Towards an Explainable Mortality Prediction Model
2020 IEEE 30th International Workshop on Machine Learning for Signal Processing (MLSP)(2020)
摘要
Influence functions are analytical tools from robust statistics that can help interpret the decisions of black-box machine learning models. Influence functions can be used to attribute changes in the loss function due to small perturbations in the input features. The current work on using influence functions is limited to the features available before the last layer of deep neural networks (DNNs). We extend the influence function approximation to DNNs by computing gradients in an end-to-end manner and relate changes in the loss function to individual input features using an efficient algorithm. We propose an accurate mortality prediction neural network and show the effectiveness of extended influence functions on the eICU dataset. The features chosen by proposed extended influence functions were more like those selected by human experts than those chosen by other traditional methods.
更多查看译文
关键词
Explainable AI,XAI,Bioinformatics,Influence Functions,Robust Statistics,Mortality Prediction,Explainable deep learning
AI 理解论文
溯源树
样例
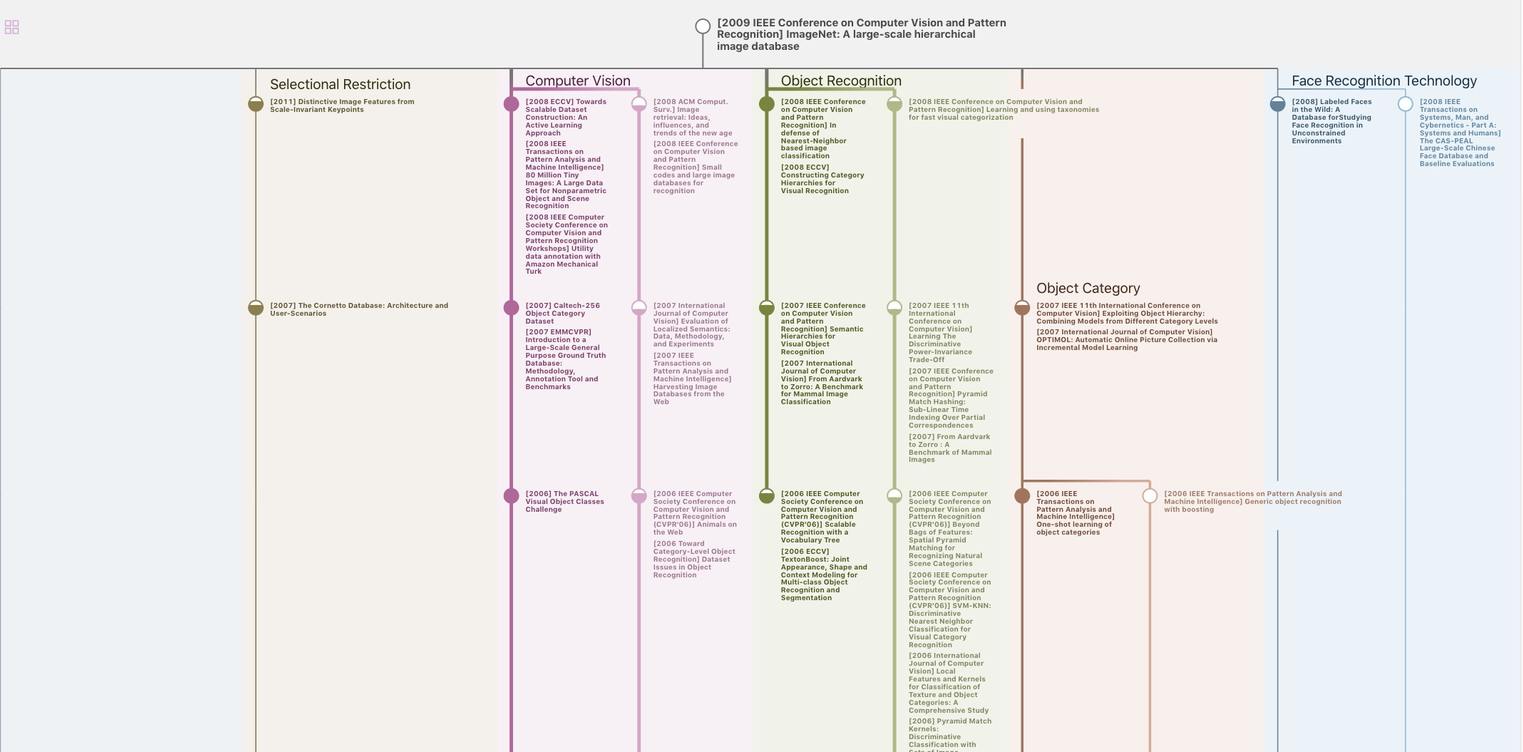
生成溯源树,研究论文发展脉络
Chat Paper
正在生成论文摘要