InfoSalGAIL - Visual Attention-empowered Imitation Learning of Pedestrian Behavior in Critical Traffic Scenarios.
IJCCI(2020)
摘要
The imitation learning of complex pedestrian behavior based on visual input is a challenge due to the underlying large state space and variations. In this paper, we present a novel visual attention-based imitation learning framework, named InfoSalGAIL, for end-to-end imitation learning of (safe, unsafe) pedestrian navigation policies through visual expert demonstrations empowered by eye fixation sequence and augmented reward function. This work shows the relation in latent space between the policy estimated trajectories and visual-attention map. Moreover, the conducted experiments revealed that InfoSalGAIL can significantly outperform the state-of-the-art baseline InfoGAIL. In fact, its visual attention-empowered imitation learning tends to much better generalize the overall policy of pedestrian behavior leveraging apprenticeship learning to generate more human-like pedestrian trajectories in virtual traffic scenes with the open source driving simulator OpenDS. InfoSalGAIL can be utilized in the process of generating and validating critical scenarios for adaptive driving assistance systems.
更多查看译文
关键词
Visual Attention-empowered Imitation Learning, End-to-End Human-like Data-driven Simulation, Critical Scenario Generation
AI 理解论文
溯源树
样例
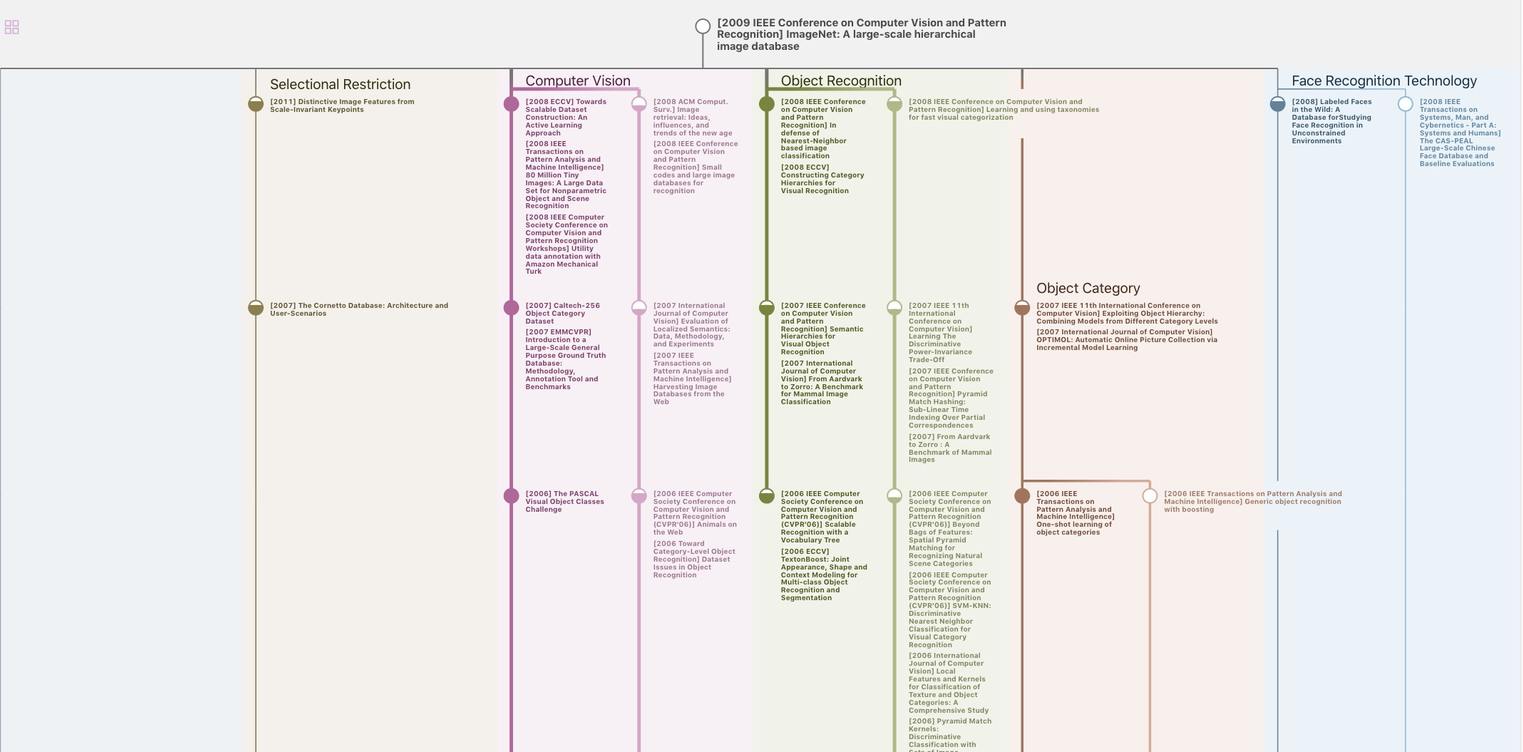
生成溯源树,研究论文发展脉络
Chat Paper
正在生成论文摘要