Evading a Machine Learning-based Intrusion Detection System through Adversarial Perturbations.
RACS(2020)
摘要
Machine-learning based Intrusion Detection and Prevention Systems provide significant value to organizations because they can efficiently detect previously unseen variations of known threats, new threats related to known malware or even zero-day malware, unrelated to any other known threats. However, while such systems prove invaluable to security personnel, researchers have observed that data subject to inspection by behavioral analysis can be perturbed in order to evade detection. We investigated the use of adversarial techniques for adapting the communication patterns between botnet malware and control unit in order to evaluate the robustness of an existing Network Behavioral Analysis solution. We implemented a packet parser that let us extract and edit certain properties of network flows and automated an approach for conducting a grey-box testing scheme of Stratosphere Linux IPS. As part of our implementation, we provided several techniques for providing perturbation to network flow parameters, including a Simultaneous Perturbation Stochastic Approximation method, which was able to produce sufficiently perturbed network flow patterns while adhering to an underlying objective function. Our results showed that network flow parameters could indeed be perturbed to ultimately enable evasion of intrusion detection based on the detection models that were used with the Intrusion Detection System. Additionally, we demonstrated that it was possible to combine evading detection with techniques for optimization problems that aimed to minimize the magnitude of perturbation to network flows, effectively enabling adaptive network flow behavior.
更多查看译文
AI 理解论文
溯源树
样例
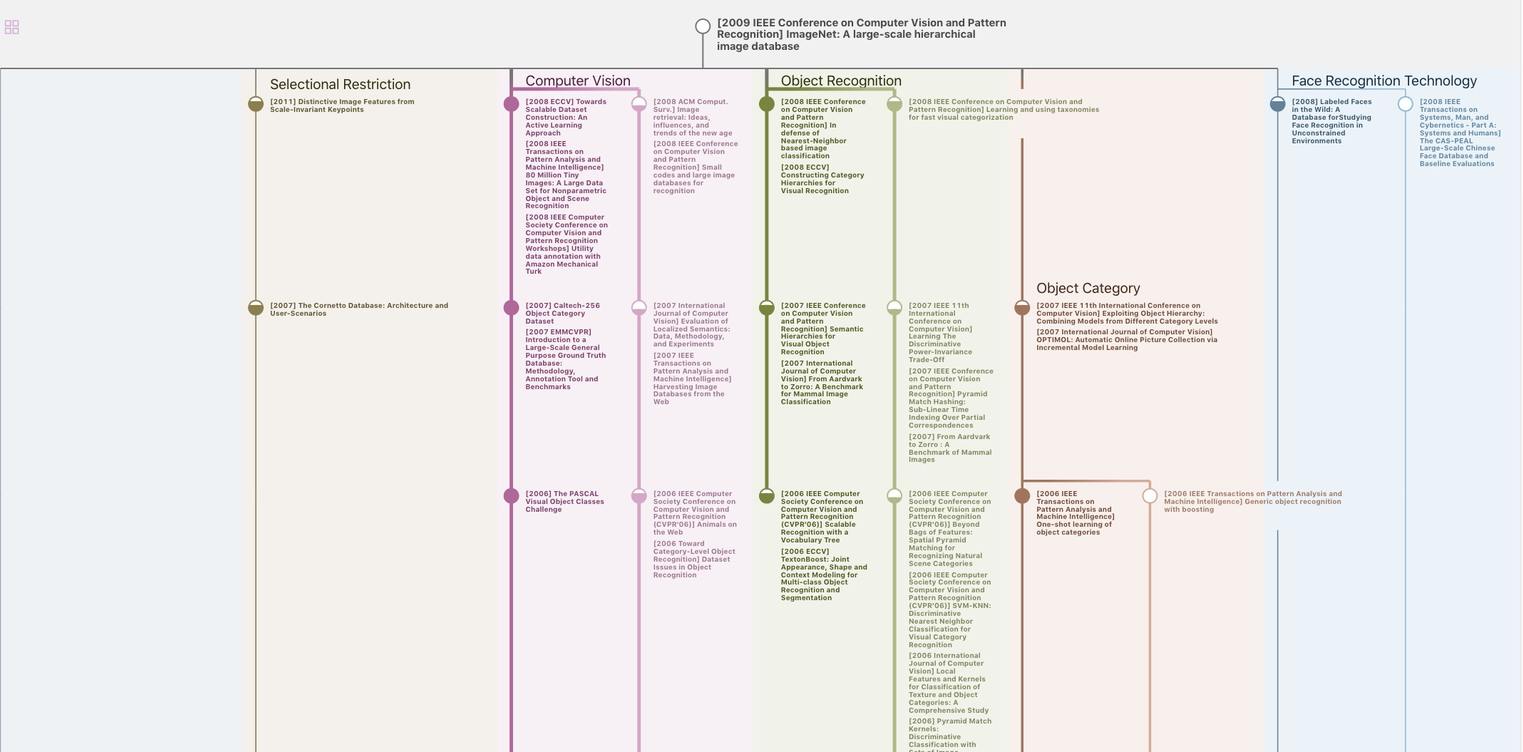
生成溯源树,研究论文发展脉络
Chat Paper
正在生成论文摘要