Language Model Pre-Training Method In Machine Translation Based On Named Entity Recognition
INTERNATIONAL JOURNAL ON ARTIFICIAL INTELLIGENCE TOOLS(2020)
摘要
Neural Machine Translation (NMT) model has become the mainstream technology in machine translation. The supervised neural machine translation model trains with abundant of sentence-level parallel corpora. But for low-resources language or dialect with no such corpus available, it is difficult to achieve good performance. Researchers began to focus on unsupervised neural machine translation (UNMT) that monolingual corpus as training data. UNMT need to construct the language model (LM) which learns semantic information from the monolingual corpus. This paper focuses on the pre-training of LM in unsupervised machine translation and proposes a pre-training method, NER-MLM (named entity recognition masked language model). Through performing NER, the proposed method can obtain better semantic information and language model parameters with better training results. In the unsupervised machine translation task, the BLEU scores on the WMT'16 English-French, English-German, data sets are 35.30, 27.30 respectively. To the best of our knowledge, this is the highest results in the field of UNMT reported so far.
更多查看译文
关键词
Unsupervised machine translation, language model, named entity recognition
AI 理解论文
溯源树
样例
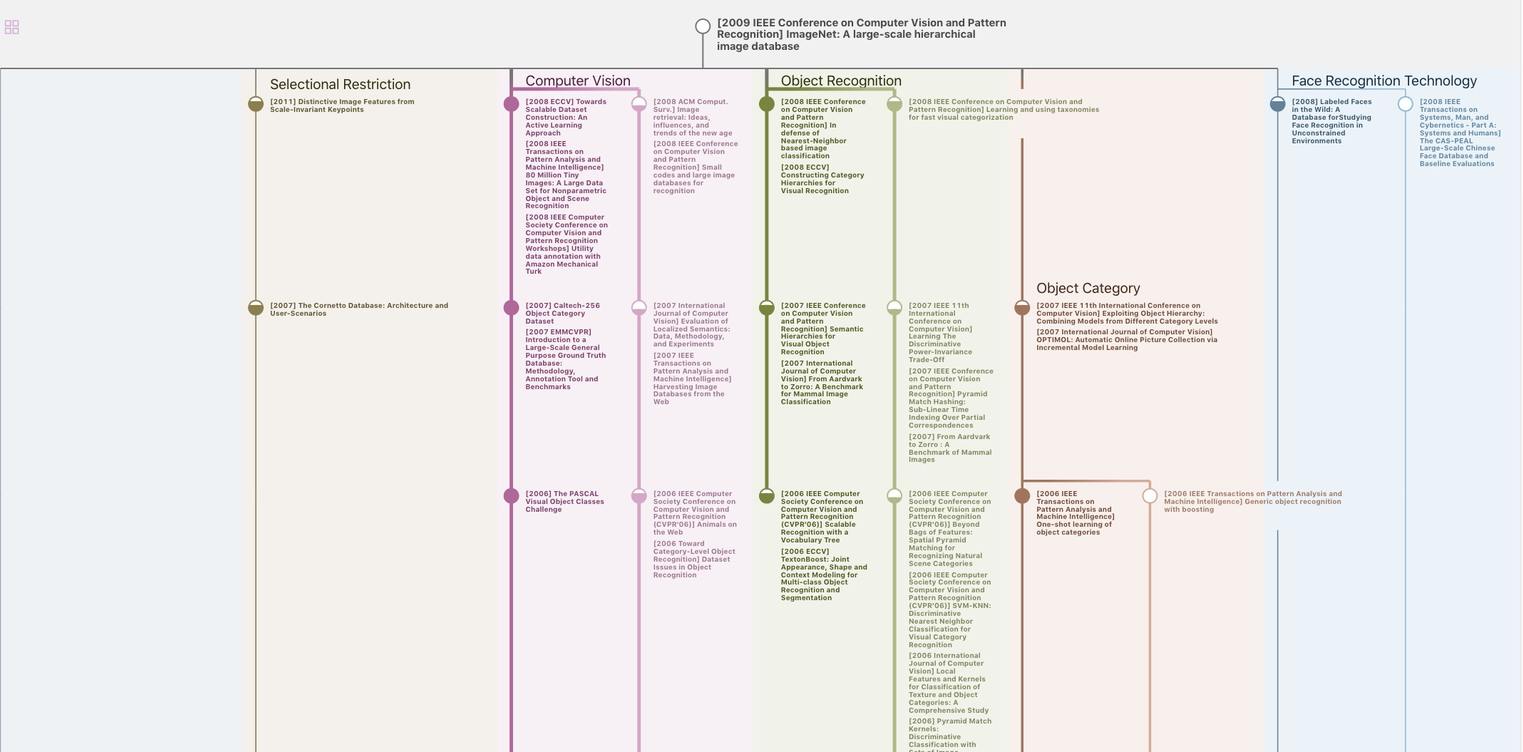
生成溯源树,研究论文发展脉络
Chat Paper
正在生成论文摘要