On the Vulnerability of Hyperdimensional Computing-Based Classifiers to Adversarial Attacks.
NSS(2020)
摘要
Hyperdimensional computing (HDC) has been emerging as a brain-inspired in-memory computing architecture, exhibiting ultra energy efficiency, low latency and strong robustness against hardware-induced bit errors. Nonetheless, state-of-the-art designs for HDC classifiers are mostly security-oblivious, raising concerns with their safety and immunity to adversarial inputs. In this paper, we study for the first time adversarial attacks on HDC classifiers and highlight that HDC classifiers can be vulnerable to even minimally-perturbed adversarial samples. Specifically, using handwritten digit classification as an example, we construct a HDC classifier and formulate a grey-box attack problem, where an attacker's goal is to mislead the target HDC classifier to produce erroneous prediction labels while keeping the amount of added perturbation noise as little as possible. Then, we propose a modified genetic algorithm to generate adversarial samples within a reasonably small number of queries, and further apply critical gene crossover and perturbation adjustment to limit the amount of perturbation noise. Our results show that adversarial images can successfully mislead the HDC classifier to produce wrong prediction labels with a high probability (i.e., 78% when the HDC classifier uses a fixed majority rule for decision).
更多查看译文
关键词
adversarial attacks,vulnerability,computing-based
AI 理解论文
溯源树
样例
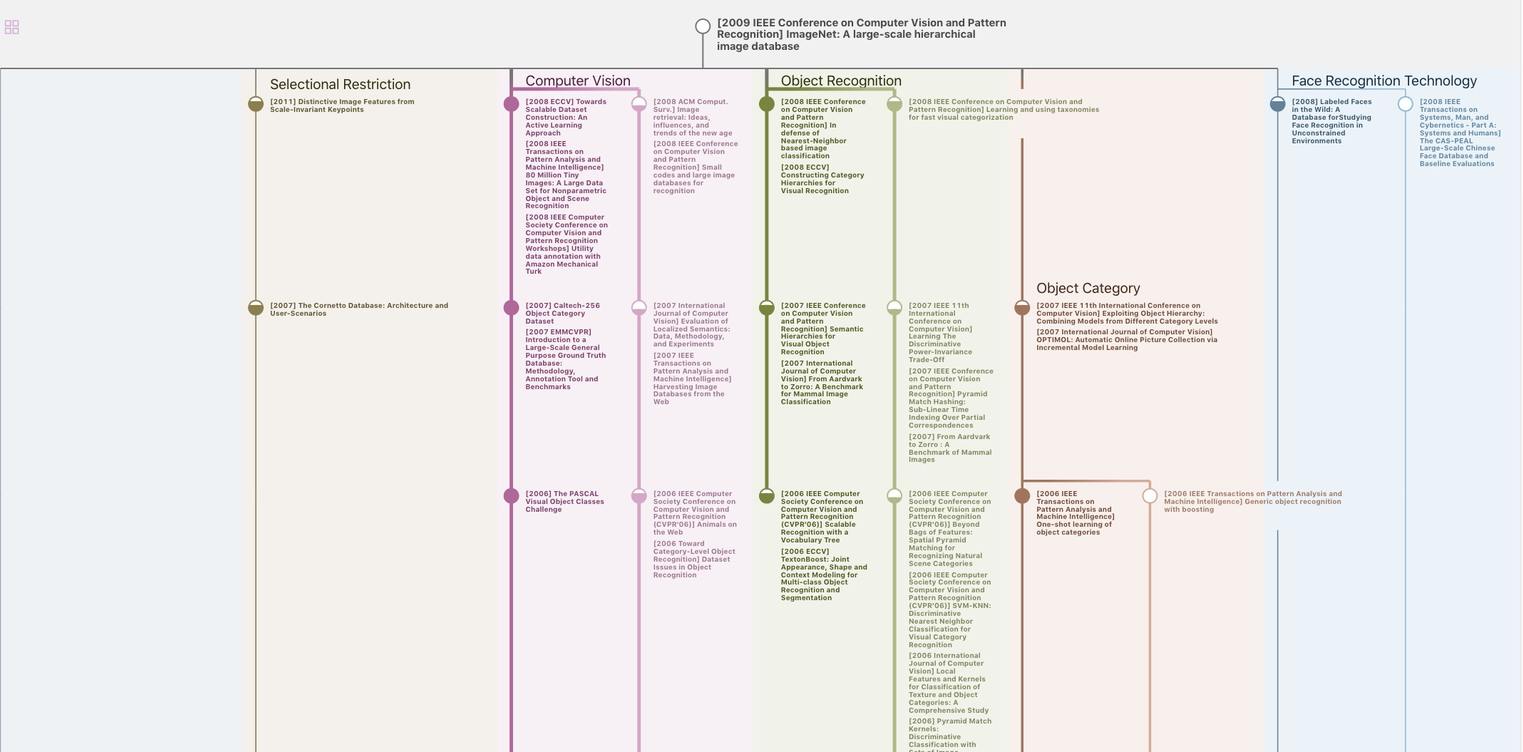
生成溯源树,研究论文发展脉络
Chat Paper
正在生成论文摘要