Predicting protein secondary structure by an ensemble through feature-based accuracy estimation
BCB(2020)
摘要
ABSTRACTProtein secondary structure prediction is a fundamental task in computational biology, basic to many bioinformatics workflows, with a diverse collection of tools currently available. An approach from machine learning with the potential to capitalize on such a collection is ensemble prediction, which runs multiple predictors and combines their predictions into one, output by the ensemble. We conduct a thorough study of seven different approaches to ensemble secondary structure prediction, several of which are novel, and show we can indeed obtain an ensemble method that significantly exceeds the accuracy of individual state-of-the-art tools. The best approaches build on a recent technique known as feature-based accuracy estimation, which estimates the unknown true accuracy of a prediction, here using features of both the prediction output and the internal state of the prediction method. In particular, a hybrid approach to ensemble prediction that leverages accuracy estimation is now the most accurate method currently available: on average over standard CASP and PDB benchmarks, it exceeds the state-of-the-art Q3 accuracy for 3-state prediction by nearly 4%, and exceeds the Q8 accuracy for 8-state prediction by more than 8%. A preliminary implementation of our approach to ensemble protein secondary structure prediction, in a new tool we call Ssylla, is available free for non-commercial use at ssylla.cs.arizona.edu.
更多查看译文
关键词
Protein secondary structure prediction, ensemble methods, feature-based accuracy estimation, method hybridization
AI 理解论文
溯源树
样例
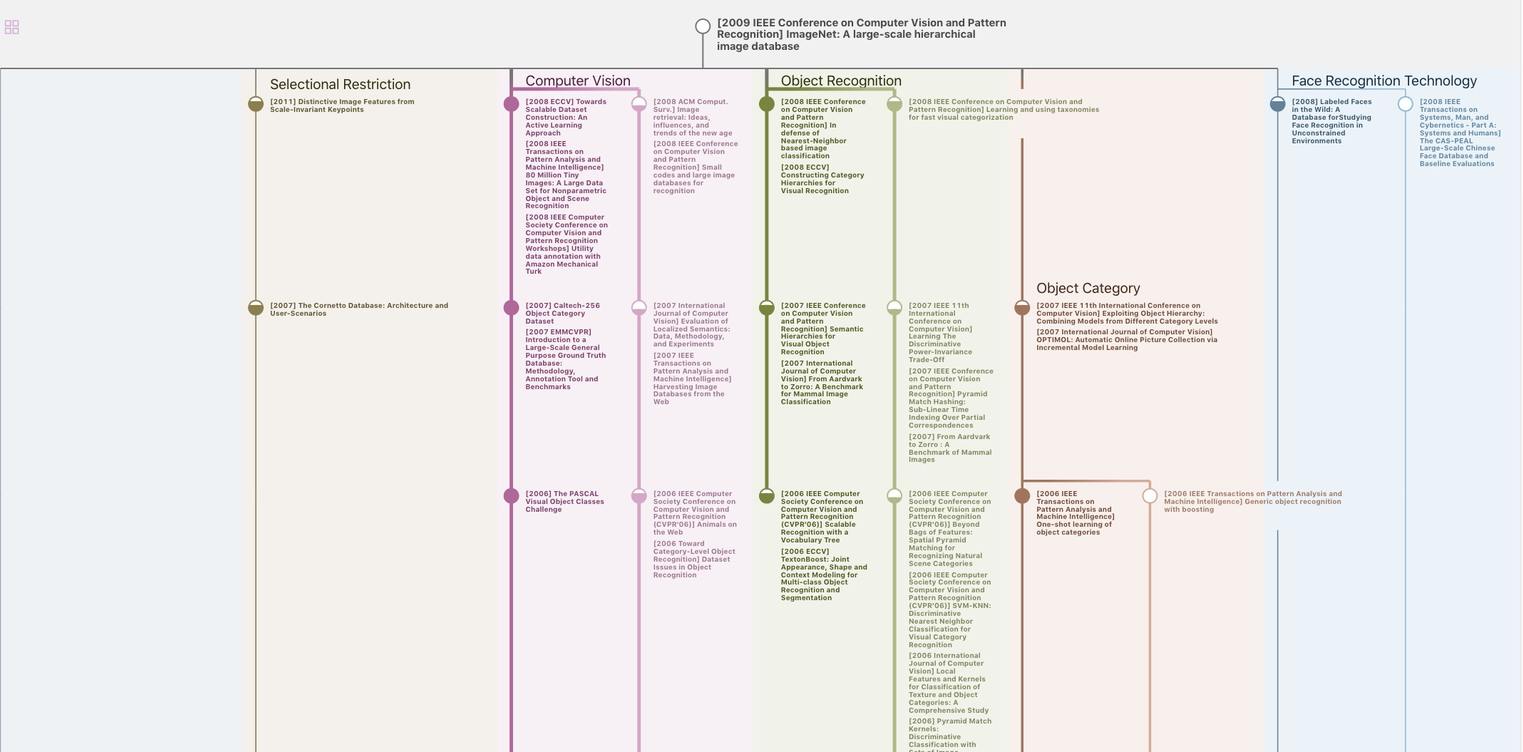
生成溯源树,研究论文发展脉络
Chat Paper
正在生成论文摘要