Learning Multi-modal Representations of Narrative Multimedia - a Case Study of Webtoons.
RACS(2020)
Abstract
This study aims to learn task-agnostic representations of narrative multimedia. The existing studies focused on only stories in the narrative multimedia without considering their physical features. We propose a method for incorporating multi-modal features of the narrative multimedia into a unified vector representation. For narrative features, we embed character networks as with the existing studies. Textual features can be represented using the LSTM (Long-Short Term Memory) autoencoder. We apply the convolutional autoencoder to visual features. The convolutional autoencoder also can be used for the spectrograms of audible features. To combine these features, we propose two methods: early fusion and late fusion. The early fusion method composes representations of features on each scene. Then, we learn representations of a narrative work by predicting time-sequential changes in the features. The late fusion method concatenates feature vectors that are trained for allover the narrative work. Finally, we apply the proposed methods on webtoons (i.e., comics that are serially published through the web). The proposed methods have been evaluated by applying the vector representations to predicting the preferences of users for the webtoons.
MoreTranslated text
Key words
narrative,learning,multi-modal
AI Read Science
Must-Reading Tree
Example
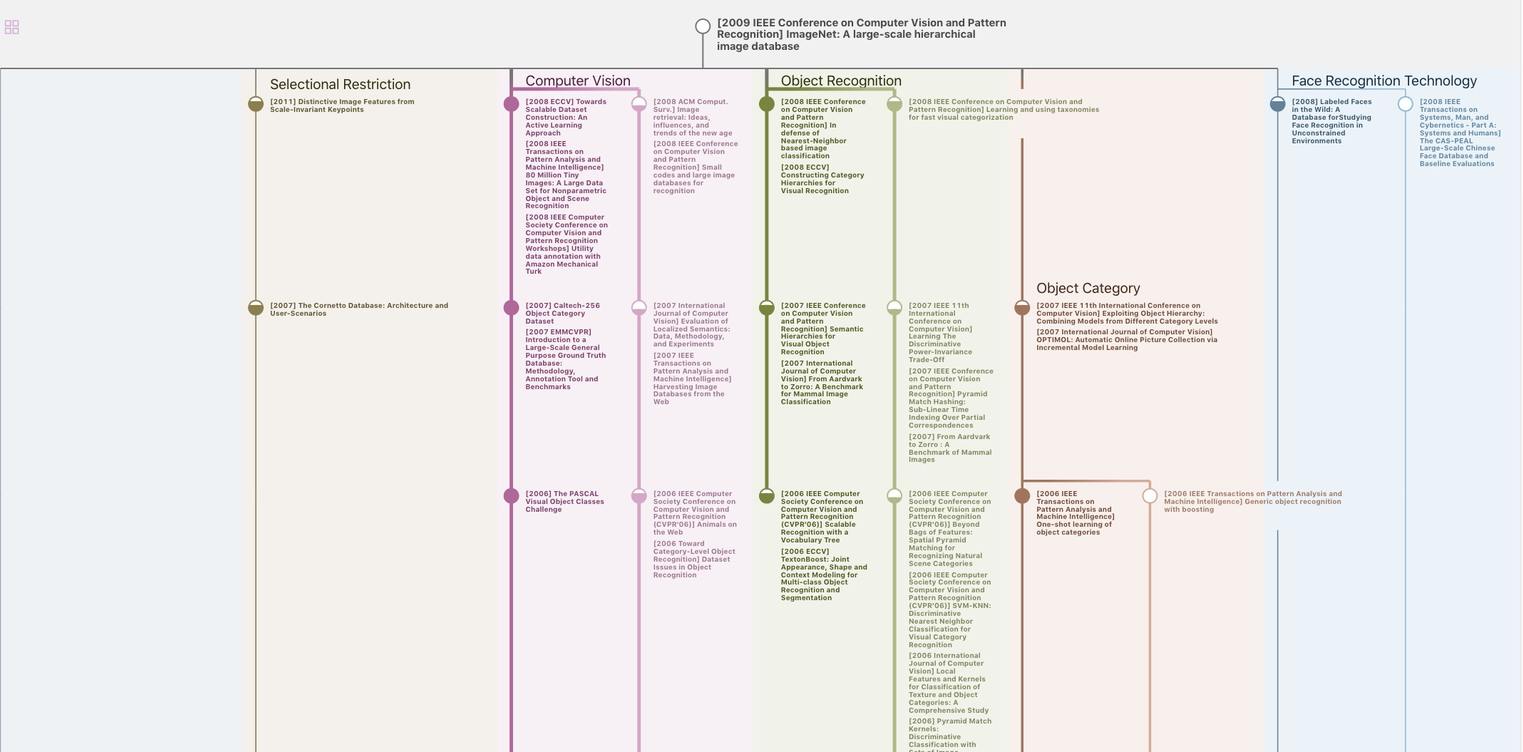
Generate MRT to find the research sequence of this paper
Chat Paper
Summary is being generated by the instructions you defined