Infant Sound Classification on Multi-stage CNNs with Hybrid Features and Prior Knowledge.
AIMS(2020)
摘要
We propose an approach of generating a hybrid feature set and using prior knowledge in a multi-stage CNNs for robust infant sound classification. The dominant and auxiliary features within the set are beneficial to enlarge the coverage as well as keeping a good resolution for modeling the diversity of variations within infant sound. The novel multi-stage CNNs method work together with prior knowledge constraints in decision making to overcome the limited data problem in infant sound classification. Prior knowledge either from rules or from statistical results provides a good guidance for searching and classification. The effectiveness of proposed method is evaluated on commonly used Dustan Baby Language Database and Baby Chillanto Database. It gives an encouraging reduction of 4.14% absolute classification error rate compared with the results from the best model using one-stage CNN. In addition, on Baby Chillanto Database, a significant absolute error reduction of 5.33% is achieved compared to one-stage CNN and it outperforms all other existing related studies.
更多查看译文
AI 理解论文
溯源树
样例
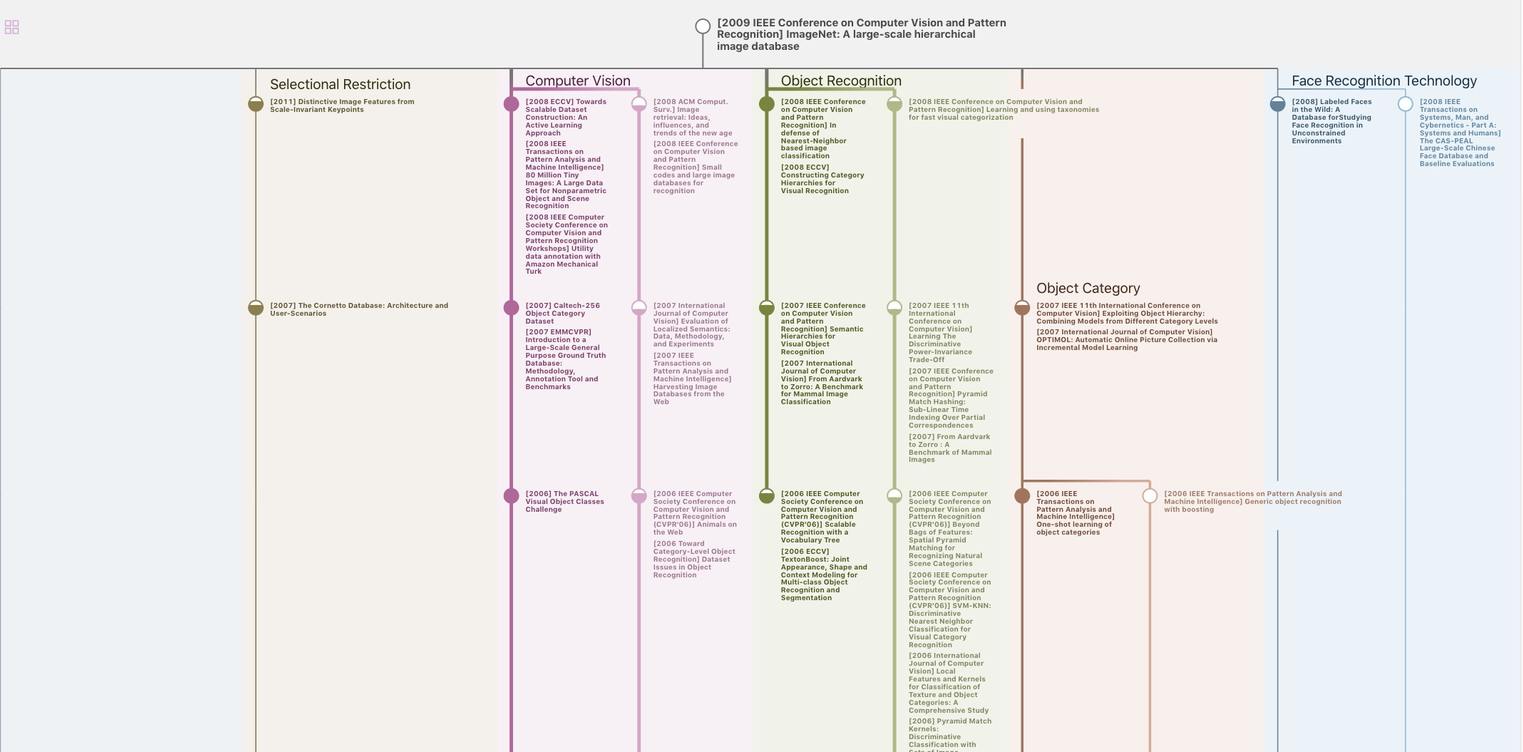
生成溯源树,研究论文发展脉络
Chat Paper
正在生成论文摘要