Learning Temporal Action Models Via Constraint Programming
ECAI 2020: 24TH EUROPEAN CONFERENCE ON ARTIFICIAL INTELLIGENCE(2020)
摘要
We present a solver-independent Constraint Programming (CP) formulation for learning action models in temporal planning scenarios beyond PDDL2.1. Inspired by the CP approach for temporal planning, our formulation bases on a temporal plan trace and represents observations (as time-stamped states), actions, causal-link relationships, condition threats and effect interferences. This formulation is very expressive and supports a wide range of input knowledge. It also evidences the connection between the tasks of: i) action model learning, ii) plan validation, and iii) plan synthesis. Our experiments evaluate the quality of the learned models under different learning scenarios and in different planning domains.
更多查看译文
AI 理解论文
溯源树
样例
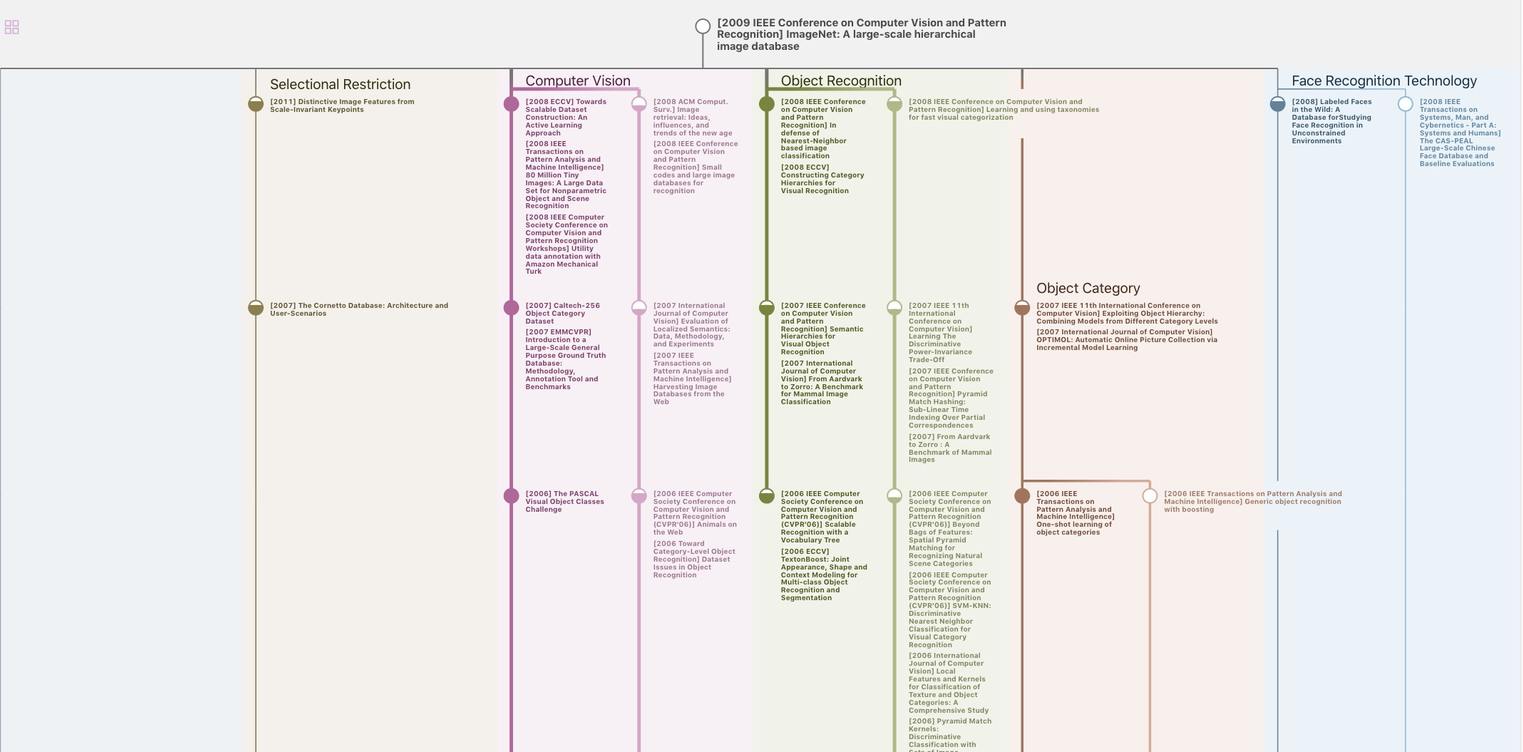
生成溯源树,研究论文发展脉络
Chat Paper
正在生成论文摘要