Label-Driven Brain Deformable Registration Using Structural Similarity and Nonoverlap Constraints.
MLMI@MICCAI(2020)
摘要
Accurate deformable image registration is important for brain analysis. However, there are two challenges in deformation registration of brain magnetic resonance (MR) images. First, the global cerebrospinal fluid (CSF) regions are rarely aligned since most of them are located in narrow regions outside of gray matter (GM) tissue. Second, the small complex morphological structures in tissues are rarely aligned since dense deformation fields are too blurred. In this work, we use a weakly supervised registration scheme, which is driven by global segmentation labels and local segmentation labels via two special loss functions. Specifically, multiscale double Dice similarity is used to maximize the overlap of the same labels and also minimize the overlap of regions with different labels. The structural similarity loss function is further used to enhance registration performance of small structures, thus enhancing the whole image registration accuracy. Experimental results on inter-subject registration of T1-weightedMRbrain images from the OASIS-1 dataset show that the proposed scheme achieves higher accuracy on CSF, GM and white matter (WM) compared with the baseline learning model.
更多查看译文
关键词
MR brain images, Deformable registration, Label-driven learning, Structural similarity
AI 理解论文
溯源树
样例
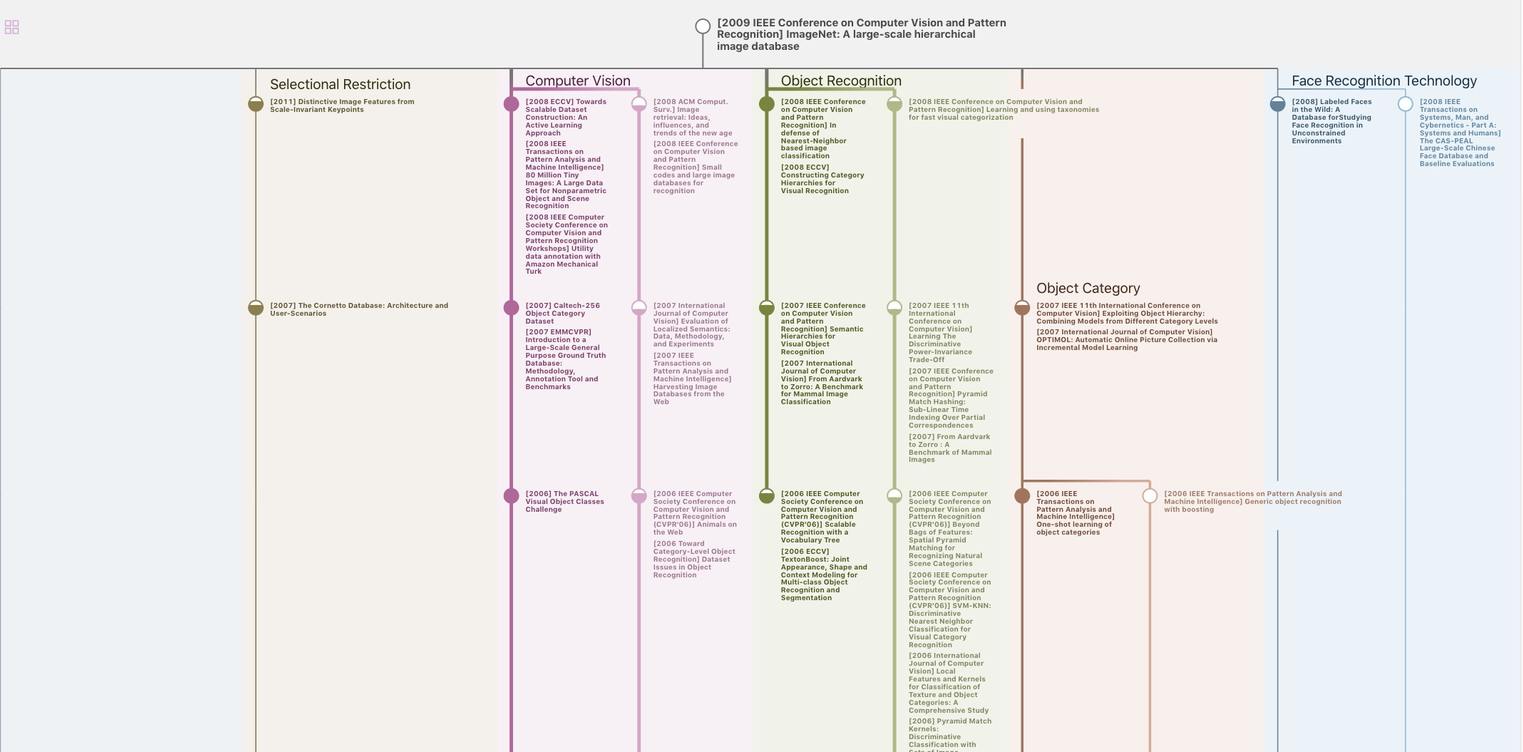
生成溯源树,研究论文发展脉络
Chat Paper
正在生成论文摘要