Deep Learning Methods for Image Guidance in Radiation Therapy.
ANNPR(2020)
摘要
Image guidance became one of the most important key technologies in radiation therapy in the last two decades. Nowadays medical images play a key role in virtually every aspect of the common treatment workflows. Advances in imaging hardware and algorithmic processing are enablers for substantial treatment quality improvements like online adaptation of the treatment, accounting for anatomical changes of the day, or intra-fraction motion monitoring and organ position verification during treatment. Going through this rapid development, an important observation is that further improvements of various methods heavily rely on model knowledge. In a classical sense such model knowledge is for example provided by mathematically formulated physical assumptions to ill-posed problems or by expert systems and heuristics. Recently, it became evident that in various applications such classical approaches get outperformed by data driven machine learning methods. Especially worth to mention is that this not only holds true in terms of precision and computational performance but also in terms of complexity reduction and maintainability. In this paper we provide an overview about the different stages in the X-ray based imaging pipeline in radiation therapy where machine learning based algorithms show promising results or are already applied in clinical routine.
更多查看译文
关键词
Radiation therapy, X-ray imaging, Cone Beam Computed Tomography (CBCT), Image Guided Radiation Therapy (IGRT), During treatment motion monitoring, Tissue tracking, Automatic segmentation, Artificial intelligence, Deep learning
AI 理解论文
溯源树
样例
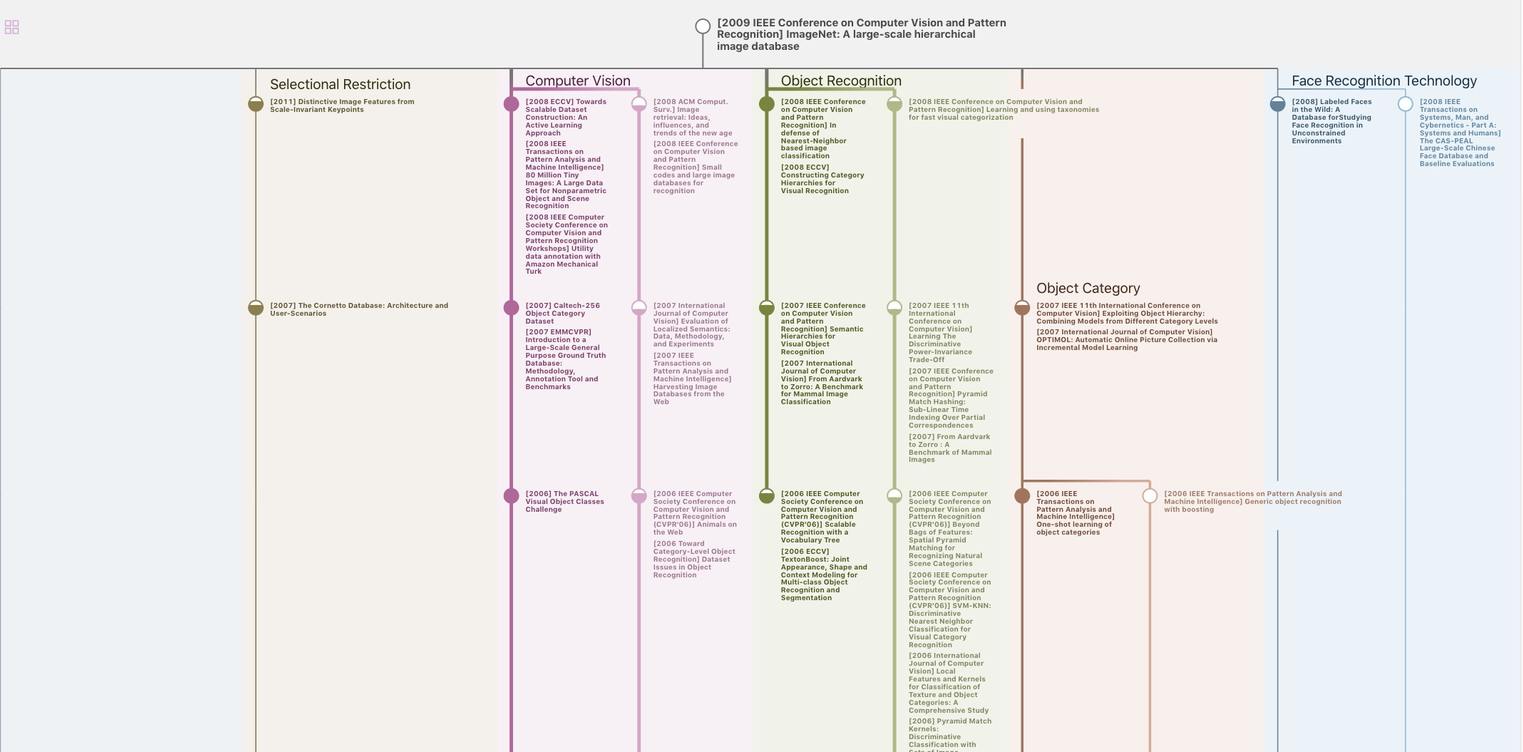
生成溯源树,研究论文发展脉络
Chat Paper
正在生成论文摘要