An incremental density-based clustering framework using fuzzy local clustering
Information Sciences(2021)
摘要
This paper presents a novel incremental density-based clustering framework using the one-pass scheme, named Fuzzy Incremental Density-based Clustering (FIDC). Employing one-pass clustering in which each data point is processed once and discarded, FIDC can process large datasets with less computation time and memory, compared to its density-based clustering counterparts. Fuzzy local clustering is employed in local clusters assignment process to reduce clustering inconsistencies from one-pass clustering. To improve the clustering performance and simplify the parameter choosing process, the modified valley seeking algorithm is used to adaptively determine the outlier thresholds for generating the final clusters. FIDC can operate in both traditional and stream data clustering. The experimental results show that FIDC outperforms state-of-the-art algorithms in both clustering modes.
更多查看译文
关键词
Incremental clustering,Density-based clustering,Fuzzy clustering,Stream data clustering
AI 理解论文
溯源树
样例
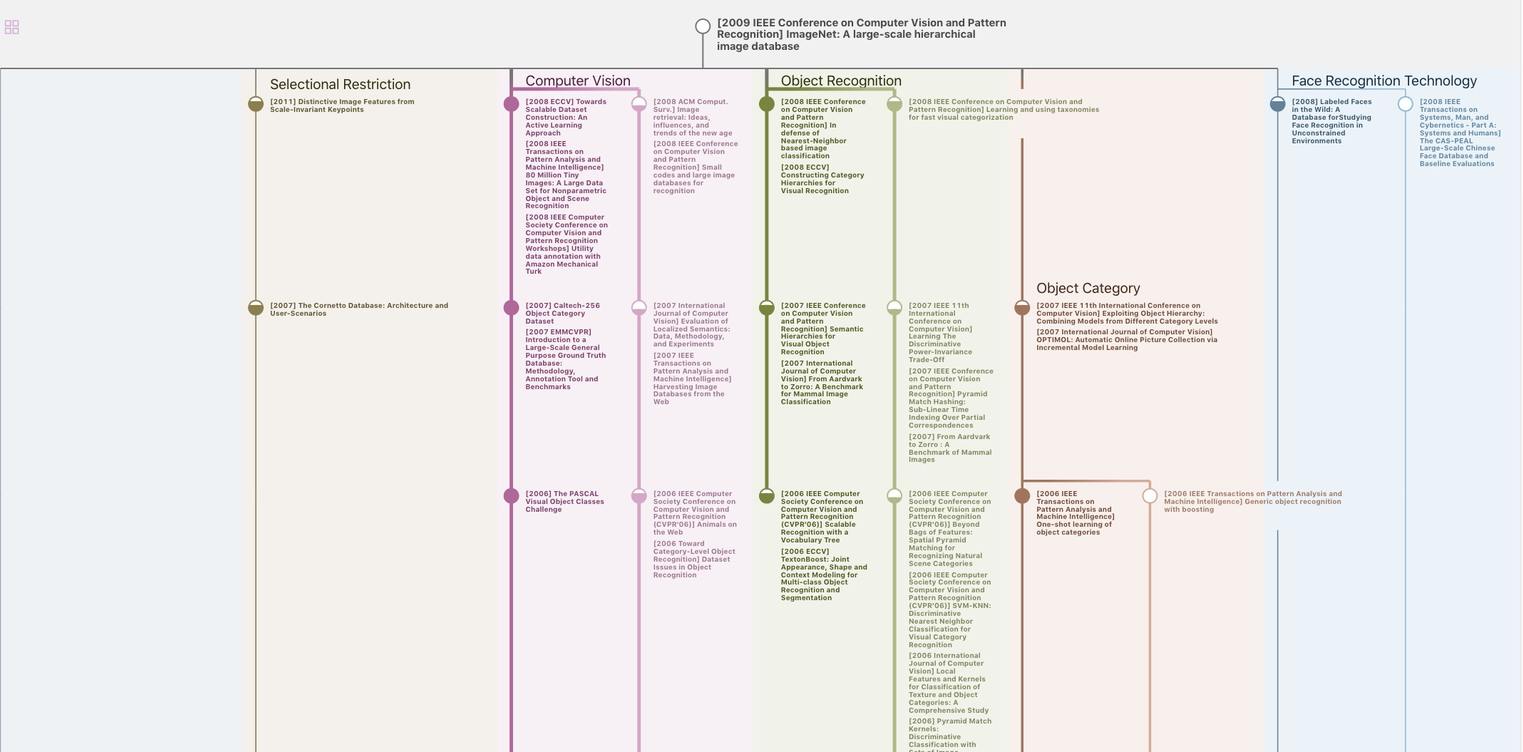
生成溯源树,研究论文发展脉络
Chat Paper
正在生成论文摘要