Resolving Hand-Object Occlusion For Mixed Reality With Joint Deep Learning And Model Optimization
COMPUTER ANIMATION AND VIRTUAL WORLDS(2020)
摘要
By overlaying virtual imagery onto the real world, mixed reality facilitates diverse applications and has drawn increasing attention. Enhancing physical in-hand objects with a virtual appearance is a key component for many applications that require users to interact with tools such as surgery simulations. However, due to complex hand articulations and severe hand-object occlusions, resolving occlusions in hand-object interactions is a challenging topic. Traditional tracking-based approaches are limited by strong ambiguities from occlusions and changing shapes, while reconstruction-based methods show a poor capability of handling dynamic scenes. In this article, we propose a novel real-time optimization system to resolve hand-object occlusions by spatially reconstructing the scene with estimated hand joints and masks. To acquire accurate results, we propose a joint learning process that shares information between two models and jointly estimates hand poses and semantic segmentation. To facilitate the joint learning system and improve its accuracy under occlusions, we propose an occlusion-aware RGB-D hand data set that mitigates the ambiguity through precise annotations and photorealistic appearance. Evaluations show more consistent overlays compared with literature, and a user study verifies a more realistic experience.
更多查看译文
关键词
deep learning, hand tracking, mixed reality, occlusion, optimization
AI 理解论文
溯源树
样例
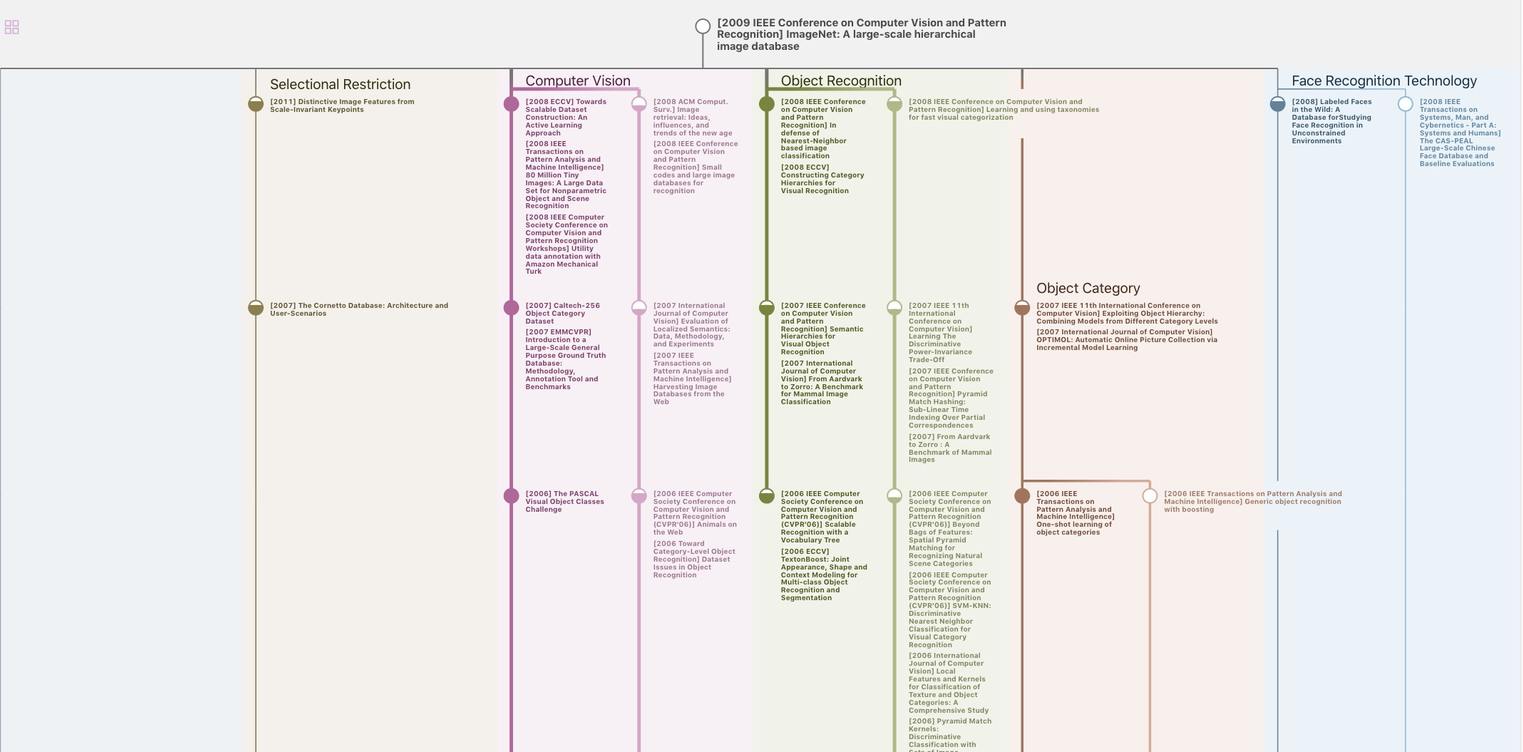
生成溯源树,研究论文发展脉络
Chat Paper
正在生成论文摘要