ARET - Aggregated Residual Extended Time-Delay Neural Networks for Speaker Verification.
INTERSPEECH(2020)
摘要
The time-delay neural network (TDNN) is widely used in speaker verification to extract long-term temporal features of speakers. Although common TDNN approaches well capture time-sequential information, they lack the delicate transformations needed for deep representation. To solve this problem, we propose two TDNN architectures. RET integrates short-cut connections into conventional time-delay blocks, and ARET adopts a split-transform-merge strategy to extract more discriminative representation. Experiments on VoxCeleb datasets without augmentation indicate that ARET realizes satisfactory performance on the VoxCeleb1 test set, VoxCeleb1-E, and VoxCeleb1-H, with 1.389%, 1.520%, and 2.614% equal error rate (EER), respectively. Compared to state-of-the-art results on these test sets, RET achieves a 23% similar to 43% relative reduction in EER, and ARET reaches 32% similar to 45%.
更多查看译文
关键词
residual transformations, aggregated transformations, time-delay neural networks, speaker verification
AI 理解论文
溯源树
样例
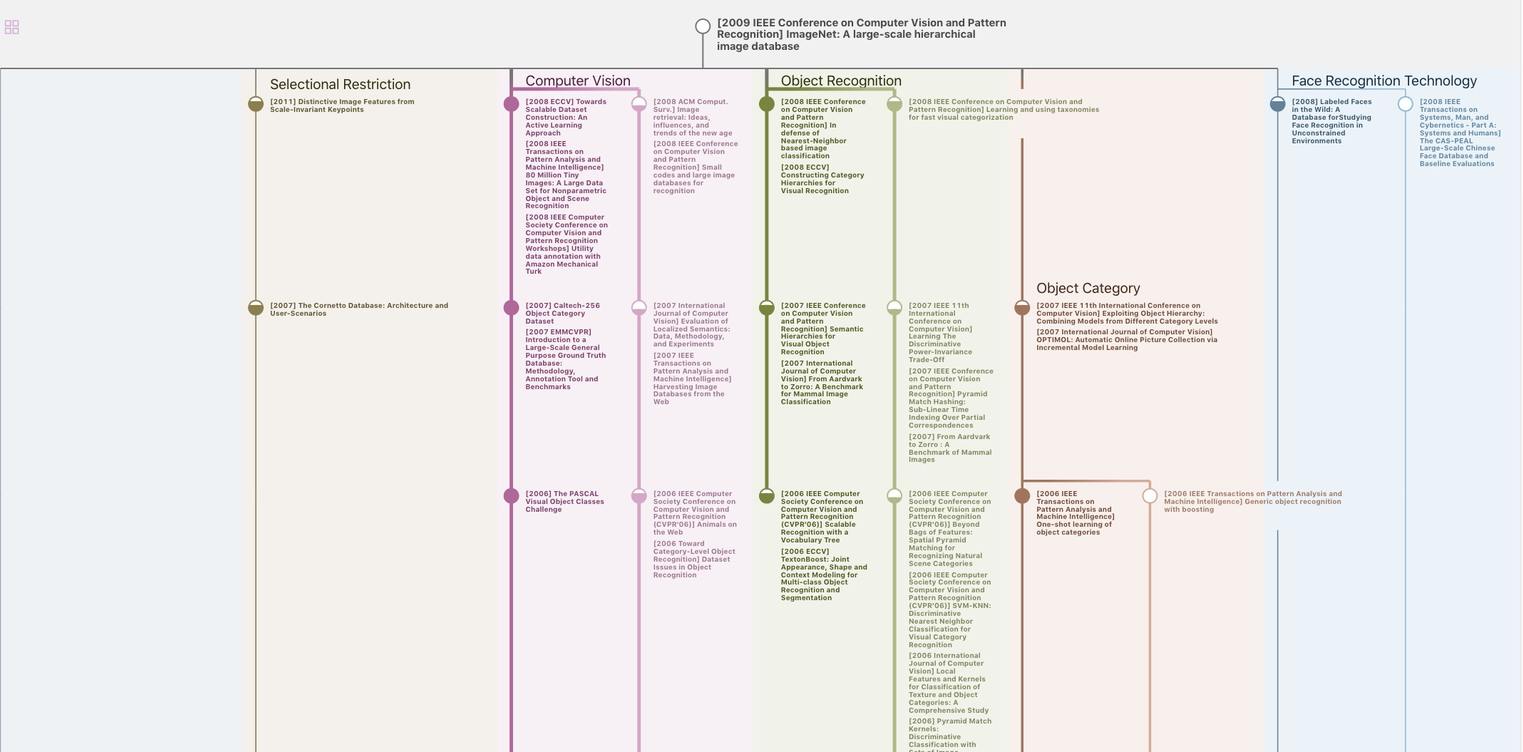
生成溯源树,研究论文发展脉络
Chat Paper
正在生成论文摘要