Aim-Net: Bring Implicit Euler To Network Design
2020 IEEE INTERNATIONAL CONFERENCE ON IMAGE PROCESSING (ICIP)(2020)
摘要
Researching networks' theoretical properties and behavior have drawn considerable attention from the perspective of ordinary differential equation (ODE). For solving ODE, explicit and implicit Euler schemes are the most common methods. Some works utilize explicit Euler theory to analyze and design networks. However, focusing on parameters convergence and system stability, implicit Euler has been proved to be better than explicit one. It motivates us to explore implicit Euler's potential in neural networks. In this paper, we establish connections between implicit Euler and networks, which also effectively explain some existing networks such as LISTA and DRRN. In addition, by re-deriving implicit Euler, we propose an adaptive implicit network (AIM-NET) which allows model to have a flexible convergence interval to ensure parameters convergence as well as model performance. Particularly, we obtain AIM-LISTA and AIM-DRRN by applying AIM-NET on LISTA and DRRN respectively. Finally, we perform experiments on both synthetic data and real images and the experimental results show that adaptive implicit structure is able to significantly improve performance.
更多查看译文
关键词
Ordinary different equation, implicit Euler, networks design, sparse signal recovery, image super-resolution
AI 理解论文
溯源树
样例
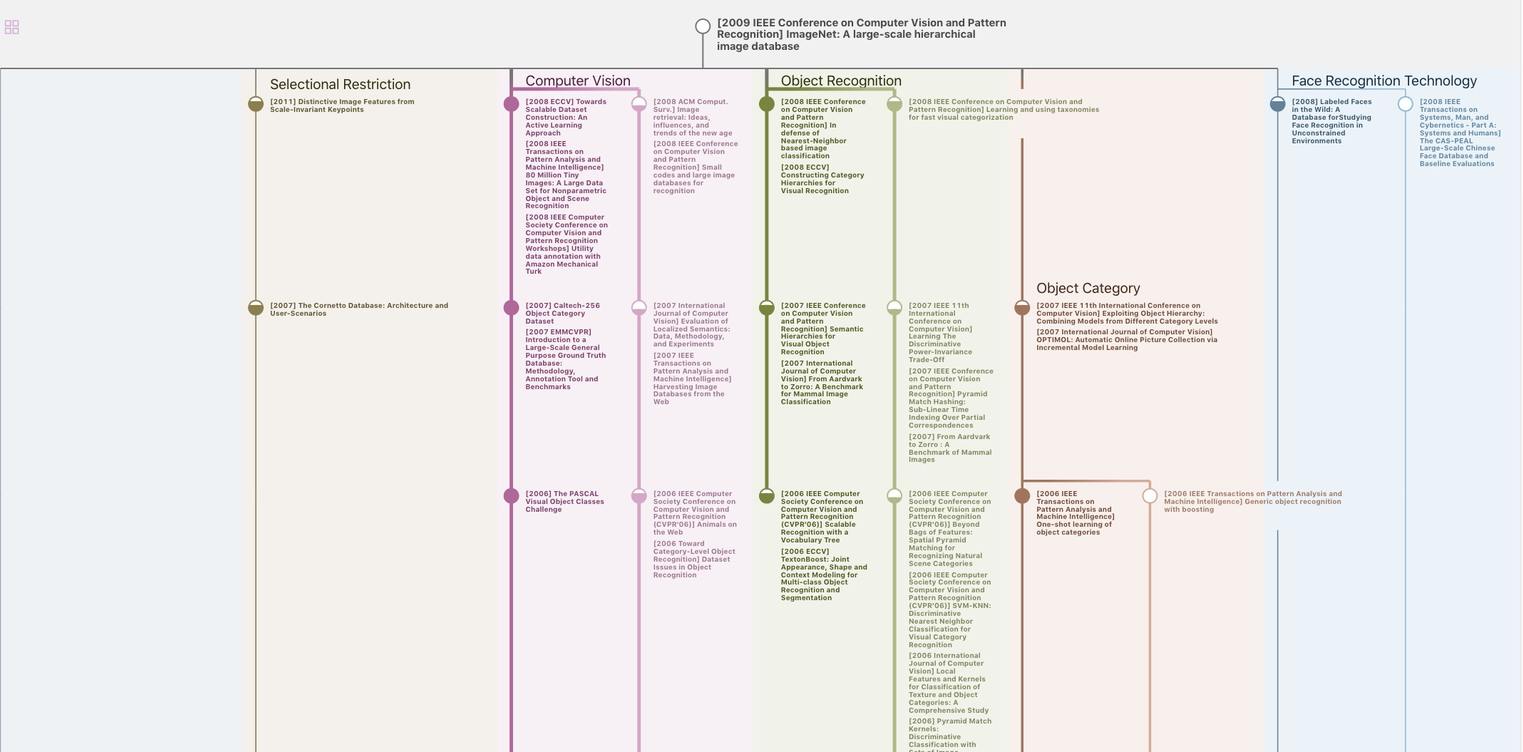
生成溯源树,研究论文发展脉络
Chat Paper
正在生成论文摘要