Object Synthesis By Learning Part Geometry With Surface And Volumetric Representations
COMPUTER-AIDED DESIGN(2021)
摘要
We propose a conditional generative model, named Part Geometry Network (PG-Net), which synthesizes realistic objects and can be used as a robust feature descriptor for object reconstruction and classification. Surface and volumetric representations of objects have complementary properties of three-dimensional objects. Combining these modalities is more informative than using one modality alone. Therefore, PG-Net utilizes complementary properties of surface and volumetric representations by estimating curvature, surface area, and occupancy in voxel grids of objects with a single decoder as a multi-task learning. Objects are combinations of multiple parts, and therefore part geometry (PG) is essential to synthesize each part of the objects. PG-Net employs a part identifier to learn the part geometry. Additionally, we augmented a dataset by interpolating individual functional parts such as wings of an airplane, which helps learning part geometry and finding local/global minima of PG-Net. To demonstrate the capability of learning object representations of PG-Net, we performed object reconstruction and classification tasks on two standard large-scale datasets. PG-Net outperformed the state-of-the-art methods in object synthesis, classification, and reconstruction in a large margin. (C) 2020 Elsevier Ltd. All rights reserved.
更多查看译文
关键词
Deep learning, Conditional generative model, Multi-task learning, Object synthesis
AI 理解论文
溯源树
样例
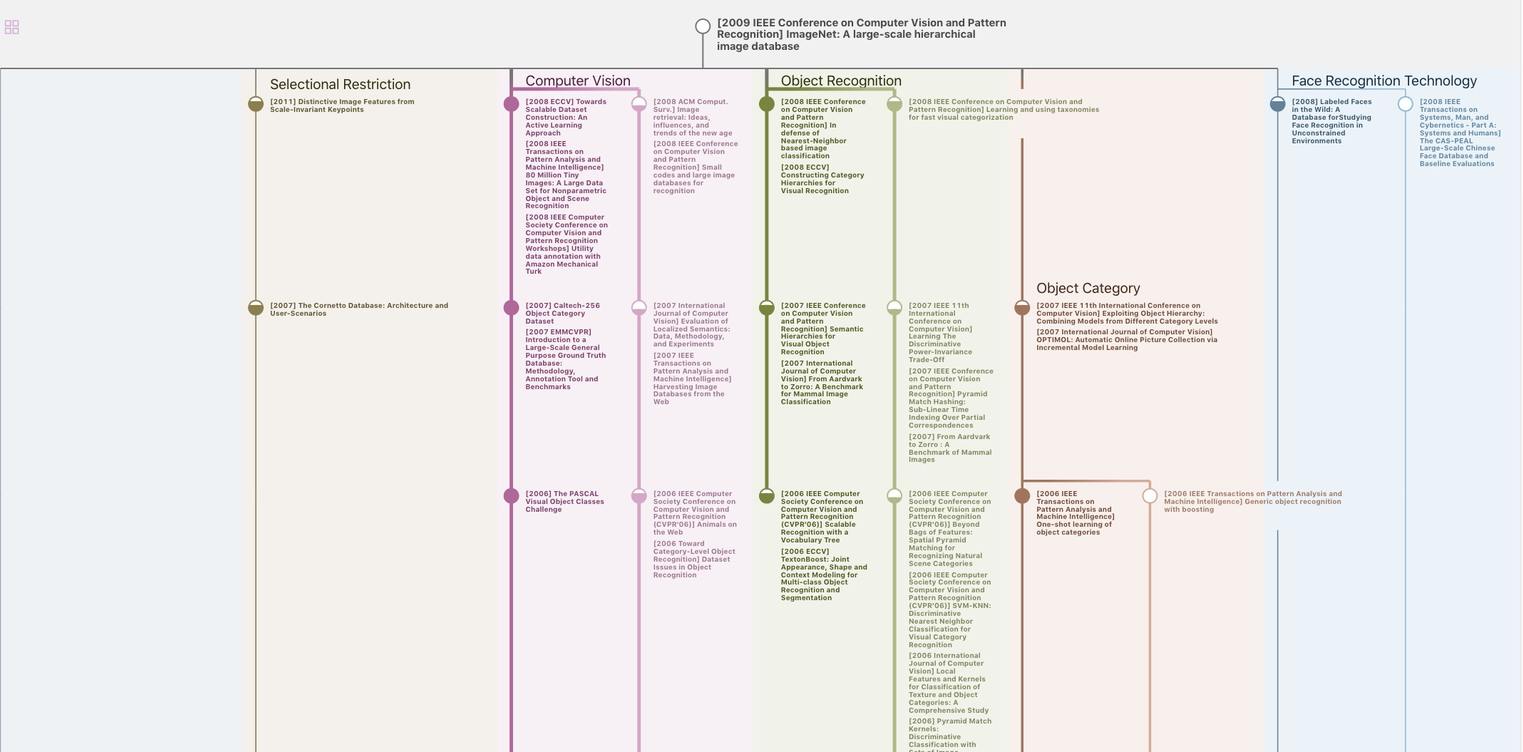
生成溯源树,研究论文发展脉络
Chat Paper
正在生成论文摘要