Dynamic Multi-channel Access in Wireless System with Deep Reinforcement Learning
2020 12th International Conference on Advanced Computational Intelligence (ICACI)(2020)
摘要
Dynamic spectrum access (DSA) has been considered a promising technology to address the scarcity of spectrum. In this paper, We investigate the use of deep reinforcement learning (DRL) to solve the DSA problem by maximizing the expected long-term normalized reward. Specifically, we propose a deep Q-network (DQN) based algorithm to solve dynamic multichannel access problem where there are multiple orthogonal channels in the wireless system. The problem is formulated as a Partially Observable Markov Decision Process (POMDP) with partially unknown system dynamics. We train the agent by interacting with the built system environment to find the optimal access policy of the single user and combine deep neural network to reduce the computational complexity caused by large action space. Finally, we compare our DRL approach with two conventional algorithms, and the simulation results show it can achieve near-optimal performance in a dynamic system, and we also compare our DQN algorithm with Double DQN (DDQN) algorithm which can achieve better performance and address overestimating action values.
更多查看译文
关键词
Dynamic Multi-channel Access,DRL,POMDP,Q-learning,DQN,DDQN
AI 理解论文
溯源树
样例
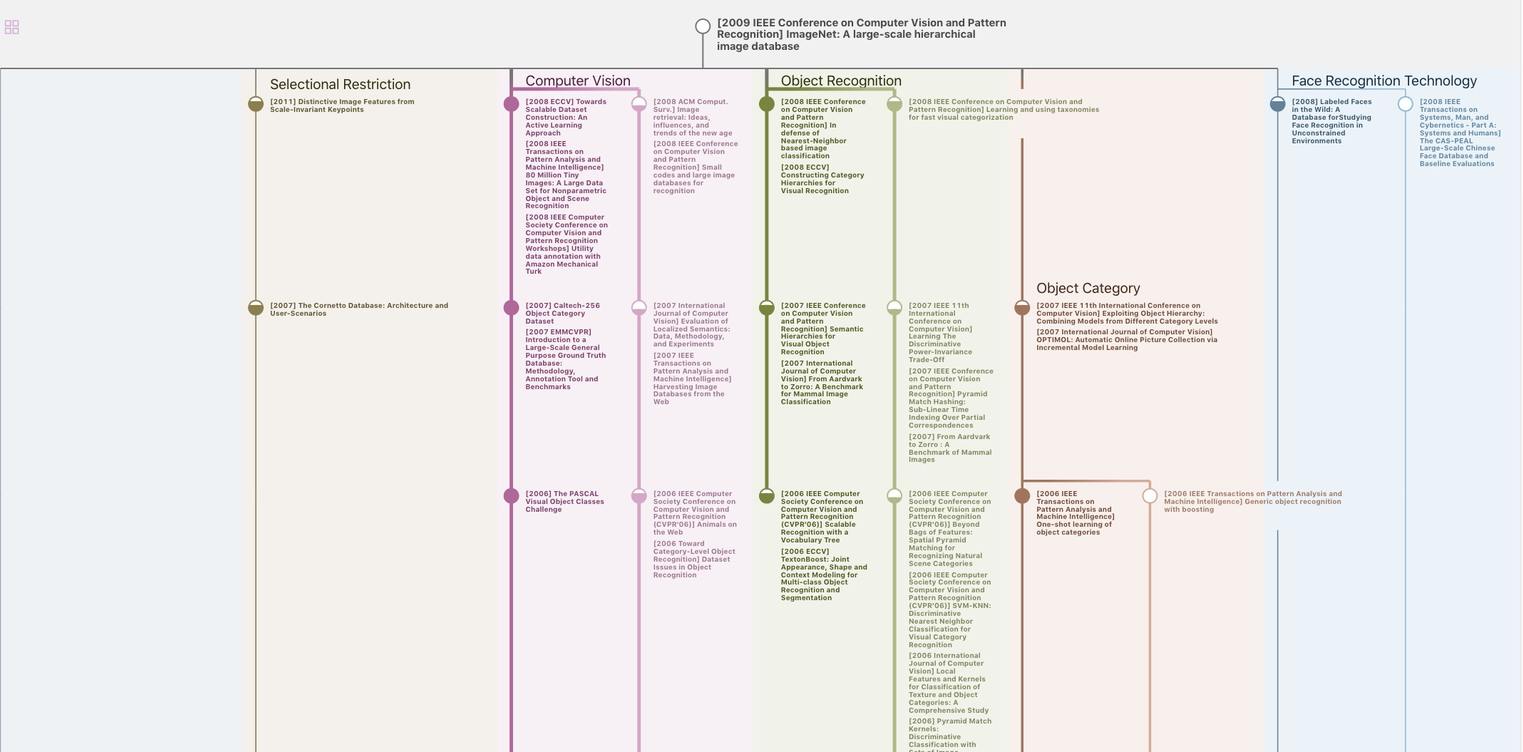
生成溯源树,研究论文发展脉络
Chat Paper
正在生成论文摘要