DQN-Based Deep Reinforcement Learning for Autonomous Driving.
WAF(2020)
摘要
The goal of this work is to evaluate the task of autonomous driving in urban environment using Deep Q-Network Agents. For this purpose, several approaches based on DQN agents will be studied. The DQN agent learn a policy (set of actions) for lane following tasks using visual and driving features obtained from sensors onboard the vehicle and a model-based path planner. The policy objective is to drive as fast as possible following the center of the lane avoiding collisions and road departures. A dynamic urban simulation environment will be designed using CARLA simulator to validate our proposal. The results show that a DQN agent could be a promising technique for self-driving a vehicle in a urban environment.
更多查看译文
关键词
deep reinforcement learning,reinforcement learning,autonomous driving,dqn-based
AI 理解论文
溯源树
样例
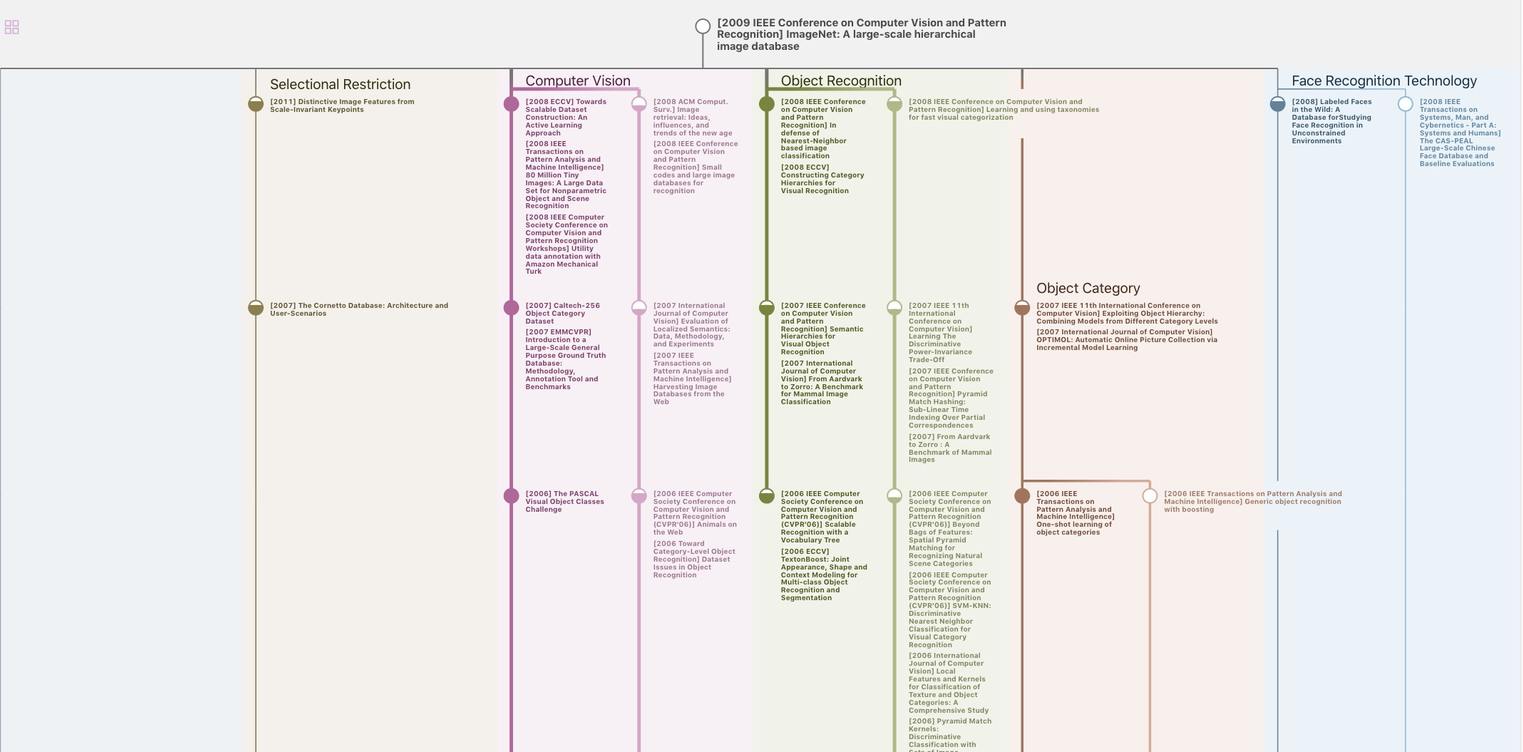
生成溯源树,研究论文发展脉络
Chat Paper
正在生成论文摘要