Using CNNs to Optimize Numerical Simulations in Geotechnical Engineering.
ANNPR(2020)
摘要
Deep excavations are today mainly designed by manually optimising the wall’s geometry, stiffness and strut or anchor layout. In order to better automate this process for sustained excavations, we are exploring the possibility of approximating key values using a machine learning (ML) model instead of calculating them with time-consuming numerical simulations. After demonstrating in our previous work that this approach works for simple use cases, we show in this paper that this method can be enhanced to adapt to complex real-world supported excavations. We have improved our ML model compared to our previous work by using a convolutional neural network (CNN) model, coding the excavation configuration as a set of layers of fixed height containing the soil parameters as well as the geometry of the walls and struts. The system is trained and evaluated on a set of synthetically generated situations using numerical simulation software. To validate this approach, we also compare our results to a set of 15 real-world situations in a t-SNE. Using our improved CNN model we could show that applying machine learning to predict the output of numerical simulation in the domain of geotechnical engineering not only works in simple cases but also in more complex, real-world situations.
更多查看译文
关键词
optimize numerical simulations,cnns,engineering
AI 理解论文
溯源树
样例
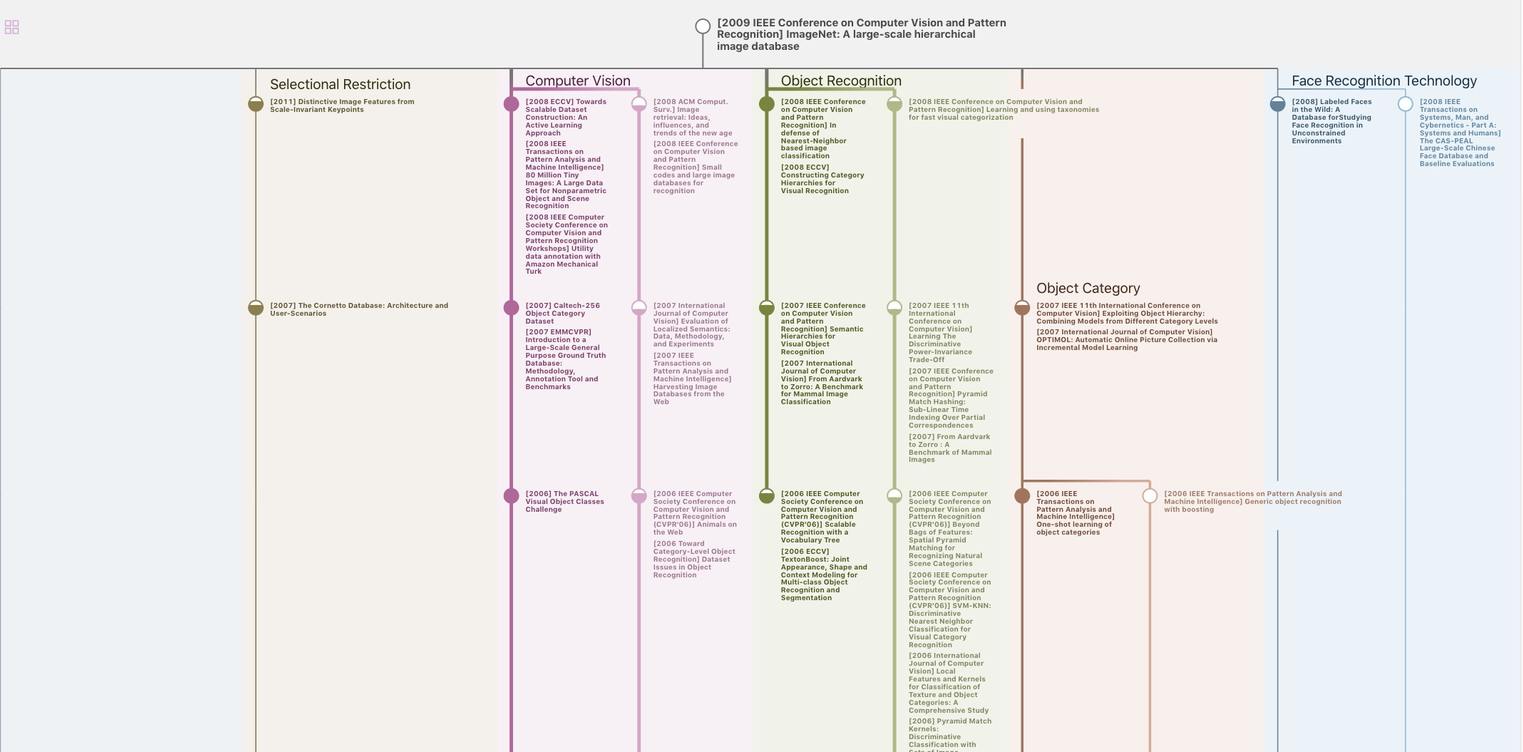
生成溯源树,研究论文发展脉络
Chat Paper
正在生成论文摘要