Discriminative Clip Mining for Video Anomaly Detection
2020 IEEE INTERNATIONAL CONFERENCE ON IMAGE PROCESSING (ICIP)(2020)
摘要
Real-world anomalous events are complicated, diverse, and rarely occurred. The main challenge to anomaly detection is to learn normal and anomalous patterns accurately. In this work, we propose discriminative clip mining for anomaly detection and classification: firstly, by introducing clip-level class activation mapping, an efficient Discriminative Anomalous Clip Miner (DACM) is developed to mine discriminative anomalous clips from a large number of normal ones; secondly, with the mined discriminative clips, an attentive ranking loss is designed to increase the anomalous instances hit rate of the traditional Multiple Instance Learning (MIL) model. Furthermore, by integrating the DACM and attentive MIL, one novel anomaly detection framework is proposed to learn more contrastive anomalous and normal patterns, and thus higher recognition performance can be achieved. Our experimental results on the widely-used UCF-Crime dataset show that, as compared to the state-of-the-art approaches, the proposed method achieves competitive performance both in anomaly detection and anomalous activity classification.
更多查看译文
关键词
anomaly detection, discriminative clip mining, multiple instance learning, contrastive pattern
AI 理解论文
溯源树
样例
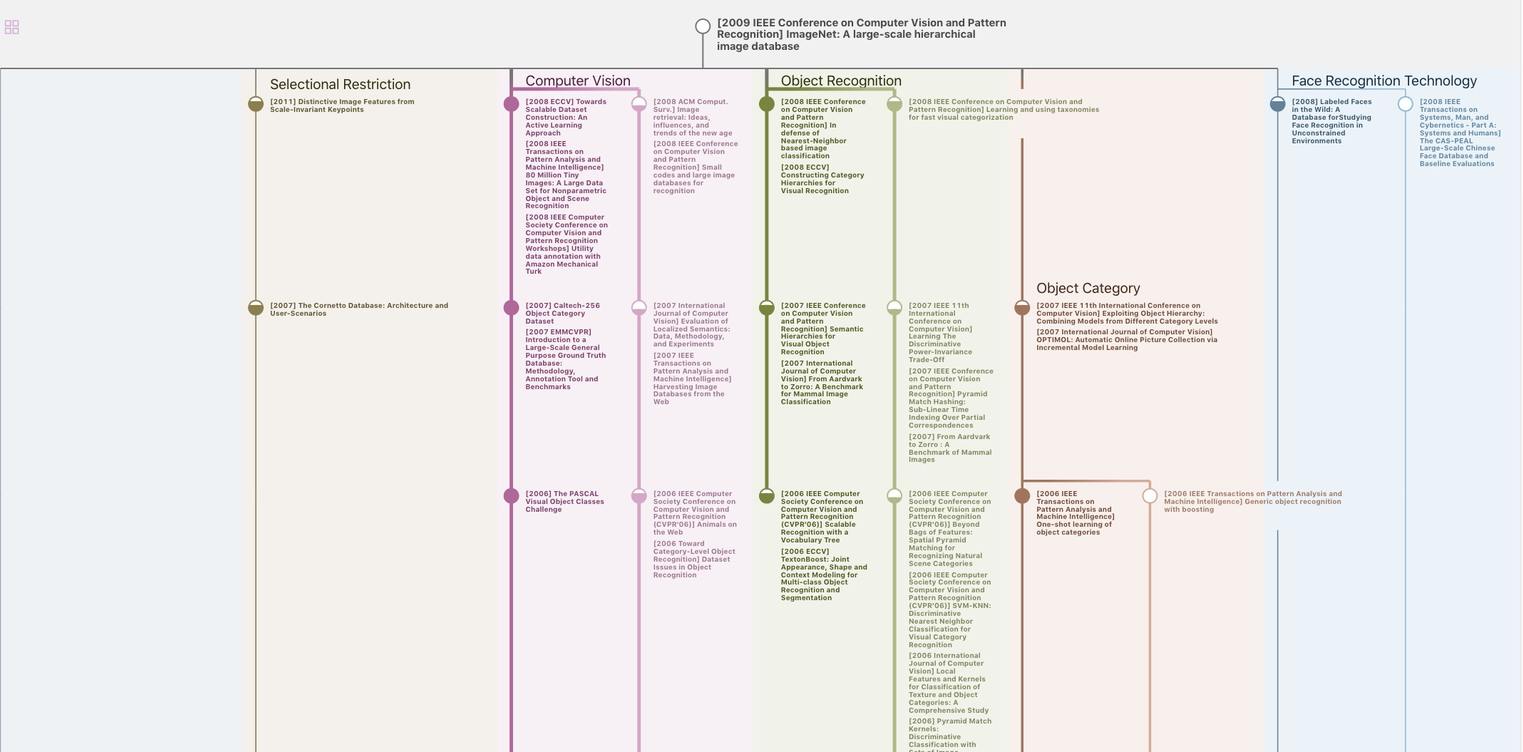
生成溯源树,研究论文发展脉络
Chat Paper
正在生成论文摘要