Marginal Association Probabilities For Multiple Extended Objects Without Enumeration Of Measurement Partitions
PROCEEDINGS OF 2020 23RD INTERNATIONAL CONFERENCE ON INFORMATION FUSION (FUSION 2020)(2020)
摘要
In the case of high-resolution or near field sensors, an object normally gives rise to multiple measurements per scan. One of the key tasks in tracking such objects is to differentiate the origins of the measurements. In this work, a new data association approach for extended object tracking, which is inspired by Joint Integrated Probabilistic Data Association (JIPDA), is proposed. The key idea is to calculate marginal association probabilities for individual measurements (instead of considering measurement partitions). Our problem formulation allows us to obtain the marginal association probabilities without collective exhaustion of association hypotheses and partitions. The proposed data association method is illustrated first using a simulation with Gaussian distributed measurements. Combined with an extended object measurement model, the data association quality is further assessed in a simulation and an experiment by tracking pedestrians using Lidar data from the KITTI dataset.
更多查看译文
关键词
KITTI dataset,Lidar data,tracking pedestrians,extended object measurement model,Gaussian distributed measurements,data association method,association hypotheses,individual measurements,joint integrated probabilistic data association,extended object tracking,data association approach,multiple extended objects,marginal association probabilities,data association quality
AI 理解论文
溯源树
样例
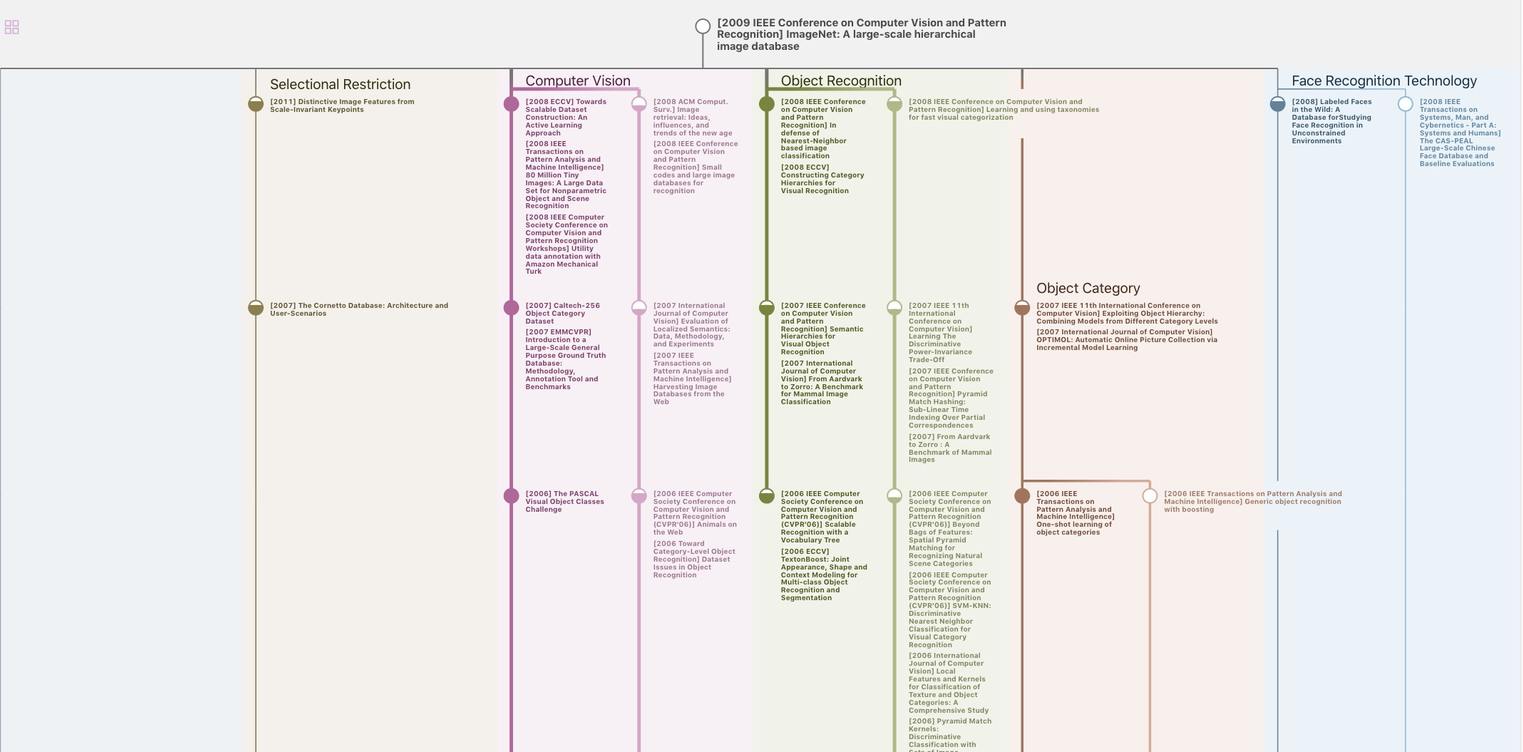
生成溯源树,研究论文发展脉络
Chat Paper
正在生成论文摘要