, with the benefit of producing a model that helps to understand the importance of sets of features for the patterns found within the data. We test our approach to a high dimensional microarray data of insect bite hypersensitivity in horses. Results show that our approach is comparable to traditional dynamic selection methods in terms of accuracy. In addition, it facilitates the interpretability of the feature importance for each class of the dataset.
更多查看译文
关键词
dynamic selection, subspace clustering, Gaussian kernel density estimator, nearest subspace search, protein microarrays, insect bite hypersensitivity
AI 理解论文
溯源树
样例
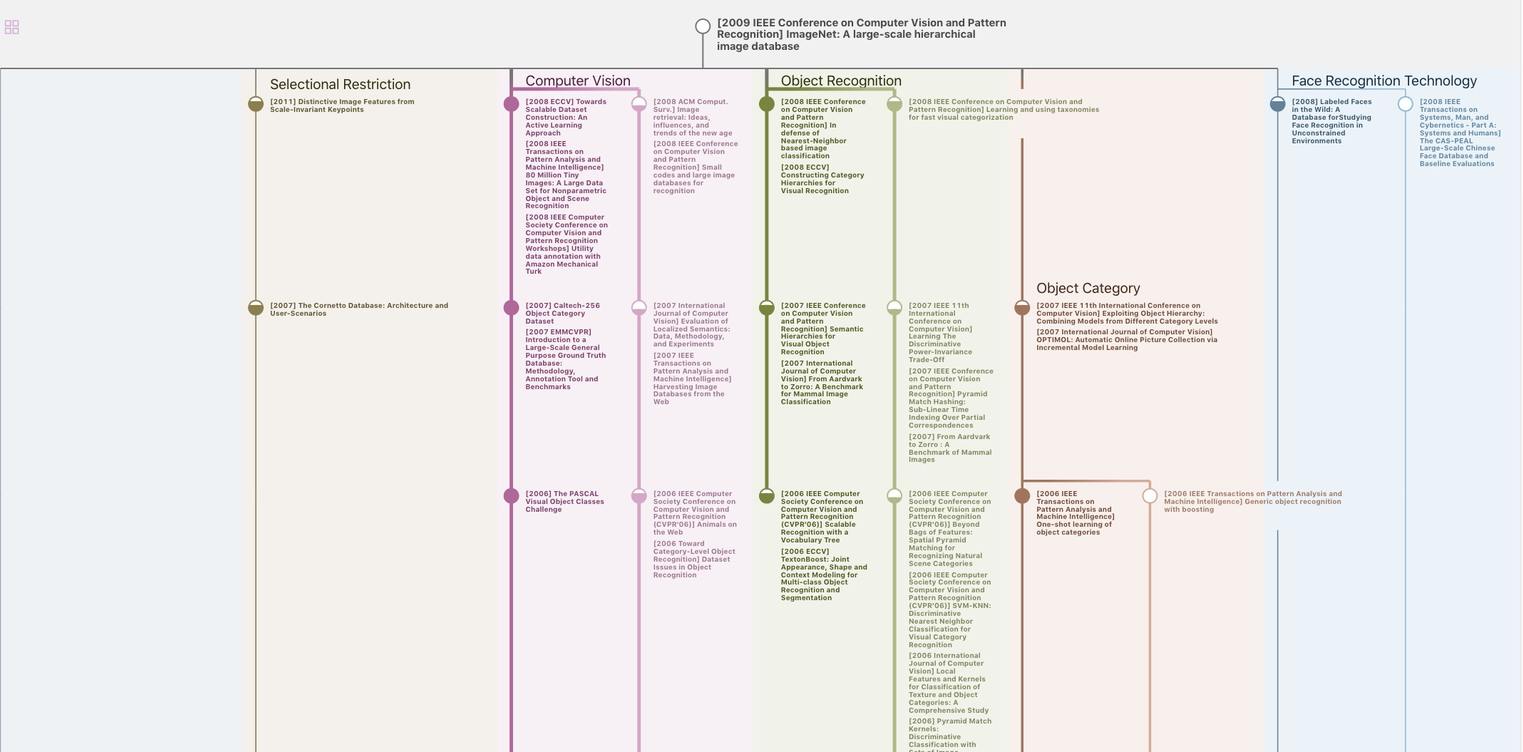
生成溯源树,研究论文发展脉络
Chat Paper
正在生成论文摘要