Research on Data Quality Control of Crowdsourcing Annotation: A Survey
2020 IEEE Intl Conf on Dependable, Autonomic and Secure Computing, Intl Conf on Pervasive Intelligence and Computing, Intl Conf on Cloud and Big Data Computing, Intl Conf on Cyber Science and Technology Congress (DASC/PiCom/CBDCom/CyberSciTech)(2020)
摘要
It is well known that many intelligent and computer-hard tasks cannot be effectively addressed by existing machine-based approaches, so that it is nature to think of utilizing the intelligence of human being. With the popularization of crowdsourcing concepts as well as the development of crowdsourcing platforms, as a new way of human intelligence to participate in machine computing, crowdsourcing annotation helps more and more supervised-learning-based approaches easily obtain enormous labeled data with relatively low cost. However, because of the diversity of the crowd employed by crowdsourcing platforms, how to control qualities of labels coming from the crowd plays a key role in crowdsourcing annotation. In this survey, we first present basic concepts and definitions of crowdsourcing annotation. Then, we review existing ground truth inference algorithms and learning models. After that, the advantages and distinctions among these algorithms and learning models as well as the levels of study progresses will be reported. And finally, we summarize realworld datasets widely utilized in the field of crowdsourcing annotation as well as available open source tools.
更多查看译文
关键词
label,truth inference,learning model,crowdsourcing
AI 理解论文
溯源树
样例
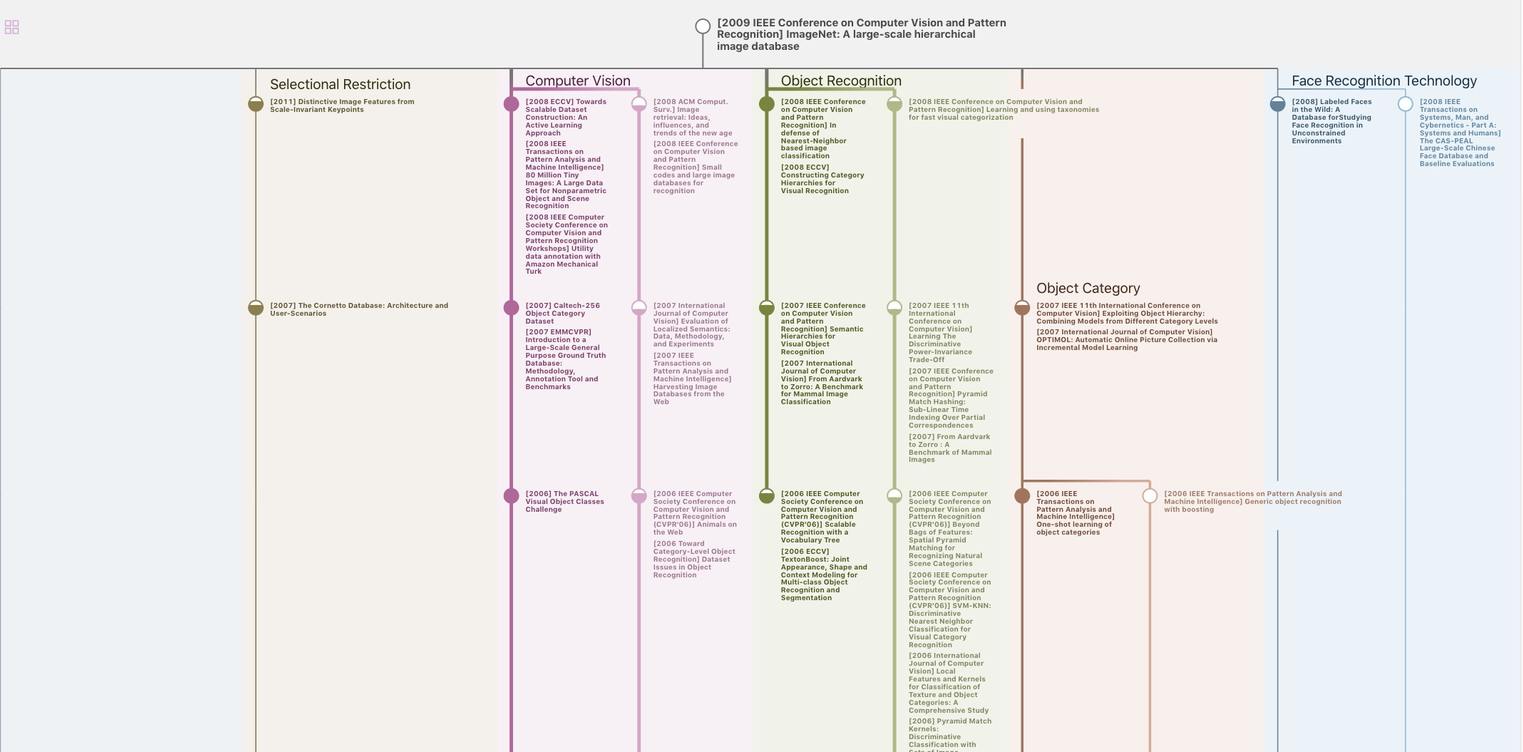
生成溯源树,研究论文发展脉络
Chat Paper
正在生成论文摘要