Resource Allocation for Infrastructure Resilience using Artificial Neural Networks
2020 IEEE 32nd International Conference on Tools with Artificial Intelligence (ICTAI)(2020)
摘要
From an optimization point of view, resource allocation is one of the cornerstones of research for addressing problems commonly arising in applications such as power outages and traffic jams. In this paper, we take a data-driven approach to estimate an optimal nodal restoration sequence for immediate recovery of infrastructure networks after natural disasters such as earthquakes. Data is generated from td-INDP, a high-fidelity simulator of optimal restoration strategies for interdependent networks, and employ artificial neural networks to approximate those strategies. Despite the fact that the underlying problem is NP-complete, the restoration sequences obtained by our method are observed to be nearly optimal. In addition, by training multiple models—the so-called estimators—for a variety of resource availability levels, our proposed method balances a tradeoff between resource utilization and restoration time. Decision-makers can use our trained models to allocate resources more efficiently before and after contingencies, and in turn, improve the community resilience. Besides their predictive power, such trained estimators unravel the effect of interdependencies among different nodal functionalities in the restoration strategies. We showcase our methodology by the real-world interdependent infrastructure of Shelby County, TN.
更多查看译文
关键词
Resource Allocation, Infrastructure Resilience, Artificial Neural Networks
AI 理解论文
溯源树
样例
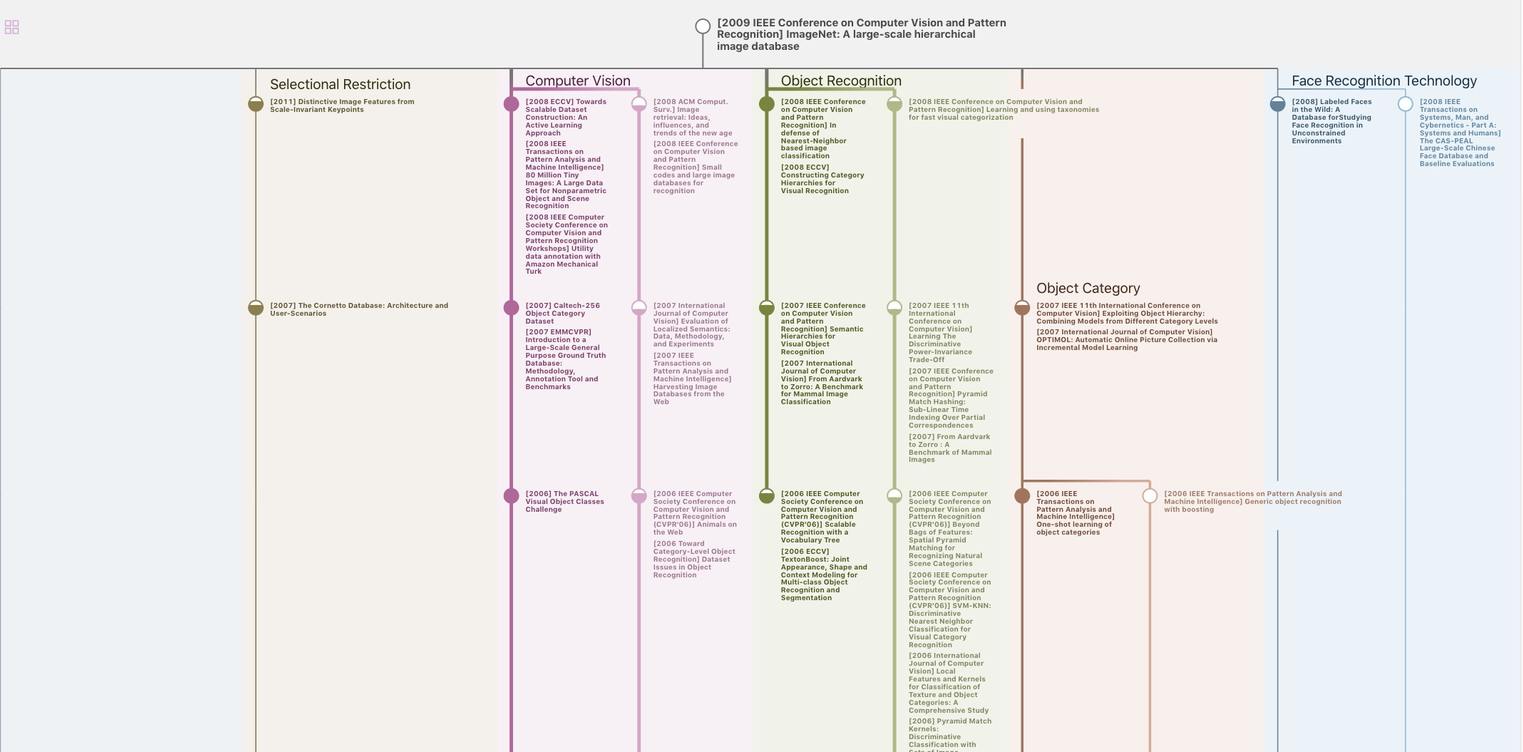
生成溯源树,研究论文发展脉络
Chat Paper
正在生成论文摘要