Sequencing, Combining And Sampling Classifiers To Help Find Needles In Haystacks
ECAI 2020: 24TH EUROPEAN CONFERENCE ON ARTIFICIAL INTELLIGENCE(2020)
摘要
Many binary prediction situations involve imbalanced datasets where the ratio of the minority class over the majority class is very low. This is especially true when dealing with problems looking to use machine learning to better detect fraud, errors or exceptions. In this paper, we address the problem of extreme imbalance, i.e. where the imbalance ratio of majority over minority instances exceeds 500. Given the scarcity of minority examples, oversampling is not sensible due to expensive computational cost. Hence, we explore and expand undersampling approaches. Specifically, we propose a modeling framework (i.e., sequence of modeling steps) that seeks to leverage as much training data as possible. Our results indicate the better trade-off between the false positives and false negatives, which makes it more suitable for real-life application.
更多查看译文
AI 理解论文
溯源树
样例
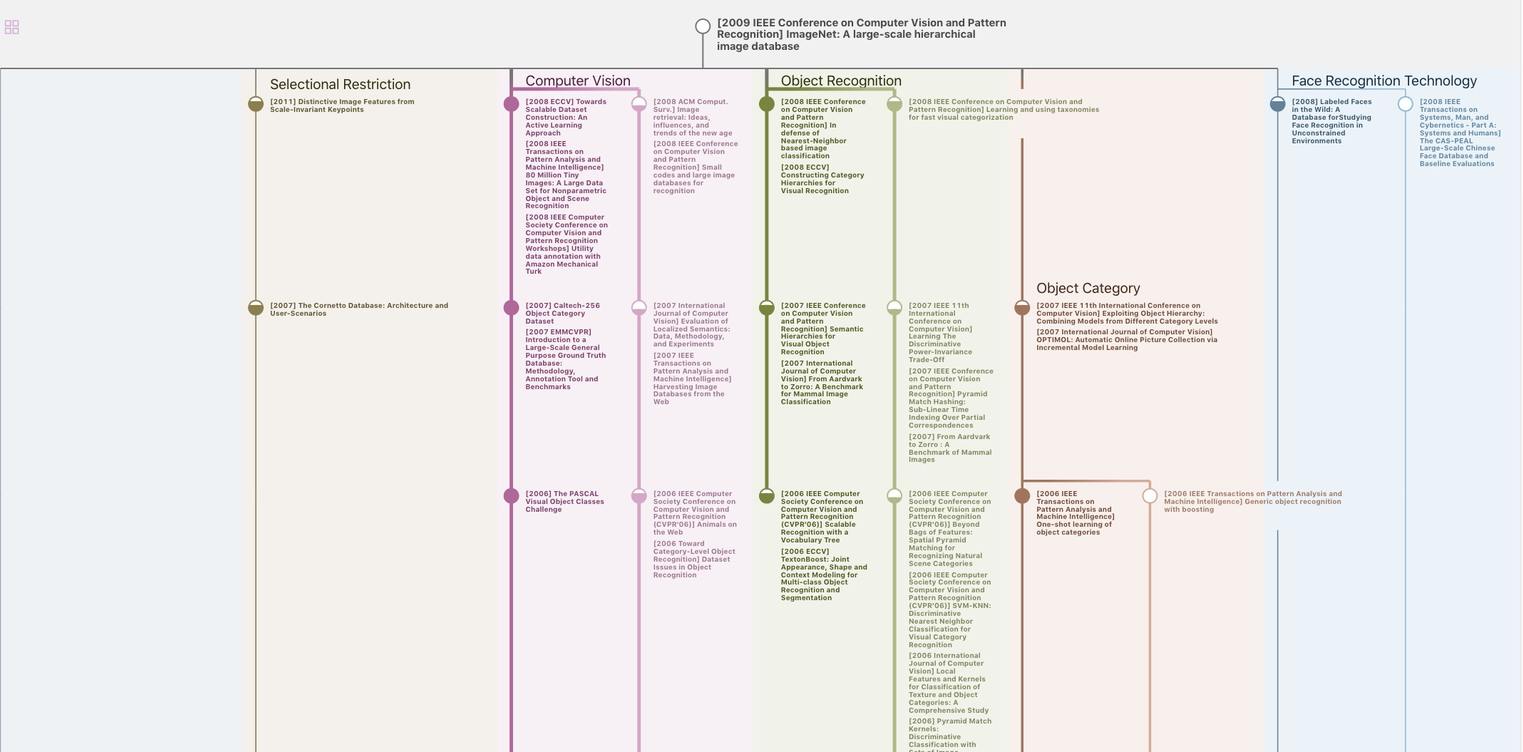
生成溯源树,研究论文发展脉络
Chat Paper
正在生成论文摘要