Deep Learning And Interactivity For Video Rotoscoping
2020 IEEE INTERNATIONAL CONFERENCE ON IMAGE PROCESSING (ICIP)(2020)
摘要
In this work we extend the idea of object co-segmentation [10] to perform interactive video segmentation. Our framework predicts the coordinates of vertices along the boundary of an object for two frames of a video simultaneously. The predicted vertices are interactive in nature and a user interaction on one frame assists the network to correct the predictions for both frames. We employ attention mechanism at the encoder stage and a simple combination network at the decoder stage which allows the network to perform this simultaneous correction efficiently. The framework is also robust to the distance between the two input frames as it can handle a distance of up to 50 frames in between the two inputs.We train our model on professional dataset, which consists pixel accurate annotations given by professional Roto artists. We test our model on DAVIS [15] and achieve state of the art results in both automatic and interactive mode surpassing Curve-GCN [11] and PolyRNN++ [1].
更多查看译文
关键词
encoder stage,decoder stage,Curve-GCN,video rotoscoping,interactive video segmentation,user interaction,deep learning,object co-segmentation,PolyRNN++,ResNet-50,video frames
AI 理解论文
溯源树
样例
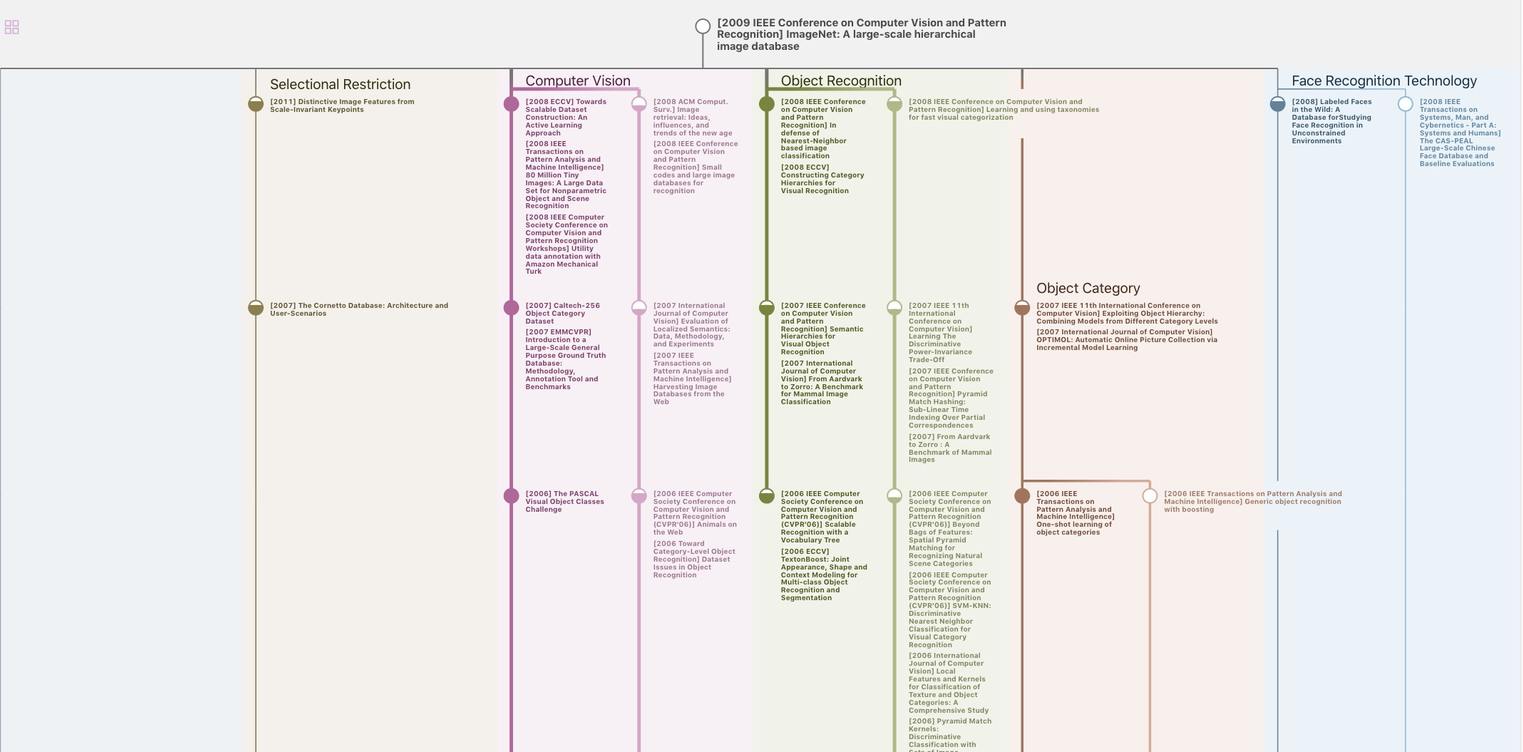
生成溯源树,研究论文发展脉络
Chat Paper
正在生成论文摘要