Guided Cnn Restoration With Explicitly Signaled Linear Combination
2020 IEEE INTERNATIONAL CONFERENCE ON IMAGE PROCESSING (ICIP)(2020)
摘要
State-of-the-art Convolutional Neural Network (CNN) based loop restoration generally involves a CNN structure with a large number of parameters and applies the CNN model to those degraded frames uniformly to generate their restored version, even though the contents within these frames are different. By contrast, in this paper, we propose a Guided CNN Restoration (GNR) scheme, where a CNN is used in conjunction with explicitly signaled guide parameters, with an aim to adapt the CNN model to different input contents. Specifically, the CNN architecture is designed such that the final restoration is constrained within the subspace generated by various output channels of the CNN, and meanwhile the weighting parameters for a linear combination of the output channels to obtain the final restoration are explicitly signaled by the encoders. The proposed GNR is incorporated into an AV1 encoder to replace the anchor in-loop filters and the weighting parameters are written into the encoded bitstream. Experimental results show that given a small CNN with 3, 312 parameters, the proposed approach achieves a BD-rate reduction of 3.06% over the AV1 anchor, while the traditional CNN-based method only achieves 1.39%.
更多查看译文
关键词
CNN, AV1, video coding, image restoration, in-loop filter
AI 理解论文
溯源树
样例
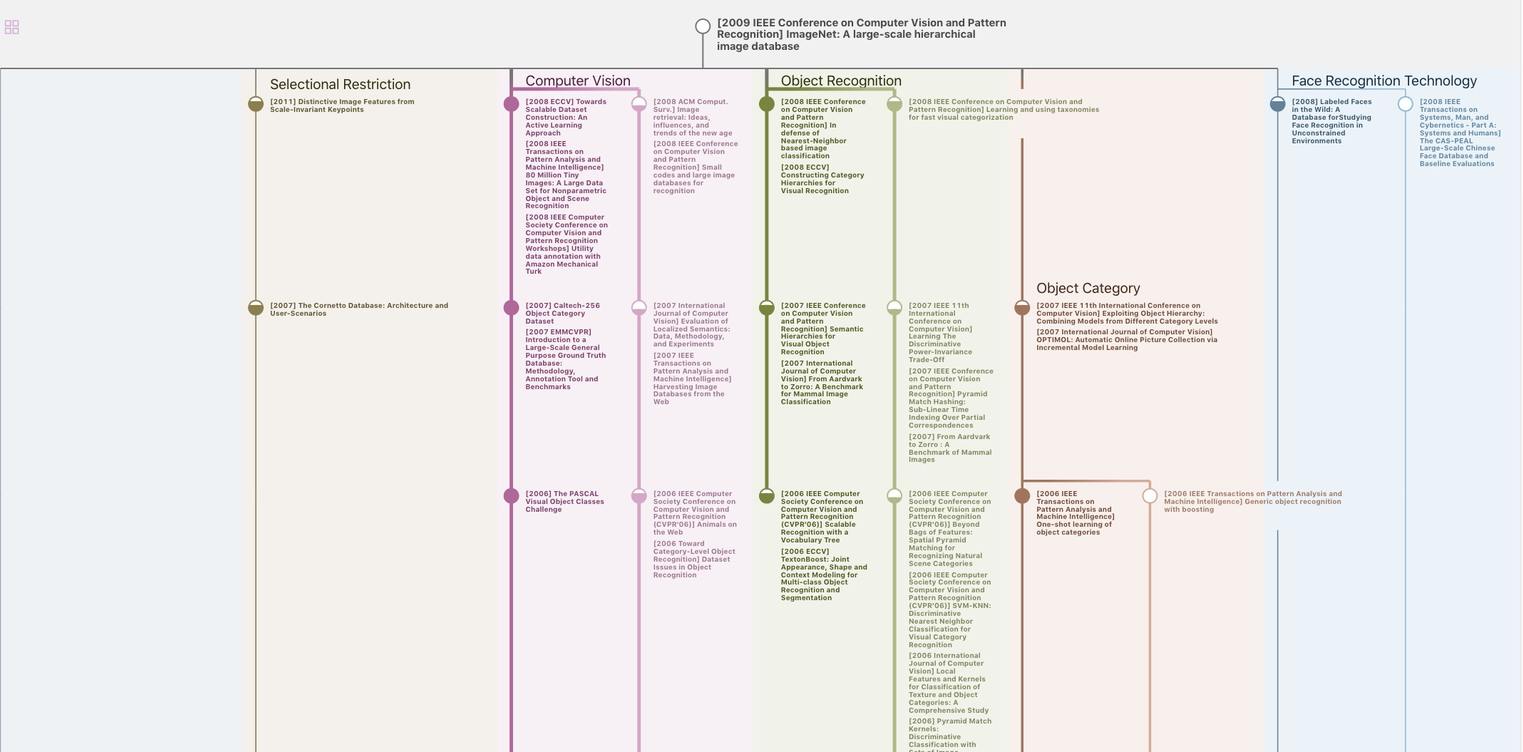
生成溯源树,研究论文发展脉络
Chat Paper
正在生成论文摘要