A Comparative Analysis Of Multi-Backbone Mask R-Cnn For Surgical Tools Detection
2020 INTERNATIONAL JOINT CONFERENCE ON NEURAL NETWORKS (IJCNN)(2020)
摘要
Real-time surgical tool segmentation and tracking based on convolutional neural networks (CNN) has gained increasing interest in the field of mini-invasive surgery. In fact, the application of this novel artificial vision technologies allows both to reduce surgical risks and to increase patient safety. Moreover, these types of models can be used both to track the tools and detect markers or external artefacts in a real-time video stream. Multiple object detection and instance segmentation can be addressed efficiently by leveraging region-based CNN models. Thus, this work provides a comparison among state-of-the-art multi-backbone Mask R-CNNs to solve these tasks. Moreover, we show that such models can serve as a basis for tracking algorithms. The models were trained and tested with a data-set of 4955 manually annotated images, validated by 3 experts in the field. We tested 12 different combinations of CNN backbones and training hyperparameters. The results show that it is possible to employ a modern CNN to tackle the surgical tool detection problem, with the best-performing Mask R-CNN configuration achieving 87% Average Precision (AP) at Intersection over Union (IOU) 0.5.
更多查看译文
关键词
comparative analysis,multibackbone Mask R-CNN,surgical tools detection,convolutional neural networks,mini-invasive surgery,artificial vision,surgical risks,patient safety,external artefacts,real-time video stream,instance segmentation,region-based CNN models,tracking algorithms,CNN backbones,surgical tool detection problem,mask R-CNN configuration,multibackbone mask R-CNNs
AI 理解论文
溯源树
样例
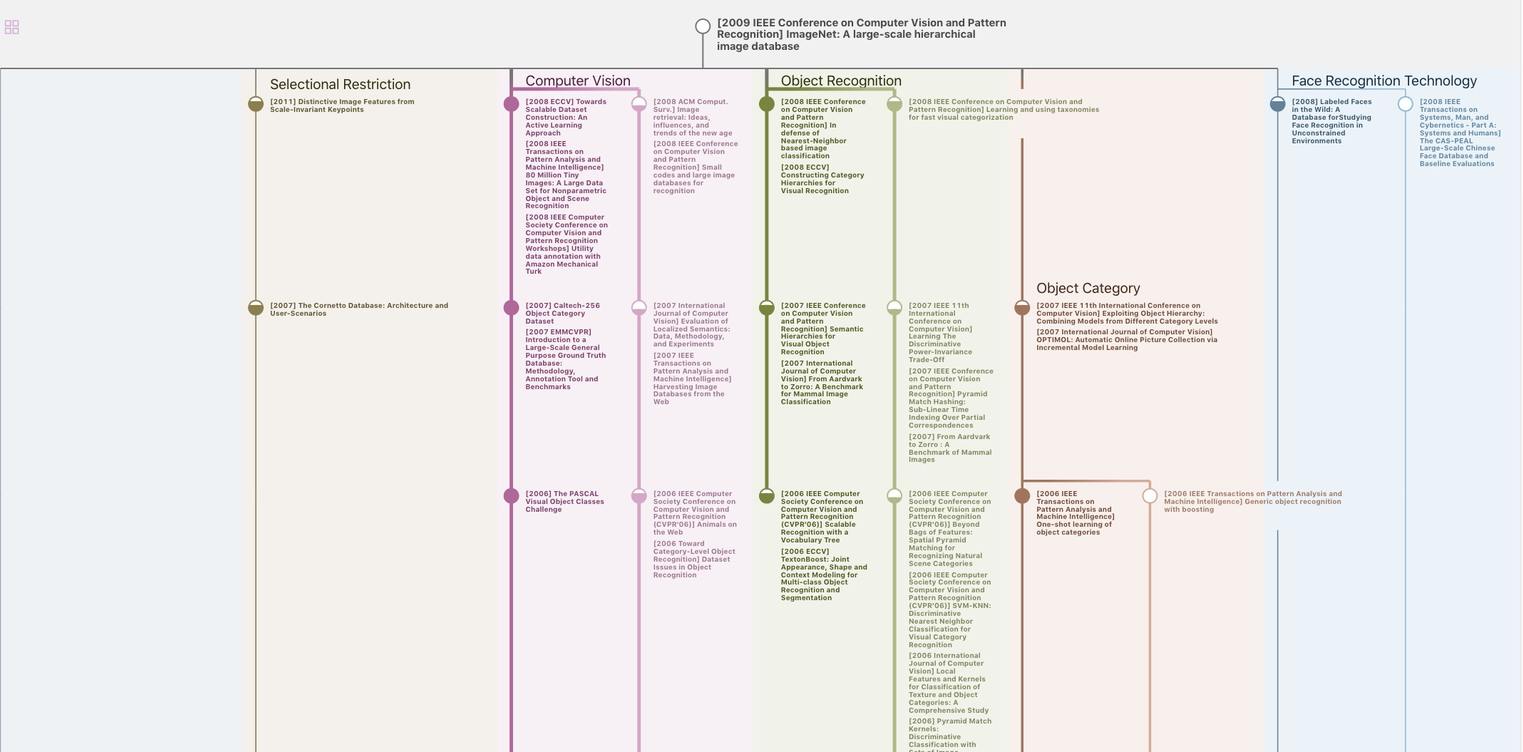
生成溯源树,研究论文发展脉络
Chat Paper
正在生成论文摘要