Unsupervised Anomaly Detection In Peripheral Venous Pressure Signals With Hidden Markov Models
BIOMEDICAL SIGNAL PROCESSING AND CONTROL(2020)
摘要
This paper proposes an automatic anomaly detection and removal algorithm for peripheral venous pressure (PVP) signals, which can be used to predict intravascular volume loss in humans. PVP signal collection is a minimally invasive procedure that can be performed by using a standard peripheral intravenous (PIV) catheter and a commercial pressure-monitoring transducer. PVP signals are highly susceptible to motion and noise artifacts such as patient movements or unintended manipulation of PIV lines. Anomalies in PVP signals can corrupt useful information and seriously affect the integrity of PVP signal analysis. We propose to detect and remove such anomalies by exploiting the properties of PVP signals. Specifically, a dynamic linear model (DLM) with a Kalman filter is used to track and predict the time-domain evolution of PVP signals. The prediction residuals of the Kalman filter are then modeled with a hidden Markov model (HMM), with the normal and anomalous status of the signal modeled by using binary states of a hidden Markov chain. The HMM parameters along with the hidden states are iteratively estimated by using an unsupervised learning algorithm with a modified Baum-Welch method. The anomaly detection algorithm is applied to clinical data from a cohort of 24 pediatric patients with hypertrophic pyloric stenosis. Experimental results demonstrate that the proposed unsupervised anomaly detection algorithm can efficiently remove anomalies in PVP signals without the need of a training phase. The algorithm can also be applied to other time series signals, such as Electrocardiography (ECG) and Photoplethysmogram (PPG) signals.
更多查看译文
关键词
Peripheral venous pressure (PVP), Anomaly detection, Motion and noise artifacts (MNA), Hidden Markov model (HMM), State Space Model (SSM), Dynamic linear model (DLM)
AI 理解论文
溯源树
样例
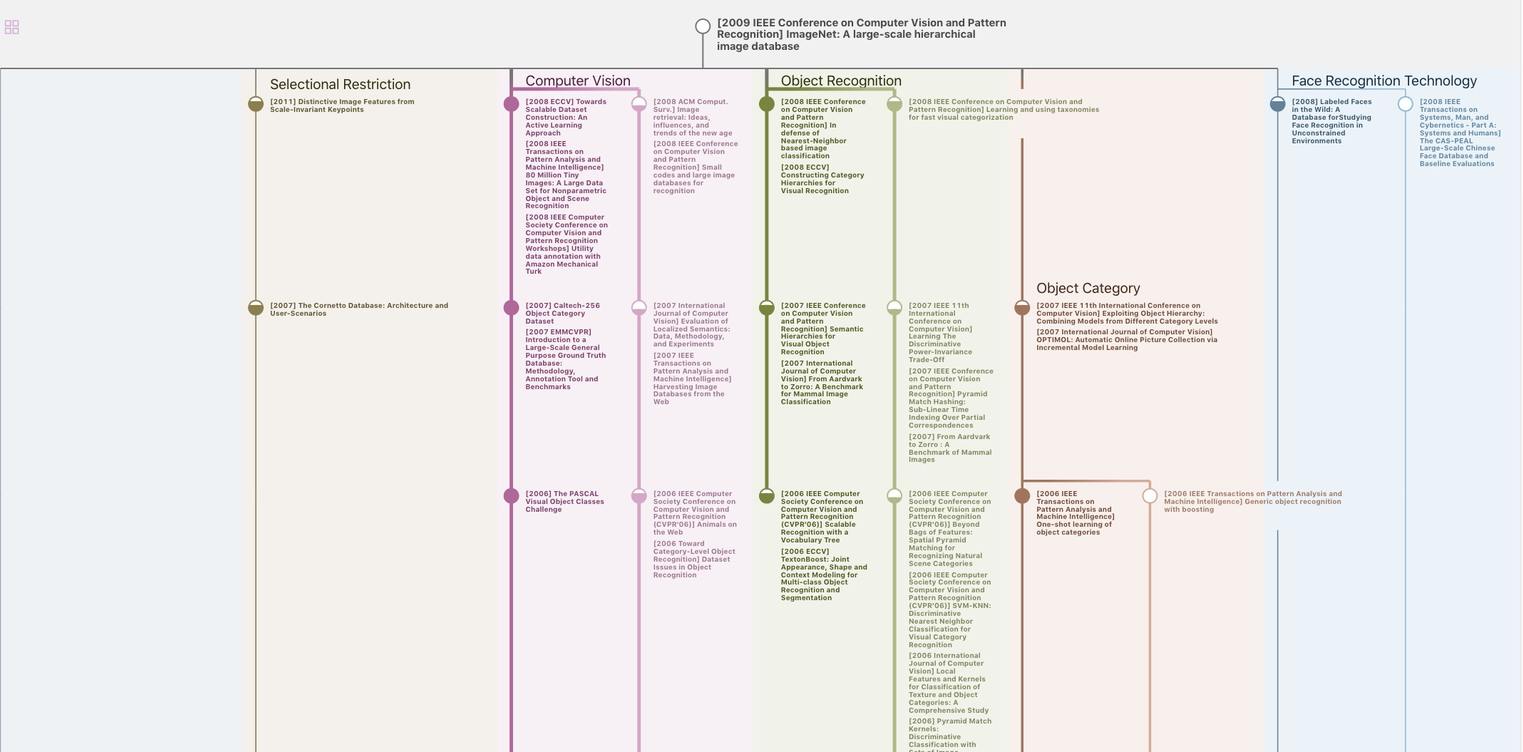
生成溯源树,研究论文发展脉络
Chat Paper
正在生成论文摘要