A Joint Dynamic Ranking System with DNN and Vector-based Clustering Bandit
RECSYS(2020)
摘要
ABSTRACT The ad-ranking module is the core of the advertising recommender system. Existing ad-ranking modules are mainly based on the deep neural network click-through rate prediction model. Recently an innovative ad-ranking paradigm called DNN-MAB has been introduced to address DNN-only paradigms’ weakness in perceiving highly dynamic user intent over time. We introduce the DNN-MAB paradigm into our ad-ranking system to alleviate the Matthew effect that harms the user experience. Due to data sparsity, however, the actual performance of DNN-MAB is lower than expected. In this paper, we propose an innovative ad-ranking paradigm called DNN-VMAB to solve these problems. Based on vectorization and clustering, it utilizes latent collaborative information in user behavior data to find a set of ads with higher relativity and diversity. As an integration of the essences of classical collaborative filtering, deep click-through rate prediction model, and contextual multi-armed bandit, it can improve platform revenue and user experience. Both offline and online experiments show the advantage of our new algorithm over DNN-MAB and some other existing algorithms.
更多查看译文
关键词
Learning to rank, Multi-arm bandits
AI 理解论文
溯源树
样例
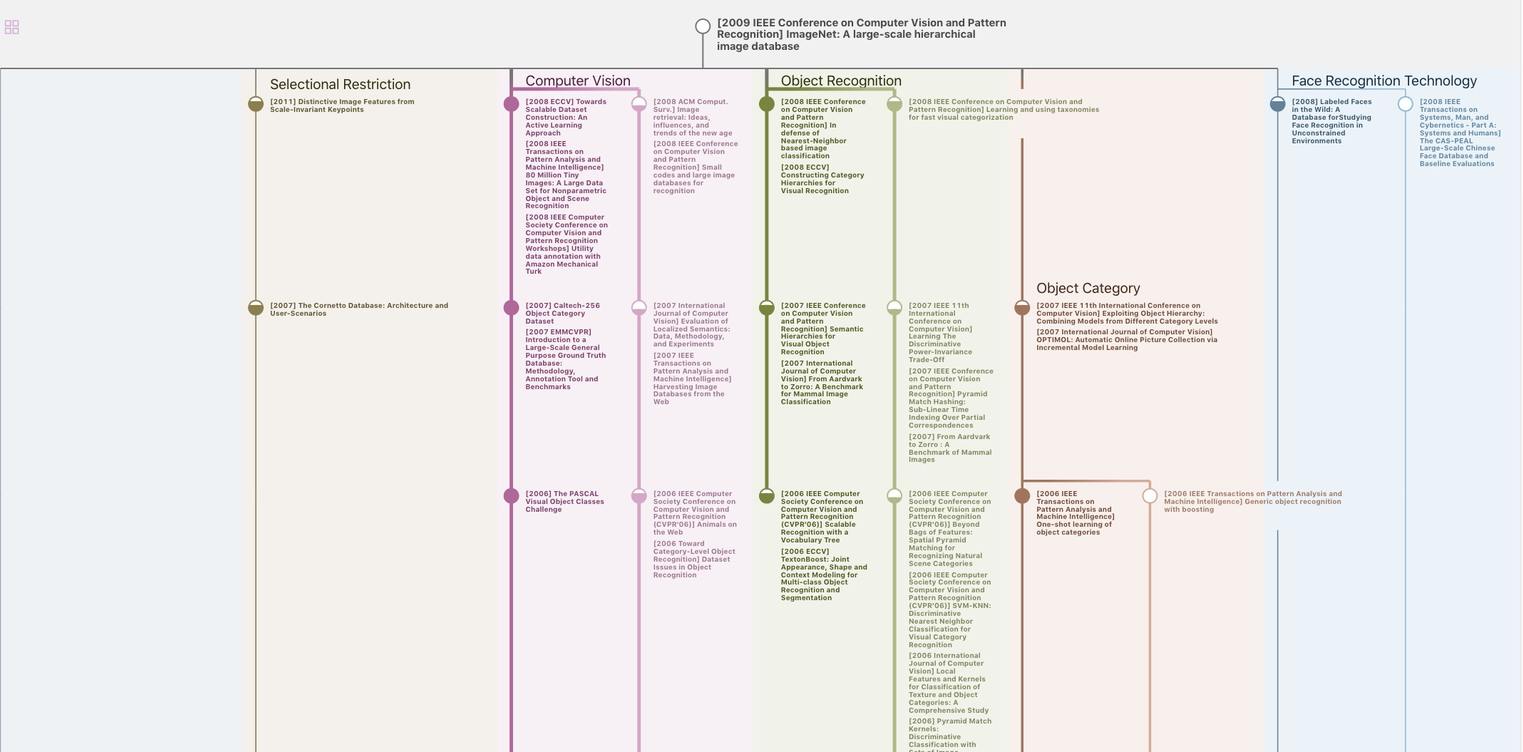
生成溯源树,研究论文发展脉络
Chat Paper
正在生成论文摘要