Using the Crowd to Prevent Harmful AI Behavior.
Proc. ACM Hum. Comput. Interact.(2020)
摘要
To prevent harmful AI behavior, people need to specify constraints that forbid undesirable actions. Unfortunately, this is a complex task, since writing rules that distinguish harmful from non-harmful actions tends to be quite difficult in real-world situations. Therefore, such decisions have historically been made by a small group of powerful AI companies and developers, with limited community input. In this paper, we study how to enable a crowd of non-AI experts to work together to communicate high-quality, reliable constraints to AI systems. We first focus on understanding how humans reason about temporal dynamics in the context of AI behavior, finding through experiments on a novel game-based testbed that participants tend to adopt a long-term notion of harm, even in uncertain situations that do not affect them directly. Building off of this insight, we explore task design for long-term constraint specification, developing new filtering approaches and new methods of promoting user reflection. Next, we develop a novel rule-based interface which allows people to craft rules in an accessible fashion without programming knowledge. We test our approaches on a real-world AI problem in the domain of education, and find that our new filtering mechanisms and interfaces significantly improve constraint quality and human efficiency. We also demonstrate how these systems can be applied to other real-world AI problems (e.g. in social networks).
更多查看译文
AI 理解论文
溯源树
样例
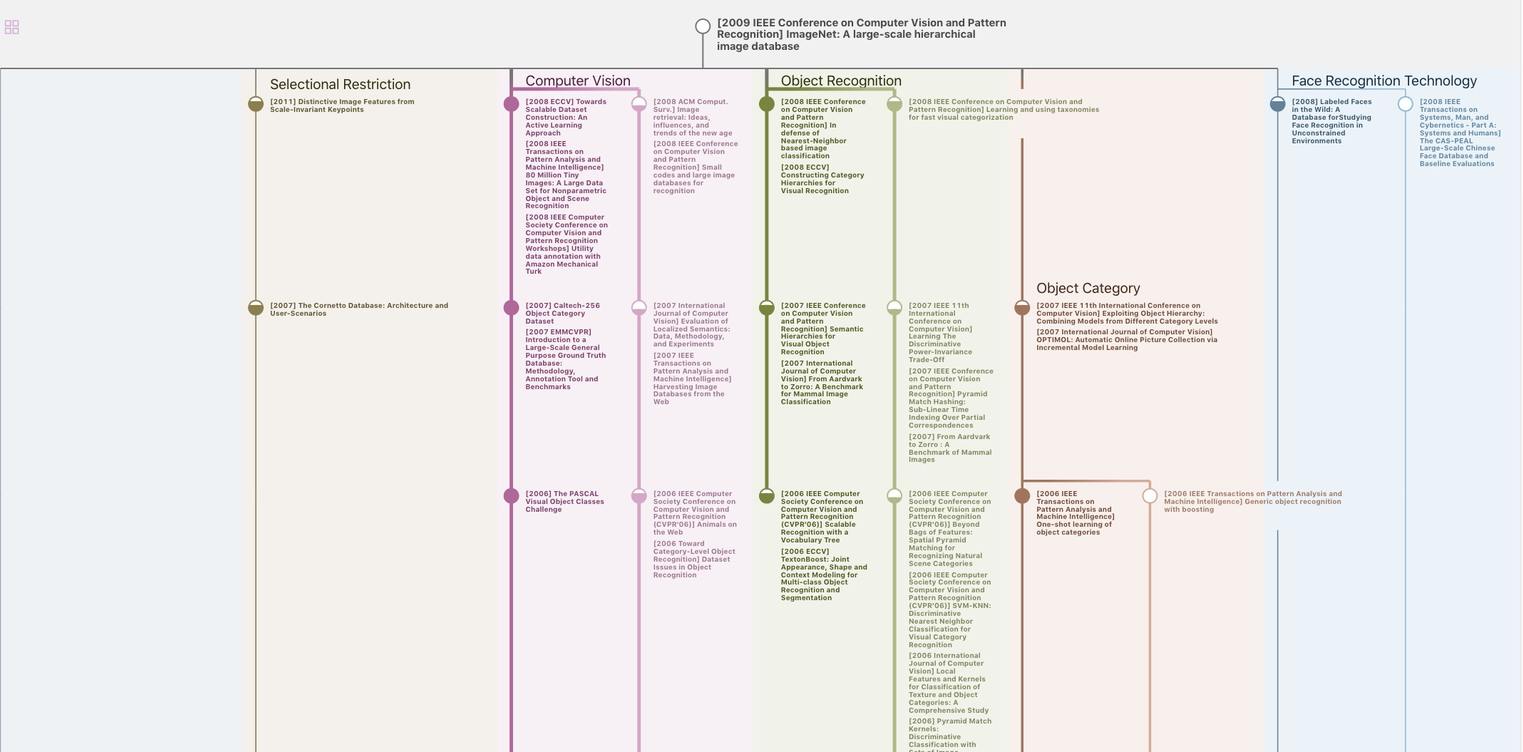
生成溯源树,研究论文发展脉络
Chat Paper
正在生成论文摘要