Predicting shareholder litigation on insider trading from financial text: An interpretable deep learning approach
Information & Management(2020)
摘要
The detrimental effects of insider trading on the financial markets and the economy are well documented. However, resource-constrained regulators face a great challenge in detecting insider trading and enforcing insider trading laws. We develop a text analytics framework that uses machine learning to predict ex-ante potentially opportunistic insider trading (using actual insider trading allegation by shareholders as the proxy) from corporate textual disclosures. Distinct from typical black-box neural network models, which have difficulty tracing a prediction back to key features, our approach combines the predictive power of deep learning with attention mechanisms to provide interpretability to the model. Further, our model utilizes representations from a business proximity network and incorporates the temporal variations of a firm’s financial disclosures. The empirical results offer new insights into insider trading and provide practical implications. Overall, we contribute to the literature by reconciling performance and interpretability in predictive analytics. Our study also informs the practice by proposing a new method for regulators to examine a large amount of text in order to monitor and predict financial misconduct.
更多查看译文
关键词
Insider trading,Predictive analytics,Deep learning,Attention models,Text mining
AI 理解论文
溯源树
样例
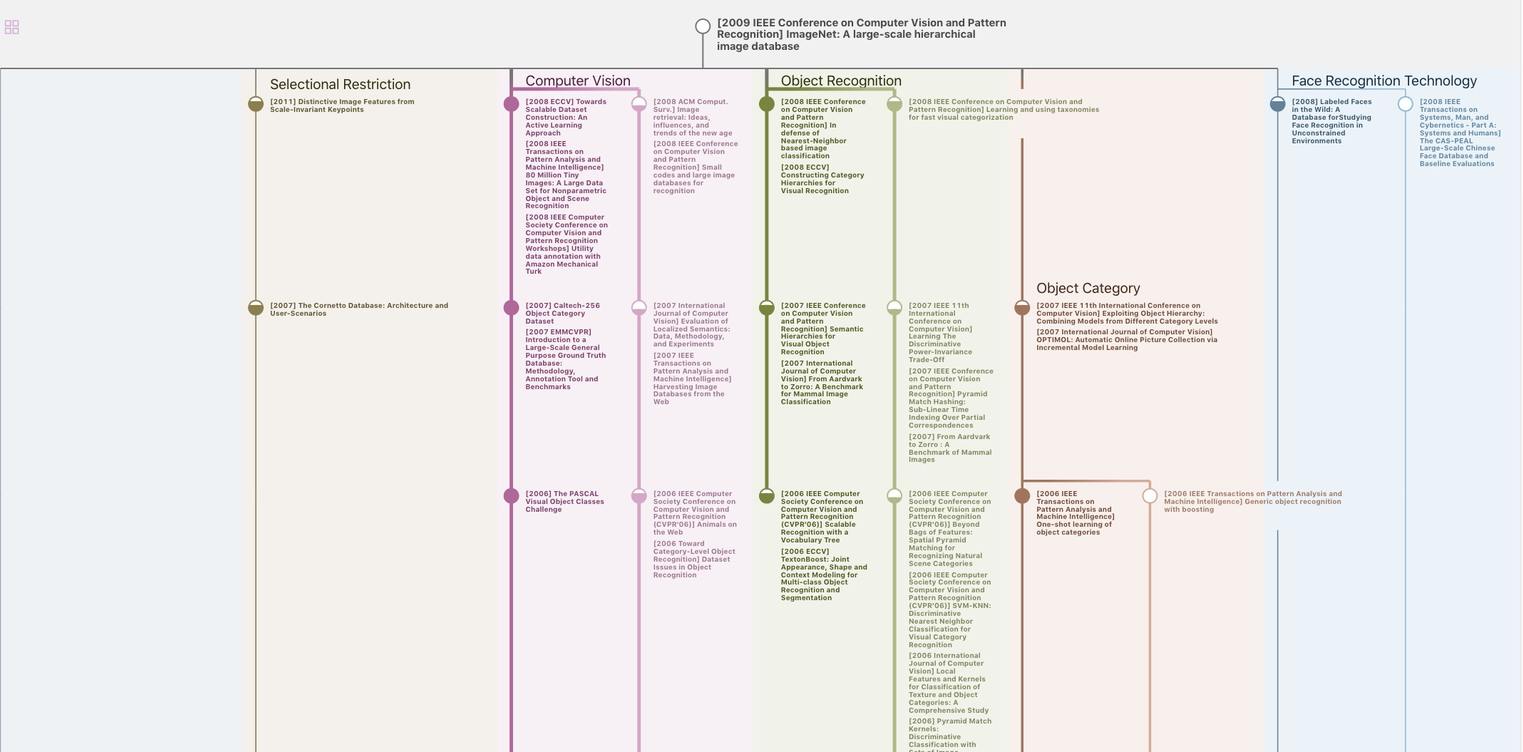
生成溯源树,研究论文发展脉络
Chat Paper
正在生成论文摘要