Comparison of Machine Learning Methods in Classification of Affective Disorders
2020 43rd International Convention on Information, Communication and Electronic Technology (MIPRO)(2020)
摘要
Depression belongs to a group of psychiatric disorders called affective disorders. In medical practice, patients are diagnosed according to the criteria in standardized diagnostic manuals. The criteria for diagnosing such disorders focus on the symptoms presented by the patient as well as on disqualifying other potential causes of the symptoms. Electroencephalography (EEG) is a noninvasive brain imaging technique that measures the electrical activity of the brain across different sites on the surface of the scalp. In this paper, 15 EEGs of depression patients and 15 EEGs of healthy control subjects are observed. The depressed and healthy subjects are paired according to age and gender to achieve a dataset that is balanced across classes, gender, and age of subjects. 475 different features are extracted from each EEG and used in the evaluation of different binary classification methods. The best F1-score of 0.7586 is achieved with the K-nearest neighbor algorithm. Sequential feature selection is performed, and sequentially selected features are used to evaluate the former binary classification methods. The best F1-score of 0.8750 is achieved with the K-nearest neighbor algorithm. Classification results are compared across different methods, as well as before and after excluding features that were not deemed significant by the sequential selection algorithm.
更多查看译文
关键词
electroencephalography,affective disorders,depression,feature selection,binary classification
AI 理解论文
溯源树
样例
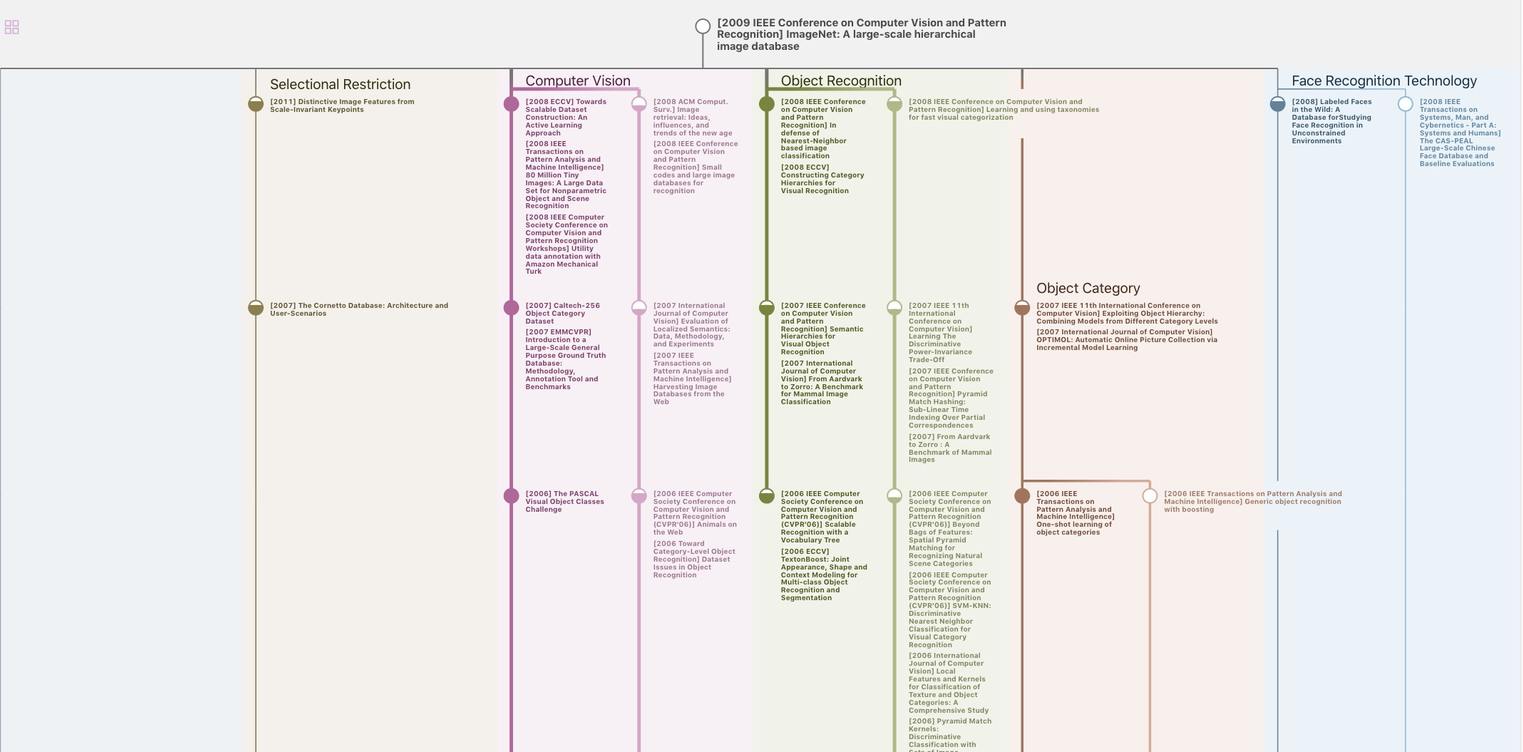
生成溯源树,研究论文发展脉络
Chat Paper
正在生成论文摘要