Reasoning With Interval-Valued Probabilities
PROCEEDINGS OF 2020 23RD INTERNATIONAL CONFERENCE ON INFORMATION FUSION (FUSION 2020)(2020)
Abstract
Adequate representative statistical training data needed for machine learning algorithms are often unavailable and, when available, they are often mired in incomplete/missing data. Imputation of such data must be guided by the relationships among different variables and/or by data 'missingness' mechanisms. Interval-valued (IV) probabilities are better suited in situations where such information is unavailable. We take the viewpoint that IV probabilities (IVPs) emerge from a single underlying probability distribution about which one has only partial information. PrBounds, the IVPs that this vantage point engenders, offer a fresh perspective of the IV counterpart notions of conditioning and independence and enable reasoning to be carried out in much the same manner as one would with probabilities. When the attribute values are unknown/missing or are known to lie within a set of values, PrBounds can be efficiently learnt by a frequency counting method. The probabilities associated with an arbitrary imputation strategy, including the underlying 'true' probabilities, are guaranteed to lie within the PrBounds learnt in this manner. We present an experiment to illustrate the proposed framework.
MoreTranslated text
Key words
Incomplete data, missing data, data imputation, interval-valued probabilities
AI Read Science
Must-Reading Tree
Example
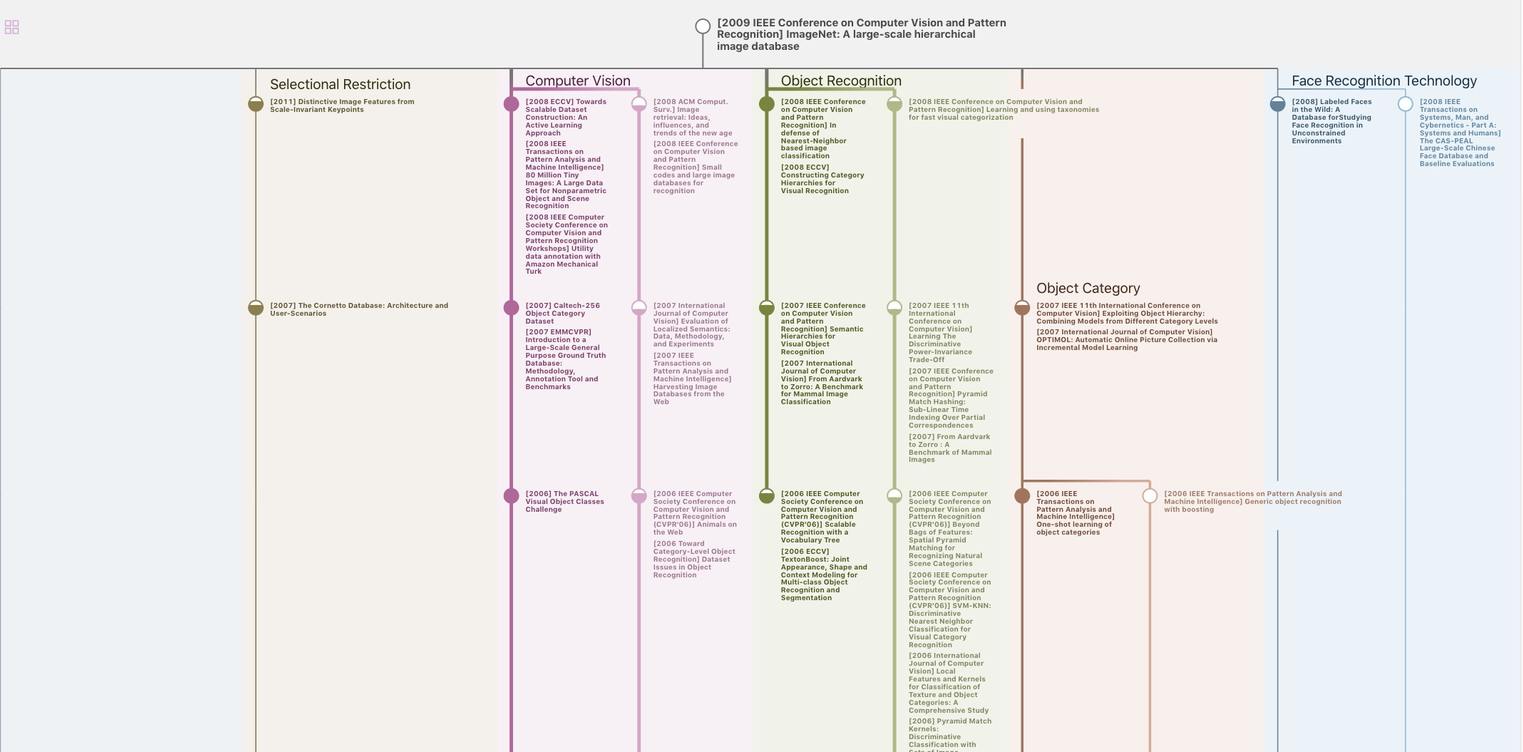
Generate MRT to find the research sequence of this paper
Chat Paper
Summary is being generated by the instructions you defined