A Community-Based Topological Distance For Brain-Connectome Classification
JOURNAL OF COMPLEX NETWORKS(2020)
摘要
Measuring differences among complex networks is a well-studied research topic. Particularly, in the context of brain networks, there are several proposals. Nevertheless, most of them address the problem considering unweighted networks. Here, we propose a metric based on modularity and Jaccard index to measure differences among brain-connectivity weighted networks built from diffusion-weighted magnetic resonance data. We use a large dataset to test our metric: a synthetic Ground Truth network (GT) and a set of networks available from a tractography challenge, three sets computed from GT perturbations, and a set of classic random graphs. We compare the performance of our proposal with the most used methods as Euclidean distance between matrices and a kernel-based distance. Our results indicate that the proposed metric outperforms those previously published distances. More importantly, this work provides a methodology that allows differentiating diverse groups of graphs based on their differences in topological structure.
更多查看译文
关键词
network communities, network distances, brain tractography
AI 理解论文
溯源树
样例
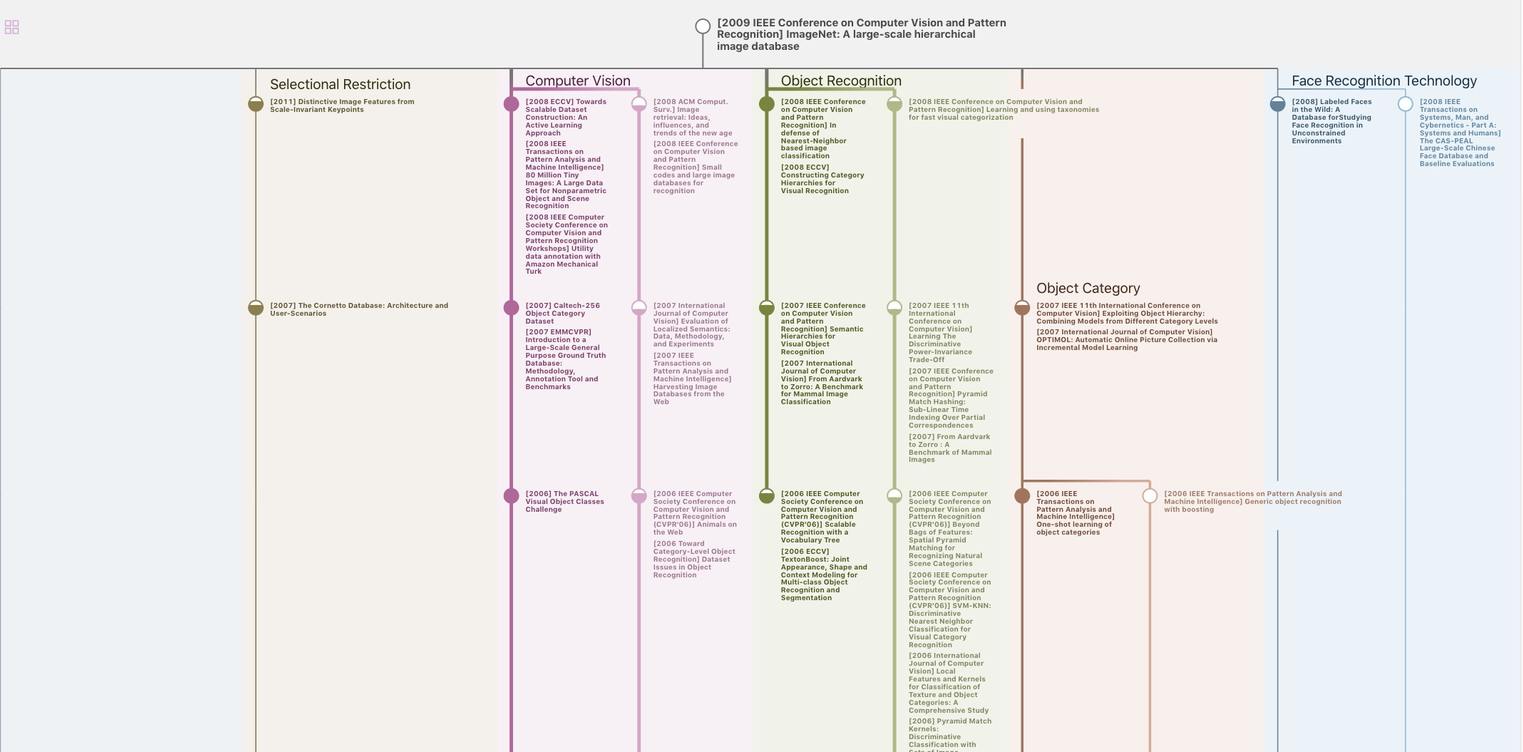
生成溯源树,研究论文发展脉络
Chat Paper
正在生成论文摘要