A systematic study of reward for reinforcement learning based continuous integration testing
Journal of Systems and Software(2020)
摘要
Continuous integration(CI) testing is characterized by continually changing test cases, limited execution time, and fast feedback, where the classical test prioritization approaches are no longer suitable. Based on the essence of continuous decision mechanism, reinforcement learning(RL) is suggested for prioritizing test cases in CI testing, in which the reward plays a crucial role. In this paper, we conducted a systematic study of the reward function and reward strategy in CI testing. In terms of reward function, the whole historical execution information of test cases is used with the consideration of the failure times and failure distribution. Further considering the validity of historical information, partial historical information is used by proposing a time-window based approach. In terms of reward strategy which means how to reward, three strategies are introduced, i.e., total reward, partial reward, and fuzzy reward. The empirical study is conducted on four industrial-level programs, and the results reveal that using the reward function with historical information improves the Recall by on average 13.21% when compared with existing TF(Test Case Failure) reward function, and the fuzzy reward strategy is more flexible and improve the NAPFD(Normalized Average Percentage of Faults Detected) by on average 3.43% when compared with the other two strategies.
更多查看译文
关键词
00-01,99-00
AI 理解论文
溯源树
样例
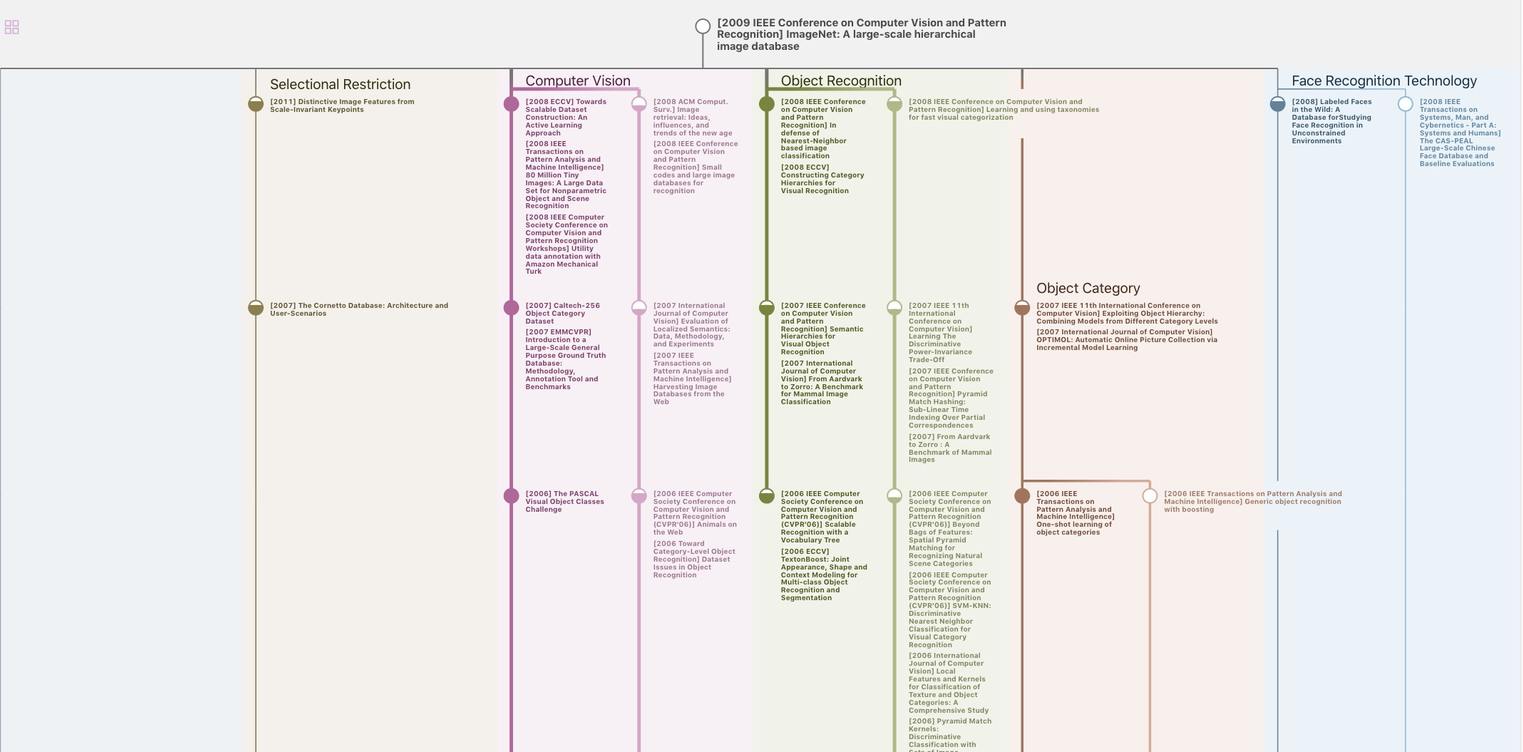
生成溯源树,研究论文发展脉络
Chat Paper
正在生成论文摘要