Analysing Word Representation from the Input and Output Embeddings in Neural Network Language Models.
CoNLL(2020)
摘要
Researchers have recently demonstrated that tying the neural weights between the input look-up table and the output classification layer can improve training and lower perplexity on sequence learning tasks such as language modelling. Such a procedure is possible due to the design of the softmax classification layer, which previous work has shown to comprise a viable set of semantic representations for the model vocabulary, and these these output embeddings are known to perform well on word similarity benchmarks. In this paper, we make meaningful comparisons between the input and output embeddings and other SOTA distributional models to gain a better understanding of the types of information they represent. We also construct a new set of word embeddings using the output embeddings to create locally-optimal approximations for the intermediate representations from the language model. These locally-optimal embeddings demonstrate excellent performance across all our evaluations.
更多查看译文
关键词
neural network language models,word representation,output embeddings,neural network
AI 理解论文
溯源树
样例
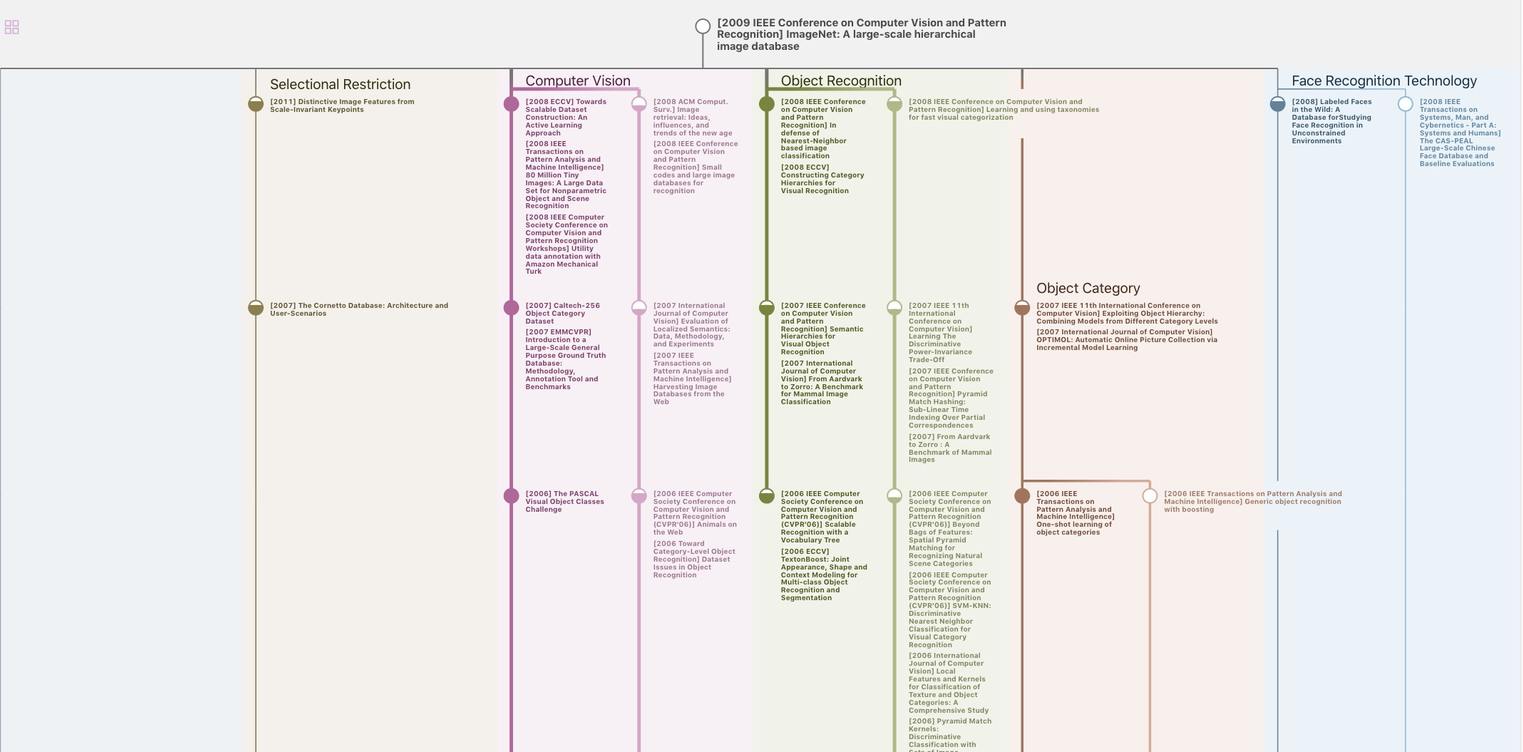
生成溯源树,研究论文发展脉络
Chat Paper
正在生成论文摘要