Qn-Docking: An Innovative Molecular Docking Methodology Based On Q-Networks
APPLIED SOFT COMPUTING(2020)
摘要
Molecular docking is often used in computational chemistry to accelerate drug discovery at early stages. Many molecular simulations are performed to select the right pharmacological candidate. However, traditional docking methods are based on optimization heuristics such as Monte Carlo or genetic that try several hundreds of these candidates giving rise to expensive computations. Thus, an alternative methodology called QN-Docking is proposed for developing docking simulations more efficiently. This new approach is built upon Q-learning using a single-layer feedforward neural network to train a single ligand or drug candidate (the agent) to find its optimal interaction with the host molecule. In addition, the corresponding Reinforcement Learning environment and the reward function based on a force-field scoring function are implemented. The proposed method is evaluated in an exemplary molecular scenario based on the kaempferol and beta-cyclodextrin. Results for the prediction phase show that QN-Docking achieves 8x speedup compared to stochastic methods such as METADOCK 2, a novel high-throughput parallel metaheuristic software for docking. Moreover, these results could be extended to many other ligand-host pairs to ultimately develop a general and faster docking method. (C) 2020 Elsevier B.V. All rights reserved.
更多查看译文
关键词
Q-network, Reinforcement learning, Artificial neural networks, Structure-based drug design, Molecular docking
AI 理解论文
溯源树
样例
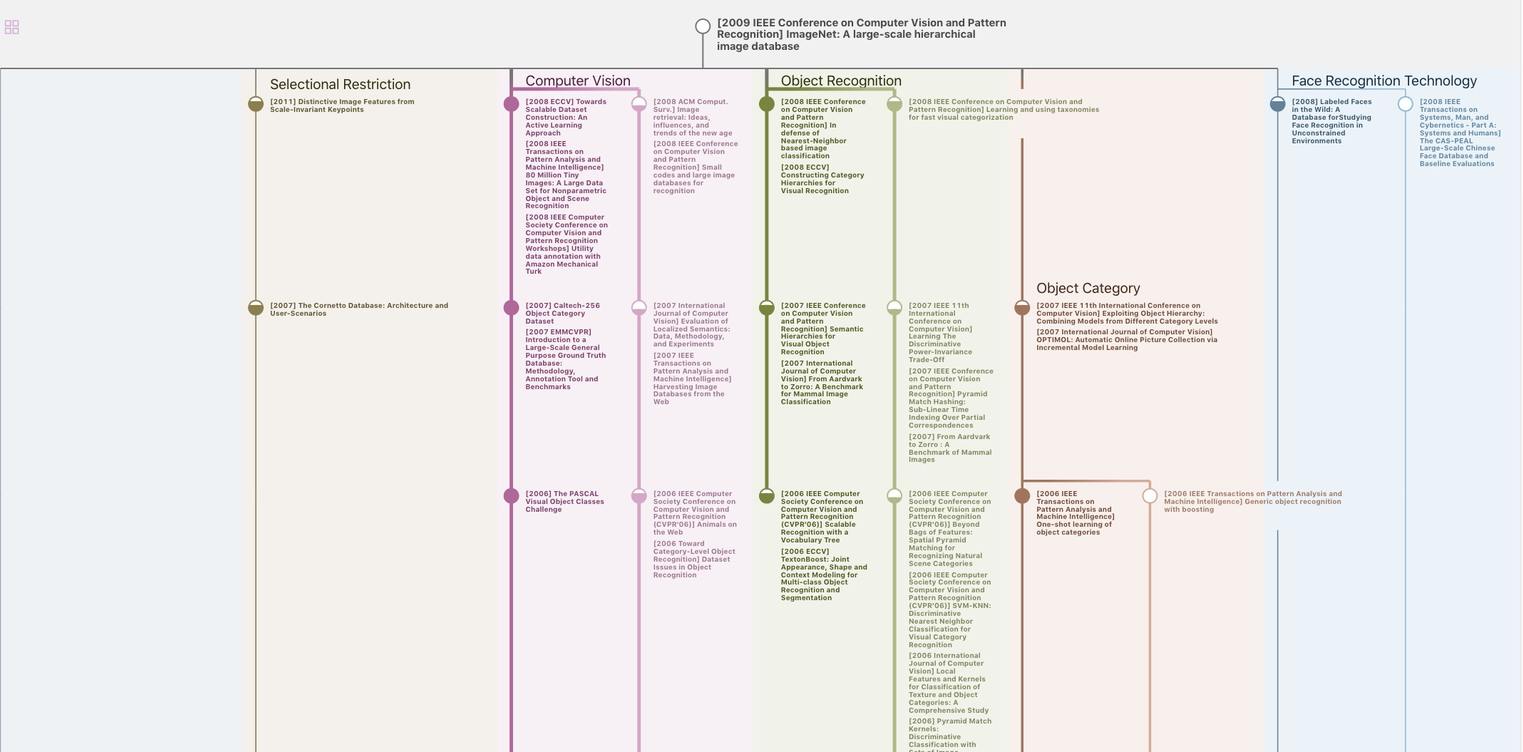
生成溯源树,研究论文发展脉络
Chat Paper
正在生成论文摘要