Predicting Student Flight Performance with Multimodal Features.
SBP-BRiMS(2020)
摘要
This paper investigates the problem of predicting student flight performance in a training simulation from multimodal features, including flight controls, visual attention, and knowledge acquisition tests. This is a key component of developing next generation training simulations that can optimize the usage of student training time. Two types of supervised machine learning classifiers (random forest and support vector machines) were trained to predict the performance of twenty-three students performing simple flight tasks in virtual reality. Our experiments reveal the following: 1) features derived from gaze tracking and knowledge acquisition tests can serve as an adequate substitute for flight control features; 2) data from the initial portion of the flight task is sufficient to predict the final outcome; 3) our classifiers perform slightly better at predicting student failure than success. These results indicate the feasibility of using machine learning for early prediction of student failures during flight training.
更多查看译文
关键词
Intelligent training simulations, Supervised learning, Gaze tracking, Multimodal features, Pilot training
AI 理解论文
溯源树
样例
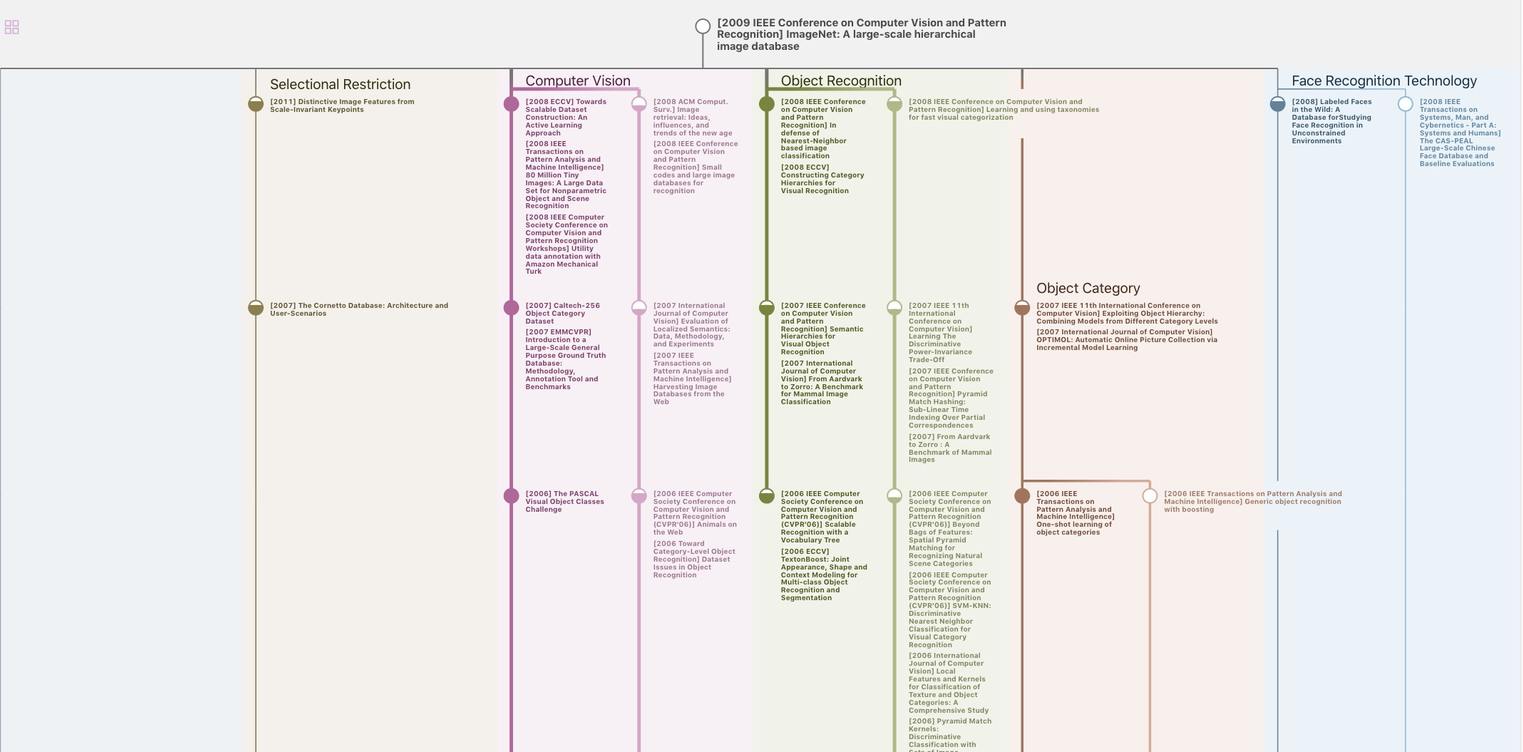
生成溯源树,研究论文发展脉络
Chat Paper
正在生成论文摘要