A New Integer Linear Programming Formulation to the Inverse QSAR/QSPR for Acyclic Chemical Compounds Using Skeleton Trees
IEA/AIE(2020)
摘要
Computer-aided drug design is one of important application areas of intelligent systems. Recently a novel method has been proposed for inverse QSAR/QSPR using both artificial neural networks (ANN) and mixed integer linear programming (MILP), where inverse QSAR/QSPR is a major approach for drug design. This method consists of two phases: In the first phase, a feature function f is defined so that each chemical compound G is converted into a vector f(G) of several descriptors of G, and a prediction function \(\psi \) is constructed with an ANN so that \(\psi (f(G))\) takes a value nearly equal to a given chemical property \(\pi \) for many chemical compounds G in a data set. In the second phase, given a target value \(y^*\) of the chemical property \(\pi \), a chemical structure \(G^*\) is inferred in the following way. An MILP \(\mathcal {M}\) is formulated so that \(\mathcal {M}\) admits a feasible solution \((x^*,y^*)\) if and only if there exist vectors \(x^*\), \(y^*\) and a chemical compound \(G^*\) such that \(\psi (x^*)=y^*\) and \(f(G^*)=x^*\). The method has been implemented for inferring acyclic chemical compounds. In this paper, we propose a new MILP for inferring acyclic chemical compounds by introducing a novel concept, skeleton tree, and conducted computational experiments. The results suggest that the proposed method outperforms the existing method when the diameter of graphs is up to around 6 to 8. For an instance for inferring acyclic chemical compounds with 38 non-hydrogen atoms from C, O and S and diameter 6, our method was \(5\times 10^4\) times faster.
更多查看译文
关键词
inverse qsar/qspr,acyclic chemical compounds,skeleton trees
AI 理解论文
溯源树
样例
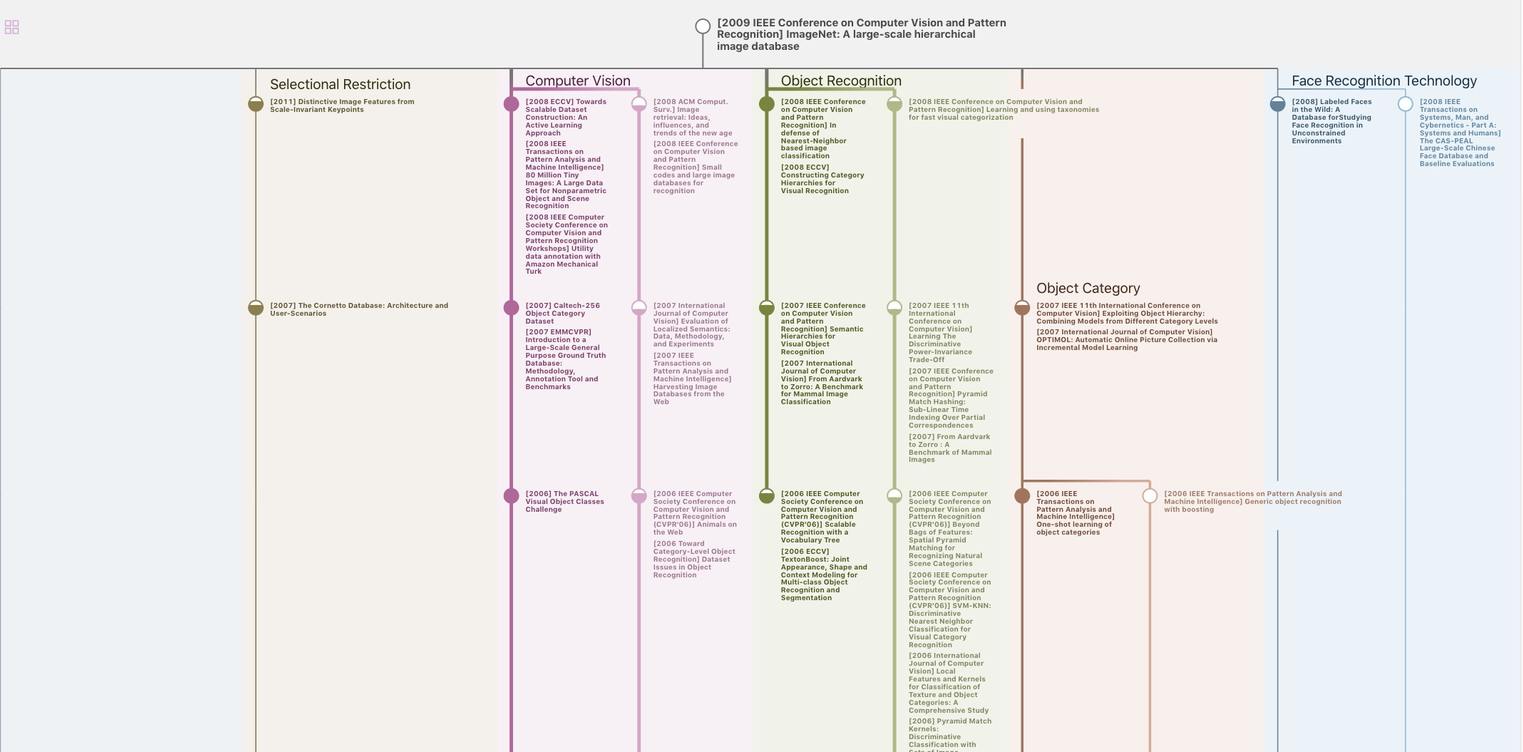
生成溯源树,研究论文发展脉络
Chat Paper
正在生成论文摘要