Multi-view feature transfer for click-through rate prediction
Information Sciences(2021)
摘要
Click-through rate prediction is an important method for online advertising and marketing evaluations. However, for environmental reasons, there is a scarcity and imbalance in the advertising data available. We found that a feature transfer can be applied in a transfer learning method to obtain potential connections from less relevant advertisement data. Considering the complexity and diverse features of advertisement data, a feature transfer cannot allow researchers to discover the relationships among such features within the advertisement data. Therefore, we propose a click-through rate method based on a multi-view feature transfer (MFT). MFT divides the data into common and selected features during the data pretreatment process. It then combines the important feature vectors obtained using a Laplacian matrix with the common features obtained during the pretreatment process to form groups of views. Therefore, combining feature transfer matrix with mutli-view clustering is an innovation of the CTR data prediction process. In our experiments, the MFT model achieved good results. Experiments on a large number of datasets of different sizes and the application of three evaluation indicators show that the MFT method delivers excellent prediction results using the transfer relationships among the characteristics of an advertising dataset, and its performance is better than that of many other advertising click-through rate prediction methods.
更多查看译文
关键词
Click-through rate,Online advertising,Transfer learning,Multi-view,Feature transfer
AI 理解论文
溯源树
样例
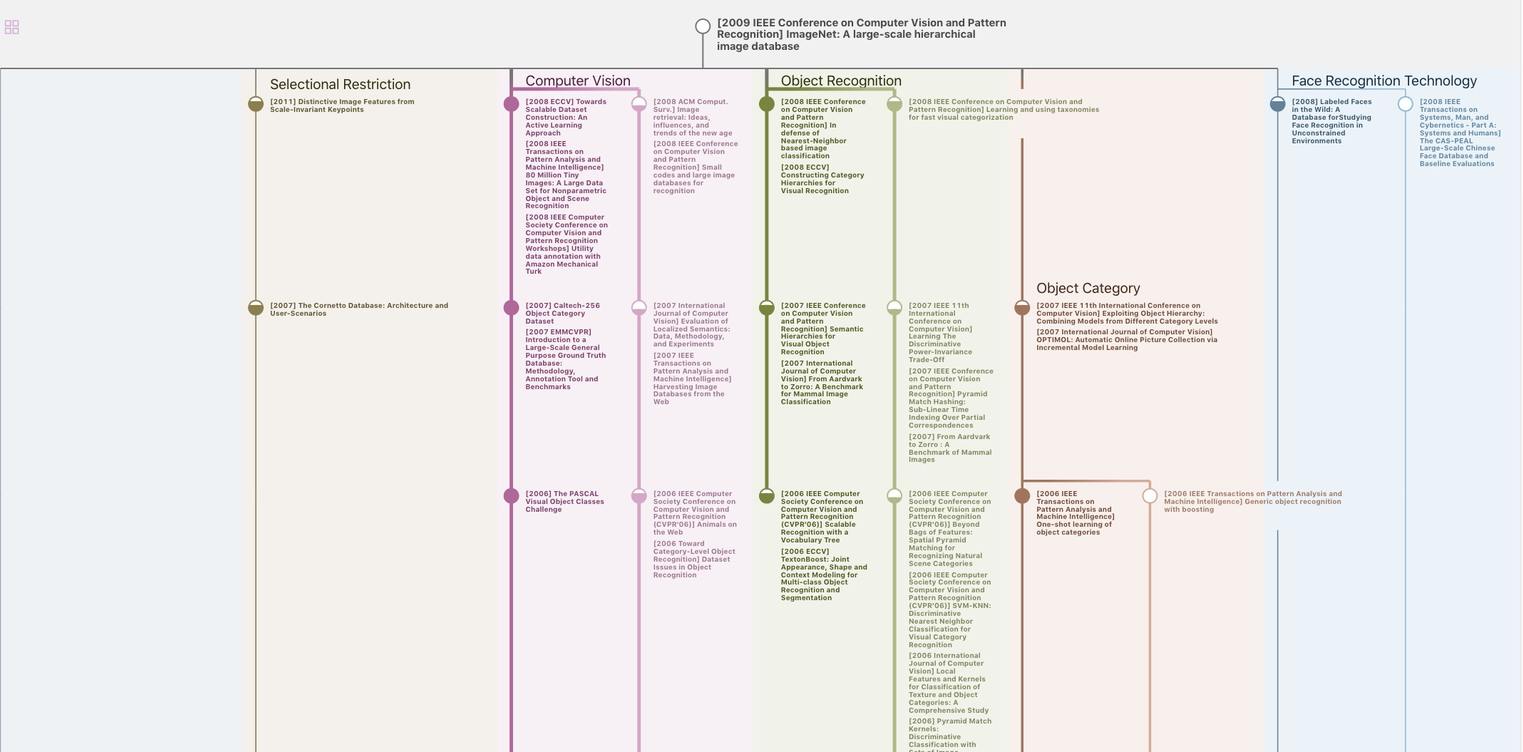
生成溯源树,研究论文发展脉络
Chat Paper
正在生成论文摘要