DeepDist: A Deep-Learning-Based IoV Framework for Real-Time Objects and Distance Violation Detection
IEEE Internet of Things Magazine(2020)
摘要
Crowd management systems play a vital role in today's smart cities and rely on several Internet of Things (IoT) solutions to build prevention mechanisms for widespread viral diseases such as Coronavirus 2019 (COVID-19). In this article, we propose a framework to aid in preventing widespread viral diseases. The proposed framework consists of a physical distancing notification system by leveraging some existing futuristic technologies, including deep learning and the Internet of Vehicles. Each vehicle is equipped with a switching camera system through thermal and vision imaging. Afterward, using the Faster R-CNN algorithm, we measure and detect physical distancing violation between objects of the same class. We evaluate the performance of our proposed architecture with vehicle-to-infrastructure communication. The obtained results show the applicability and efficiency of our proposal in providing timely notification of social distancing violations.
更多查看译文
关键词
DeepDist,IoV,real-time object detection,distance violation detection,crowd management systems,smart cities,Internet of Things,widespread viral diseases,coronavirus 2019,physical distancing notification system,deep learning,switching camera system,thermal vision imaging,Faster R-CNN algorithm,physical distancing violation,vehicle-to-infrastructure communication,social distancing violations,Internet of Vehicle
AI 理解论文
溯源树
样例
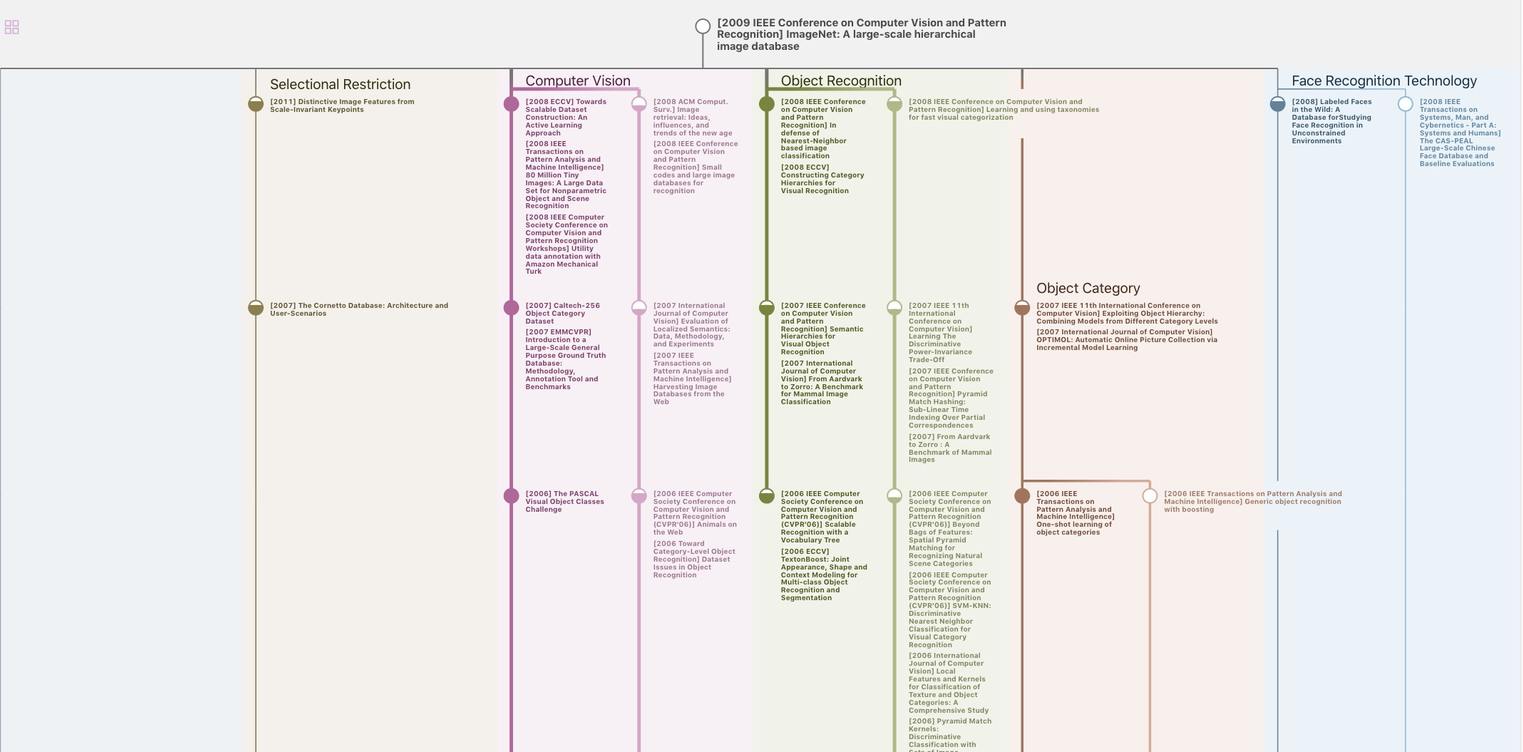
生成溯源树,研究论文发展脉络
Chat Paper
正在生成论文摘要