AA-ResNet - Energy Efficient All-Analog ResNet Accelerator.
MWSCAS(2020)
摘要
High energy efficiency is a major concern for emerging machine learning accelerators designed for IoT edge computing. Recent studies propose in-memory and mixed-signal approaches to minimize energy overhead resulting from frequent memory accesses and extensive digital computation. However, their energy efficiency gain is often limited by the overhead of digital-to-analog and analog-to-digital conversions at the boundary of the compute-memory. In this paper, we propose a new in-memory accelerator that performs all computation in the analog domain for a large, multi-level neural network (NN) for the first time avoiding any digital-to-analog or analog-to-digital conversion overhead. We propose an all-analog ResNet (AAResNet) accelerator in 28-nm CMOS, achieving an energy efficiency of 1.2 µJ/inference and inference rate of 325K images/s for the CIFAR-10 and SVHN datasets in SPICE simulation.
更多查看译文
关键词
machine learning accelerator, in-memory computing, analog computing, deep residual learning
AI 理解论文
溯源树
样例
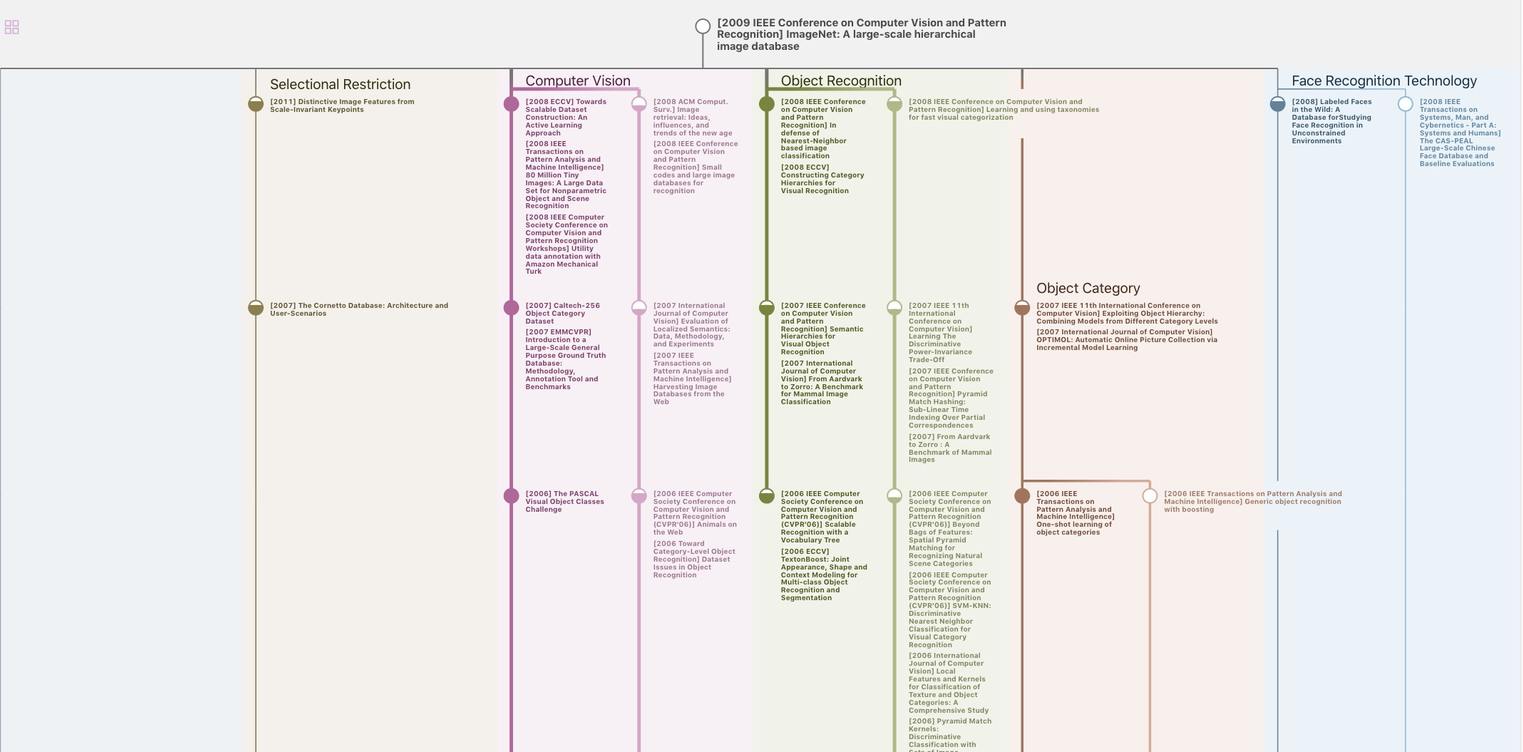
生成溯源树,研究论文发展脉络
Chat Paper
正在生成论文摘要