Overcoming Mobility Poverty with Shared Autonomous Vehicles - A Learning-Based Optimization Approach for Rotterdam Zuid.
ICCL(2020)
摘要
Residents of cities’ most disadvantaged areas face significant barriers to key life activities, such as employment, education, and healthcare, due to the lack of mobility options. Shared autonomous vehicles (SAVs) create an opportunity to overcome this problem. By learning user demand patterns, SAV providers can improve regional service levels by applying anticipatory relocation strategies that take into consideration when and where requests are more likely to appear. The nature of transportation demand, however, invariably creates learning biases towards servicing cities’ most affluent and densely populated areas, where alternative mobility choices already abound. As a result, current disadvantaged regions may end up perpetually underserviced, therefore preventing all city residents from enjoying the benefits of autonomous mobility-on-demand (AMoD) systems equally. In this study, we propose an anticipatory rebalancing policy based on an approximate dynamic programming (ADP) formulation that processes historical demand data to estimate value functions of future system states iteratively. We investigate to which extent manipulating cost settings, in terms of subsidies and penalties, can adjust the demand patterns naturally incorporated into value functions to improve service levels of disadvantaged areas. We show for a case study in the city of Rotterdam, The Netherlands, that the proposed method can harness these cost schemes to better cater to users departing from these disadvantaged areas, substantially outperforming myopic and reactive benchmark policies.
更多查看译文
关键词
shared autonomous vehicles,mobility poverty,learning-based
AI 理解论文
溯源树
样例
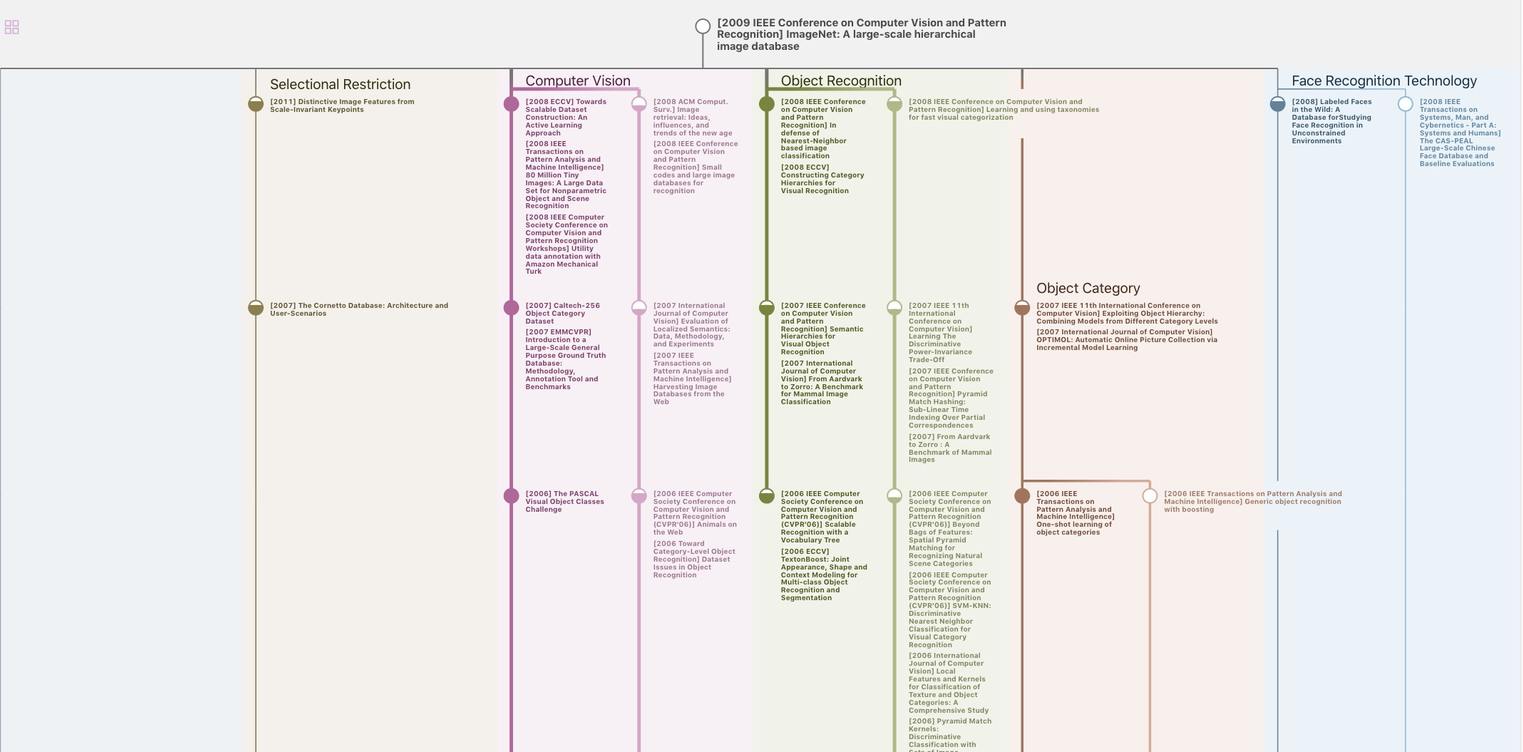
生成溯源树,研究论文发展脉络
Chat Paper
正在生成论文摘要