SpecSwap - A Simple Data Augmentation Method for End-to-End Speech Recognition.
INTERSPEECH(2020)
摘要
Recently, End-to-End (E2E) models have achieved state-of-the-art performance for automatic speech recognition (ASR). Within these large and deep models, overfitting remains an important problem that heavily influences the model performance. One solution to deal with the overfitting problem is to increase the quantity and variety of the training data with the help of data augmentation. In this paper, we present SpecSwap, a simple data augmentation scheme for automatic speech recognition that acts directly on the spectrogram of input utterances. The augmentation policy consists of swapping blocks of frequency channels and swapping blocks of time steps. We apply SpecSwap on Transformer-based networks for end-to-end speech recognition task. Our experiments on Aishell-1 show state-of-the-art performance for E2E models that are trained solely on the speech training data. Further, by increasing the depth of model, the Transformers trained with augmentations can outperform certain hybrid systems, even without the aid of a language model.
更多查看译文
关键词
end-to-end speech recognition, data augmentation
AI 理解论文
溯源树
样例
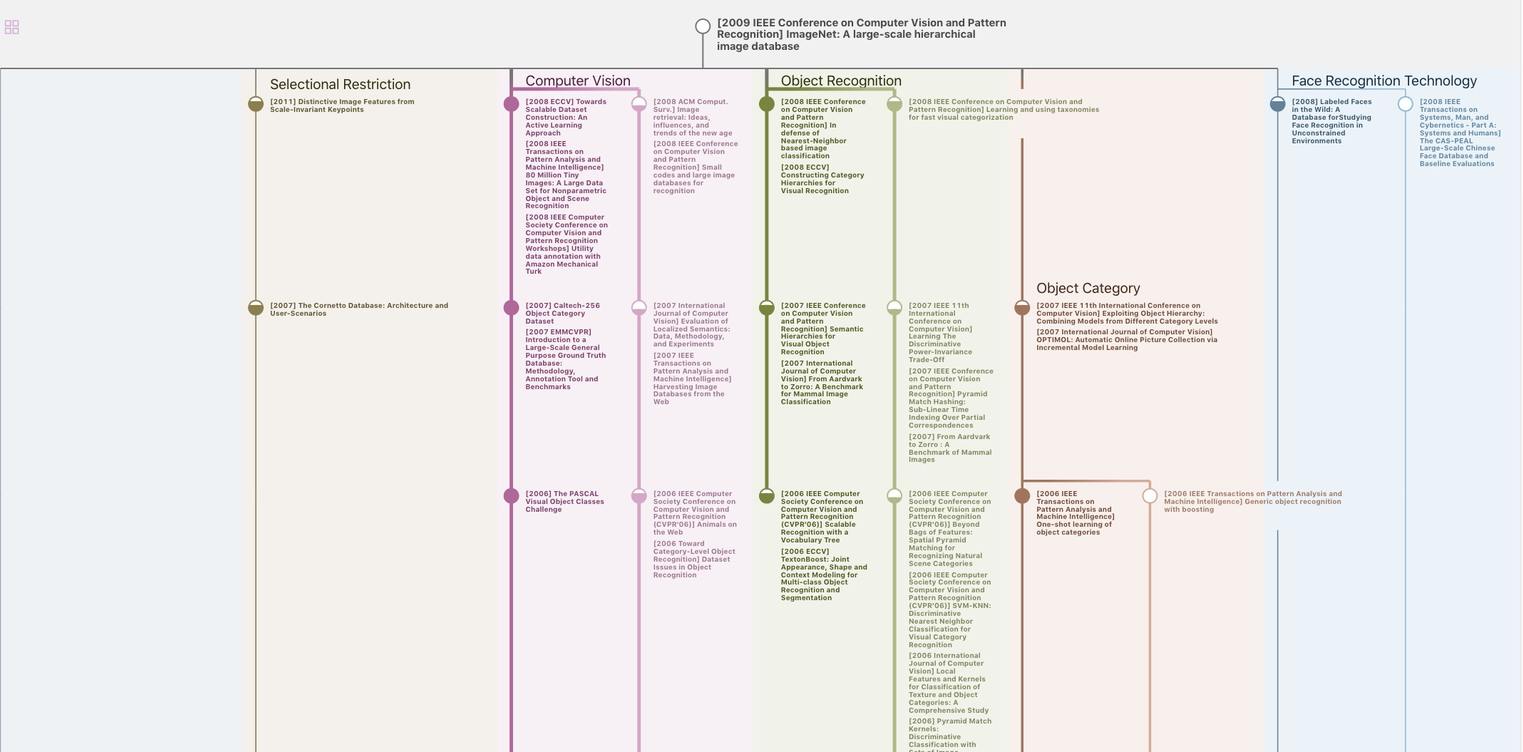
生成溯源树,研究论文发展脉络
Chat Paper
正在生成论文摘要