Active Scene Classification via Dynamically Learning Prototypical Views.
DDDAS(2020)
摘要
Scene classification is an important computer vision problem with applications to a wide range of domains including remote sensing, robotics, autonomous driving, defense, and surveillance. However, many approaches to scene classification make simplifying assumptions about the data, and many algorithms for scene classification are ill-suited for real-world use cases. Specifically, scene classification algorithms generally assume that the input data consists of single views that are extremely representative of a limited set of known scene categories. In real-world applications, such perfect data is rarely encountered. In this paper, we propose an approach for active scene classification where an agent must assign a label to the scene with high confidence while minimizing the number of sensor adjustments, and the agent is also embedded with the capability to dynamically update its underlying machine learning models. Specifically, we employ the Dynamic Data-Driven Applications Systems paradigm: our machine learning model drives the sensor manipulation, and the data captured by the manipulated sensor is used to update the machine learning model in a feedback control loop. Our approach is based on learning to identify prototypical views of scenes in a streaming setting.
更多查看译文
关键词
classification,views,dynamically,active,learning
AI 理解论文
溯源树
样例
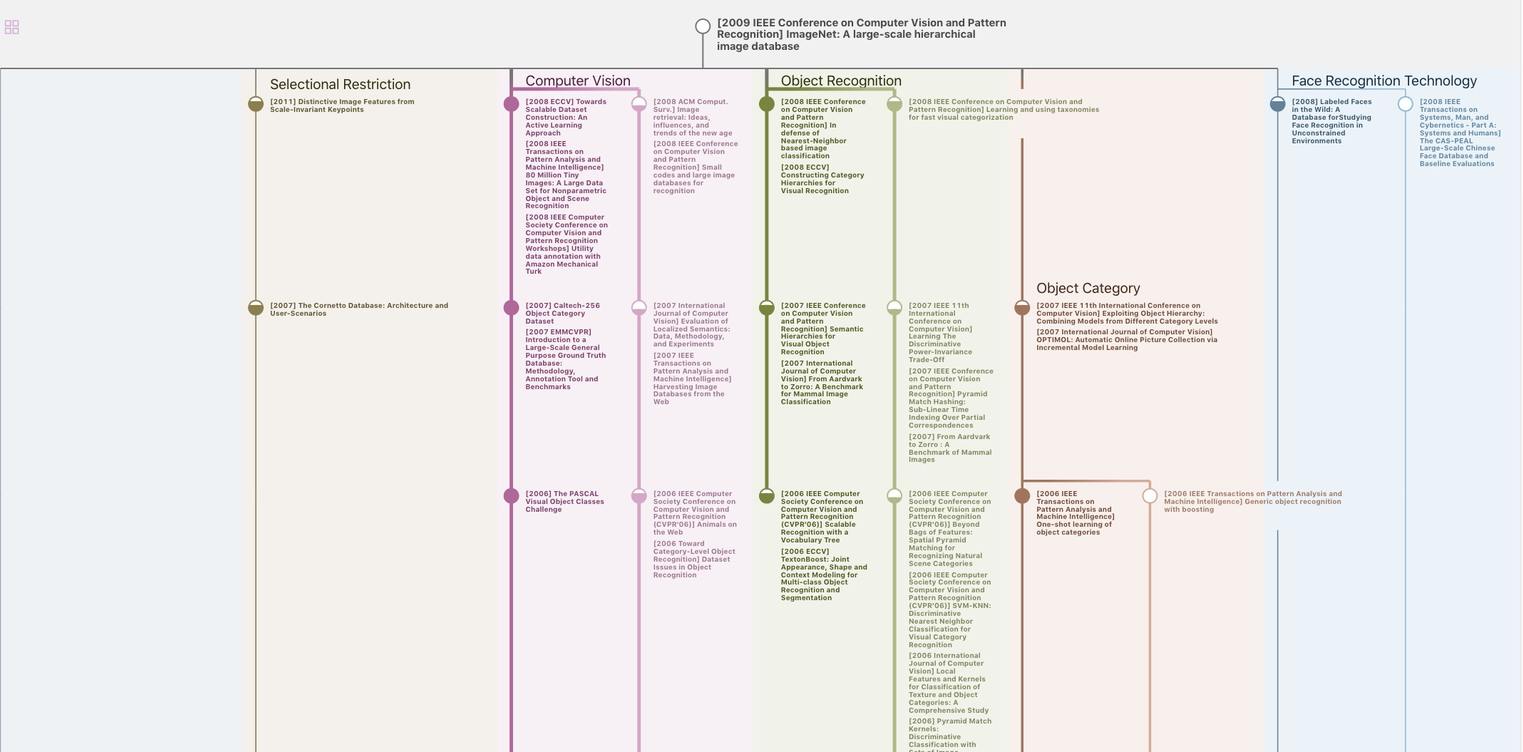
生成溯源树,研究论文发展脉络
Chat Paper
正在生成论文摘要