Mpe Computation In Bayesian Networks Using Mini-Bucket And Probability Trees Approximation
INTERNATIONAL JOURNAL OF UNCERTAINTY FUZZINESS AND KNOWLEDGE-BASED SYSTEMS(2020)
摘要
Given a set of uncertain discrete variables with a joint probability distribution and a set of observations for some of them, the most probable explanation is a set or configuration of values for non-observed variables maximizing the conditional probability of these variables given the observations. This is a hard problem which can be solved by a deletion algorithm with max marginalization, having a complexity similar to the one of computing conditional probabilities. When this approach is unfeasible, an alternative is to carry out an approximate deletion algorithm, which can be used to guide the search of the most probable explanation, by using A* or branch and bound (the approximate+search approach). The most common approximation procedure has been the mini-bucket approach. In this paper it is shown that the use of probability trees as representation of potentials with a pruning of branches with similar values can improve the performance of this procedure. This is corroborated with an experimental study in which computation times are compared using randomly generated and benchmark Bayesian networks from UAI competitions.
更多查看译文
关键词
Bayesian networks, most probable explanation, deletion algorithm, mini-bucket, probability trees
AI 理解论文
溯源树
样例
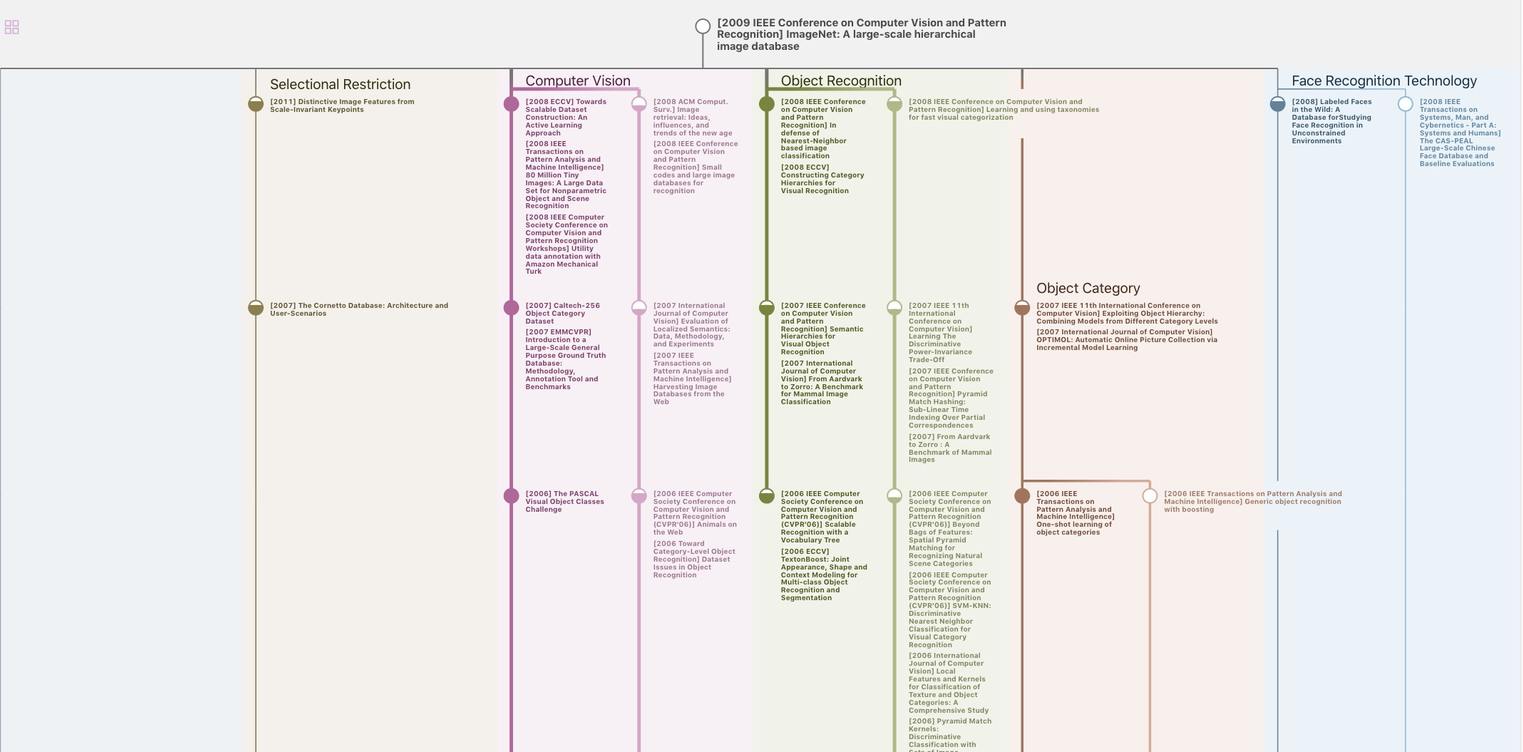
生成溯源树,研究论文发展脉络
Chat Paper
正在生成论文摘要