Deep Learning For Early Damage Detection Of Tailing Pipes Joints With A Robotic Device
2020 IEEE 16TH INTERNATIONAL CONFERENCE ON AUTOMATION SCIENCE AND ENGINEERING (CASE)(2020)
摘要
In the mining industry, it is usual to employ several kilometers of pipes to carry tailing from the plant to a dam. Only in the Salobo Mine, a copper operation in the Amazon forest from Vale S.A., there are more than three and a half kilometers of tailing pipes. Since the material passing through the tailing pipe causes an abrasion effect that could lead to failures, regular inspections are needed. However, given the risky environment to perform manual inspections, a teleoperated or autonomous robot is a crucial tool to keep track of the pipe health. In this work, we propose a deep-learning methodology to process the data stream of images from the robot, aiming to detect early failures directly on the onboard computer of the device in real-time. Multiple architectures of deep-learning image classification were evaluated to detect the anomalies. We validated the early damage detection accuracy and pinpointed the approximate location of the anomalies using the Class Activation Mapping of the networks. Then, we tested the runtime for the network architectures that obtained the best results on different hardware to analyze the need for a GPU onboard in the robot. Moreover, we also trained a Single Shot object Detector to find the boundaries of the pipe joints, which means that the anomaly classification is performed only when a joint is detected. Our results show that it is possible to build an automatic anomaly detection system in the software of the robot.
更多查看译文
关键词
onboard computer,single shot object detector,network architectures,class activation mapping,data stream,Vale S.A.,teleoperated robot,manual inspections,Amazon forest,copper operation,Salobo Mine,mining industry,robotic device,tailing pipes joints,automatic anomaly detection system,early damage detection accuracy,deep-learning image classification,early failures,pipe health,autonomous robot
AI 理解论文
溯源树
样例
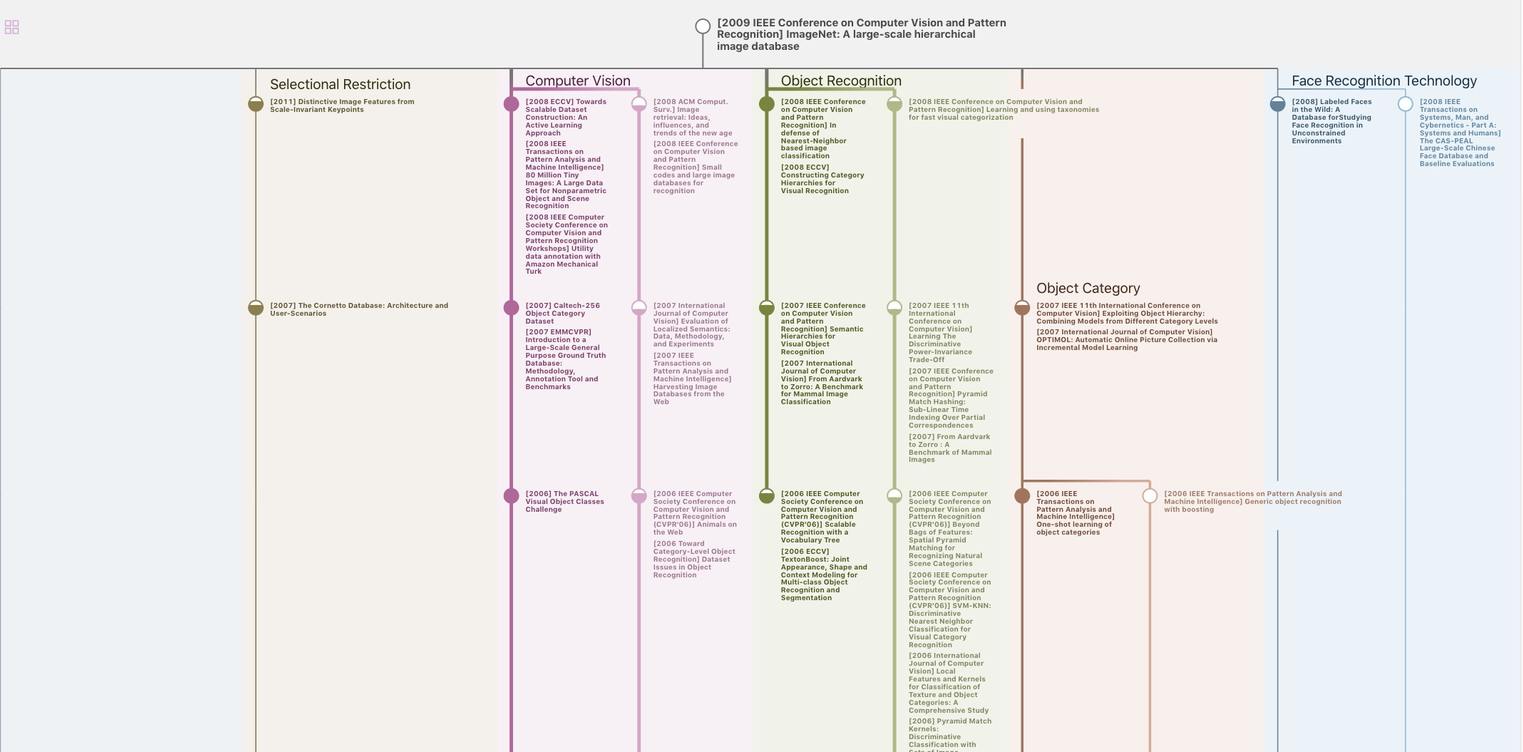
生成溯源树,研究论文发展脉络
Chat Paper
正在生成论文摘要