Building a Robust Word-Level Wakeword Verification Network
INTERSPEECH(2020)
摘要
Wakeword detection is responsible for switching on downstream systems in a voice-activated device. To prevent a response when the wakeword is detected by mistake, a secondary network is often utilized to verify the detected wakeword. Published verification approaches are formulated based on Automatic Speech Recognition (ASR) biased towards the wakeword. This approach has several drawbacks, including high model complexity and the necessity of large vocabulary training data. To address these shortcomings, we propose to use a large receptive field (LRF) word-level wakeword model, and in particular, a convolutional-recurrent-attention (CRA) network. CRA networks use a strided small receptive field convolutional front-end followed by fixed time-step recurrent layers optimized to model the temporal phonetic dependencies within the wakeword. We experimentally show that this type of modeling helps the system to be robust to errors in the location of the wakeword as estimated by the detection network. The proposed CRA network significantly outperforms previous baselines, including an LRF whole-word convolutional network and a 2-stage DNN-HMM system. Additionally, we study the importance of pre- and post-wakeword context. Finally, the CRA network has significantly fewer model parameters and multiplies, which makes it suitable for real-world production applications.
更多查看译文
关键词
wakeword spotting,voice activated devices,keyword spotting,wakeword detection,convolutional network,convolutional recurrent network,attention network
AI 理解论文
溯源树
样例
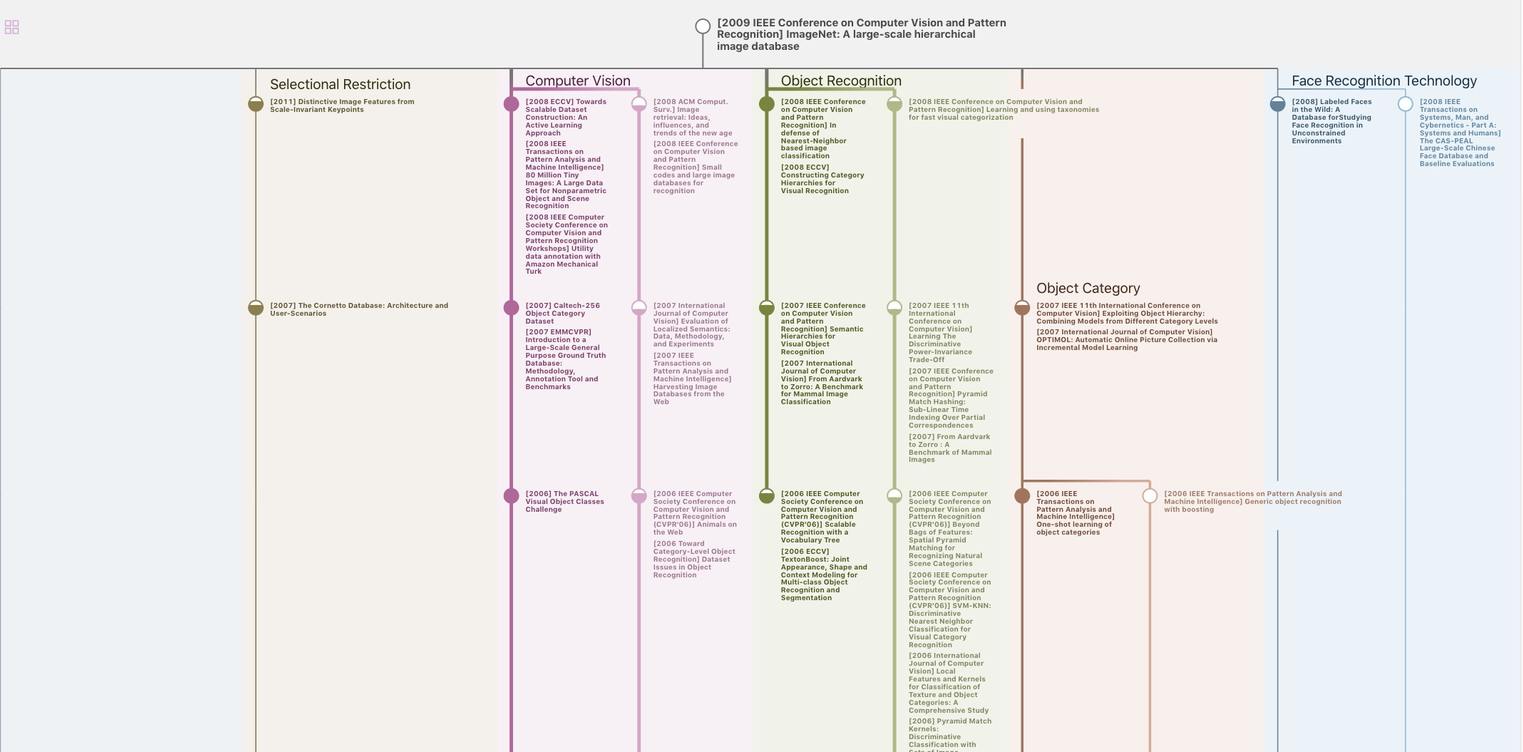
生成溯源树,研究论文发展脉络
Chat Paper
正在生成论文摘要